Selfish Herd Optimization with Improved Deep Learning based Intrusion Detection for Secure Wireless Sensor Network
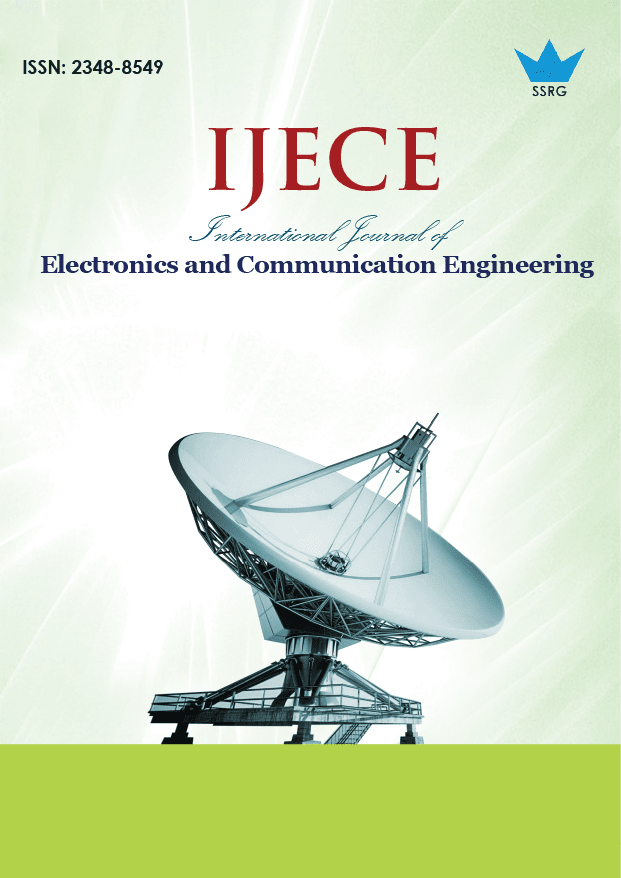
International Journal of Electronics and Communication Engineering |
© 2023 by SSRG - IJECE Journal |
Volume 10 Issue 4 |
Year of Publication : 2023 |
Authors : S. Suma Christal Mary, E. Thenmozhi, K. Murugeswari, N. Senthamilarasi |
How to Cite?
S. Suma Christal Mary, E. Thenmozhi, K. Murugeswari, N. Senthamilarasi, "Selfish Herd Optimization with Improved Deep Learning based Intrusion Detection for Secure Wireless Sensor Network," SSRG International Journal of Electronics and Communication Engineering, vol. 10, no. 4, pp. 1-8, 2023. Crossref, https://doi.org/10.14445/23488549/IJECE-V10I4P101
Abstract:
Wireless sensor networks (WSNs) are becoming more frequently utilized in many applications like environmental monitoring, smart cities, and healthcare. But, security is an important problem in WSNs because of the possible vulnerability to attacks. Intrusion detection systems (IDS) are utilized for detecting and preventing attacks on WSNs. Typical IDS depend on rule-based or signature-based methods that are limited in their capability for detecting before unseen attacks. Deep learning (DL)-based IDS are exposed to promising outcomes in identifying novel attacks. DL-based IDS for WSNs are created utilizing an integration of supervised and unsupervised learning approaches. Therefore, this study designs a Selfish herd optimization with Improved Deep Learning based Intrusion Detection (SHOIDL-ID) technique for secure WSN. The presented SHOIDL-ID technique focuses on the process of identifying and classifying intrusions in the WSN. The presented SHOIDL-ID approach applies data preprocessing to normalize the input data to accomplish this. The SHOIDL-ID technique employs an attention-based bidirectional long short-term memory (ABiLSTM) approach for intrusion recognition and classification. Finally, the SHO approach was utilized for the optimal hyperparameter tuning of the ABiLSTM algorithm. The experimental validation of the SHOIDL-ID approach takes place on the WSN-DS dataset. The outcomes indicate the improved performance of the SHOIDL-ID methodology over other existing approaches in terms of different measures.
Keywords:
Intrusion detection, Wireless sensor networks, Security, Deep learning, Selfish herd optimization.
