Various Types of Functional Link Artificial Neural Network Based Nonlinear Equalizers used in CO-OFDM System for Nonlinearities Mitigation
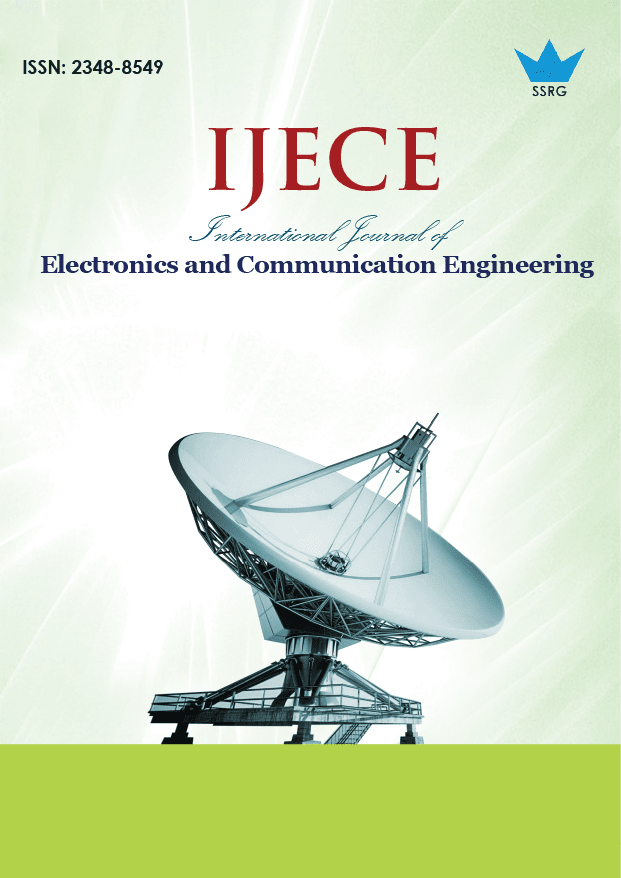
International Journal of Electronics and Communication Engineering |
© 2018 by SSRG - IJECE Journal |
Volume 5 Issue 2 |
Year of Publication : 2018 |
Authors : Gurpreet Kaur and Gurmeet Kaur |
How to Cite?
Gurpreet Kaur and Gurmeet Kaur, "Various Types of Functional Link Artificial Neural Network Based Nonlinear Equalizers used in CO-OFDM System for Nonlinearities Mitigation," SSRG International Journal of Electronics and Communication Engineering, vol. 5, no. 2, pp. 1-6, 2018. Crossref, https://doi.org/10.14445/23488549/IJECE-V5I2P101
Abstract:
Artificial neural network based nonlinear equalizers (ANN-NLEs) have received much attention in the last three years due to its ability of complex mapping between the complex input and output spaces.In the history of ANN-NLE, the focus has always been improving the performance of coherent optical orthogonal frequency division multiplexing (CO-OFDM) system. Recent developments in ANN-NLE have led to a decision that a single neuron based ANN i.e., functional link artificial neural network (FLANN) has been considered as an efficient technique of performance improvement with less computational complexity.There are many types of FLANN available in literature depending on the expansion technique used in network such as PPN (Polynomial Perceptron Network), T-FLANN (Trigonometric Functional link Artificial Neural Network), Le-FLANN (Legendre Functional link Artificial Neural Network) and Ch-FLANN (Chebyshev Functional link Artificial Neural Network).Until now this methodology has only been applied to Ch-FLANN based NLE. It has not yet been established whether other types of FLANN can do the task of nonlinearity mitigation in CO-OFDM system. In this context authors tried to use other types of FLANN-NLE for the mitigation of nonlinearities in CO-OFDM system.
Keywords:
Chebyshev Functional Link ANN (Ch-FLANN); Coherent Optical Orthogonal Frequency Division Multiplexing (CO-OFDM); Legendre Functional Link ANN (Le-FLANN); Multilayer Perceptron (MLP); Polynomial Perceptron Network (PPN); Trigonometric Functional Link ANN (T-FLANN).
References:
[1] W. Shieh, H. Bao, and Y. Tang, “Coherent Optical OFDM: Theory and Design”, Optics Express, vol.16, pp. 842-859, Jan. 2008.
[2] E. Ip, A. Pak Tao Lau, D. J. F. Barros, and J. M. Kahn, “Coherent Detection in Optical Fiber Systems”, Optics Express, vol.16, pp.753-791, Jan.2008.
[3] W. Shieh and I. Djordjevic, OFDM for Optical Communications, Elsevier, Ch.7, 2010.
[4] G. P. Agrawal, Nonlinear Fiber Optics, Academic Press, 2007.
[5] P. Savazzi, L. Favalli, E. Costamagna and A. Mecocci, “A suboptimal approach to channel equalization based on the nearest neighbor rule,” IEEE Journal of Selected Areas in Communications,vol. 16, no. 9, pp. 1640-1648, Dec. 1998.
[6] G. Charalabopoulos, P. Stavroulakis, and A. H. Aghvami, “A frequency domain neural network equalizer for OFDM,” Proceedings IEEE GLOBECOM, vol. 2, pp. 571–575, Dec. 2003.
[7] M. A. Jarajreh, E. Giacoumidis et al., “Artificial Neural Network Nonlinear Equalizer for Coherent Optical OFDM”, IEEE Photonics Technoology Letters, vol. 27, no. 4, Feb. 2015.
[8] Y. H. Pao, Adaptive Pattern Recognition and Neural Network, Reading, MA, Addison Wesley, Ch. 8, pp.197-222, Dec. 1989.
[9] J. C. Patra and R. N. Pal, "A functional link artificial neural network for adaptive channel equalization", EURASIP Signal Processing Journal, vol. 43, no. 2, 1995.
[10] M. A. Jarajreh, J. L. Wei, J. Tang, Z. Ghassemlooy, and W. P. Ng, "Effect of number of sub-carriers, cyclic prefix and analogue to digital converter parameters on coherent optical orthogonal frequency division multiplexing modem's transmission performance," Journal of IET Communications, vol. 4, pp. 213- 233, 2010.
[11] N. T., Complex-valued neural networks: utilizing high-dimensional parameters. Hershey, New York: Information science reference, 2009
[12] G. Kaur, G. Kaur, “Application of Functional Link Artificial Neural Network for Mitigating Nonlinear Effects in Coherent Optical OFDM”, Optical and Quantum Electronics, Springer, May 2017.
[13] M. Riedmiller and H. Braun, “A direct adaptive method for faster backpropagation learning: The RPROP algorithm,” Proceedings IEEE ICNN, San Francisco, CA, USA, pp. 586–591, Apr. 1993.
[14] H. Jeffreys, and B. S. Jeffreys, "Weierstrass's Theorem on Approximation by Polynomials" and "Extension of Weierstrass's Approximation Theory." Methods of Mathematical Physics, 3rd editionCambridge, England: Cambridge University Press, pp. 446-448, 1988.
[15] S. K. Nanda and D. P. Tripathy “Application of Functional Link Artificial Neural Network for Prediction of Machinery Noise in Opencast Mines” Advances in Fuzzy Systems, 2011. Article ID 831261, doi:10.1155/2011/831261
[16] Y. H. Pao and Y. Takefuji, "Functional-link net computing: theory, system architecture, and functionalities," Computer, vol. 25, pp. 76-79, 1992.
[17] Y. H. Pao, "Adaptive pattern recognition and neural networks," 1989.
[18] J. C. Patra and R. N. Pal, "A functional link artificial neural network for adaptive channel equalization," Signal Processing, vol. 43, pp. 181- 195, 1995.