References:
[1] Abhilash Singh et al., “LT-FS-ID: Log-Transformed Feature Learning and Feature-Scaling-Based Machine Learning Algorithms to Predict the K-Barriers for Intrusion Detection Using Wireless Sensor Network,” Sensors, vol. 22, no. 3, p. 1070, 2022.
[CrossRef] [Google Scholar] [Publisher Link]
[2] Nitin Goyal, Jasminder Kaur Sandhu, and Luxmi Verma, “Machine Learning based Data Agglomeration in Underwater Wireless Sensor Networks,” International Journal of Management, Technology and Engineering, vol. 9, no. 6, pp.240-245, 2019.
[Google Scholar]
[3] V. Subburaj et al., “DDoS Defense Mechanism by Applying Stamps using Cryptography,” International Journal of Computer Applications, vol. 1, no. 6, pp. 48-52, 2010.
[4] I. Gethzi Ahila Poornima, and B. Paramasivan, “Anomaly Detection in Wireless Sensor Network Using Machine Learning Algorithm,” Computer Communications, vol. 151, pp. 331-337, 2020.
[CrossRef] [Google Scholar] [Publisher Link]
[5] Dongxian Yu, Jiatao Kang, and Junlei Dong “Service Attack Improvement in Wireless Sensor Network Based on Machine Learning,” Microprocessors and Microsystems, vol. 80, p. 103637, 2021.
[CrossRef] [Google Scholar] [Publisher Link]
[6] S. Gavaskar, R. Surendiran, and E. Ramaraj, “Three Counter Defense Mechanism for TCP SYN Flooding Attacks,” International Journal of Computer Applications, vol. 6, no. 6, pp. 12-15, 2010.
[CrossRef] [Google Scholar] [Publisher Link]
[7] Periasamy Nancy et al., “Intrusion Detection using Dynamic Feature Selection and Fuzzy Temporal Decision Tree Classification for Wireless Sensor Networks,” IET Communications, vol. 14, no. 5, pp. 888-895, 2020.
[CrossRef] [Google Scholar] [Publisher Link]
[8] Lansheng Han et al., “Intrusion Detection Model of Wireless Sensor Networks Based on Game Theory and an Autoregressive Model,” Information Sciences, vol. 476, pp. 491-504, 2019.
[CrossRef] [Google Scholar] [Publisher Link]
[9] Rui-Hong Dong, Hou-Hua Yan, and Qiu-Yu Zhang, “An Intrusion Detection Model for Wireless Sensor Network Based on Information Gain Ratio and Bagging Algorithm,” International Journal of Network Security, vol. 22, no. 2, pp. 218-230, 2020.
[CrossRef] [Google Scholar]
[10] Gaoming Yang et al., “An Intrusion Detection Algorithm for Sensor Network Based on Normalized Cut Spectral Clustering,” PloS one, vol. 14, no. 10, p. e0221920, 2019.
[CrossRef] [Google Scholar] [Publisher Link]
[11] R. Surendiran, and K. Alagarsamy, “A Crtitical Approach for Intruder Detection in Mobile Devices,” SSRG International Journal of Computer Science and Engineering, vol. 1, no. 4, pp.6-14, 2014.
[CrossRef] [Publisher Link]
[12] Abhishek Raghuvanshi et al., “Intrusion Detection Using Machine Learning for Risk Mitigation in Iot-Enabled Smart Irrigation in Smart Farming,” Journal of Food Quality, pp. 1-8, 2022.
[CrossRef] [Google Scholar] [Publisher Link]
[13] Bandar Almaslukh et al., “Deep Learning and Entity Embedding-Based Intrusion Detection Model for Wireless Sensor Networks,” Computers, Materials & Continua, vol. 69, pp. 1343-1360, 2021.
[CrossRef] [Google Scholar] [Publisher Link]
[14] Prabhdeep Singh, Navdeep Kaur, and Ravneet Kaur, "A Review: Comparative Analysis of Routing Protocols in Wireless Sensor Network," International Journal of P2P Network Trends and Technology, vol. 3, no. 1, pp. 12-17, 2013.
[Google Scholar] [Publisher Link]
[15] Nada M. Alruhaily, and Dina M. Ibrahim, “A Multi-Layer Machine Learning-Based Intrusion Detection System for Wireless Sensor Networks,” International Journal of Advanced Computer Science and Applications, vol. 12, no. 4, pp. 281-288, 2021.
[CrossRef] [Google Scholar] [Publisher Link]
[16] Ruirui Zhang, and Xin Xiao, “Intrusion Detection in Wireless Sensor Networks with an Improved NSA Based on Space Division,” Journal of Sensors, 2019.
[CrossRef] [Google Scholar] [Publisher Link]
[17] Ravneet Kaur, Deepika Sharma, and Navdeep Kaur, "Comparative Analysis of Leach and its Descendant Protocols in Wireless Sensor Network," International Journal of P2P Network Trends and Technology, vol. 3, no. 1, pp. 22-27, 2013.
[Google Scholar] [Publisher Link]
[18] Jeng-Shyang Pan et al., “A Lightweight Intelligent Intrusion Detection Model for Wireless Sensor Networks,” Security and Communication Networks, pp. 1-15, 2021.
[CrossRef] [Google Scholar] [Publisher Link]
[19] Mohit Mittal et al., “Machine Learning Techniques for Energy Efficiency and Anomaly Detection in Hybrid Wireless Sensor Networks,” Energies, vol. 14, no. 11, p. 3125, 2021.
[CrossRef] [Google Scholar] [Publisher Link]
[20] Aashima Singla, and Ratika Sachdeva, "Review on Energy Conservation in Wireless Sensor Network," International Journal of P2P Network Trends and Technology, vol. 3, no. 2, pp. 1-3, 2013.
[Publisher Link]
[21] Xiaopeng Tan et al., “Wireless Sensor Networks Intrusion Detection Based on SMOTE and the Random Forest Algorithm,” Sensors, vol. 19, no. 1, p. 203, 2019.
[CrossRef] [Google Scholar] [Publisher Link]
[22] Samir Fenanir, Fouzi Semchedine, and Abderrahmane Baadache, “A Machine Learning-Based Lightweight Intrusion Detection System for the Internet of Things,” Revue d'Intelligence Artificielle, vol. 33, no. 3, pp. 203-211, 2019.
[CrossRef] [Google Scholar] [Publisher Link]
[23] Mnahi Alqahtani et al., “A Genetic-Based Extreme Gradient Boosting Model for Detecting Intrusions in Wireless Sensor Networks,” Sensors, vol. 19, no. 20, p. 4383, 2019.
[CrossRef] [Google Scholar] [Publisher Link]
[24] S. Gavaskar, E. Ramaraj, and R. Surendiran, “A Compressed Anti IP Spoofing Mechanism Using Cryptography,” International Journal of Computer Science and Network Security, vol. 12, no. 11, pp. 137-140, 2012.
[Google Scholar]
[25] Kanwal Yousaf, and Tabassam Nawaz, “A Deep Learning-Based Approach for Inappropriate Content Detection and Classification of Youtube Videos,” IEEE Access, vol. 10, pp. 16283-16298, 2022.
[CrossRef] [Google Scholar] [Publisher Link]
[26] Narendra Kumar Jena et al., “Fuzzy Adaptive Selfish Herd Optimization Based Optimal Sliding Mode Controller for Frequency Stability Enhancement of a Microgrid,” Engineering Science and Technology, An International Journal, vol. 33, p. 101071, 2022.
[CrossRef] [Google Scholar] [Publisher Link]
[27] Iman Almomani, Bassam Al-Kasasbeh, and Mousa AL-Akhras, “WSN-DS: A Dataset for Intrusion Detection Systems in Wireless Sensor Networks,” Journal of Sensors, pp. 1–16, 2016.
[CrossRef] [Google Scholar] [Publisher Link]
[28] Shuai Jiang, Juan Zhao, and Xiaolong Xu “SLGBM: An Intrusion Detection Mechanism for Wireless Sensor Networks in Smart Environments,” IEEE Access, vol. 8, pp. 169548-169558, 2020.
[CrossRef] [Google Scholar] [Publisher Link]