A Systematic Review on Deep Convolutional Neural Network-based Breast Cancer Classification on Histopathological Images
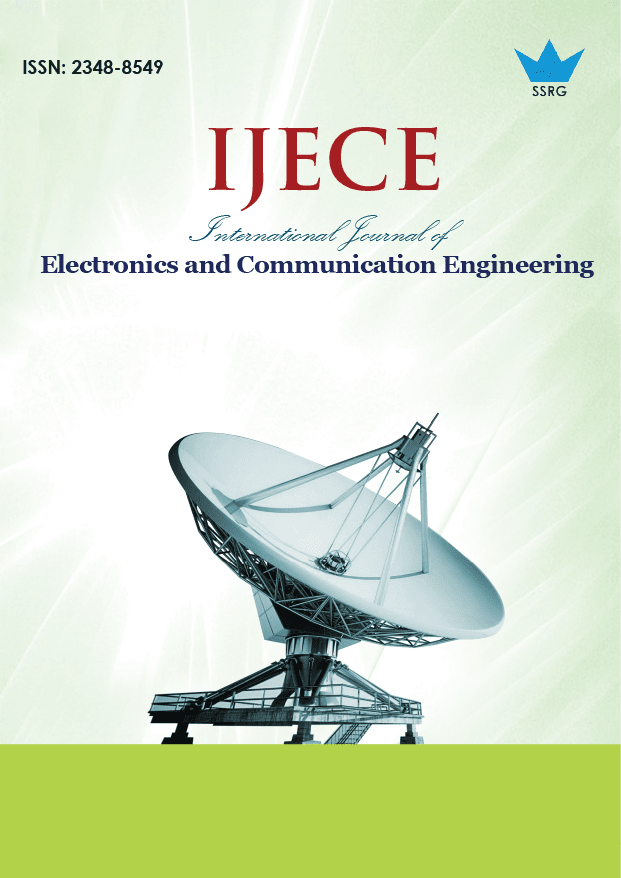
International Journal of Electronics and Communication Engineering |
© 2023 by SSRG - IJECE Journal |
Volume 10 Issue 4 |
Year of Publication : 2023 |
Authors : R. Gurumoorthy, M. Kamarasan |
How to Cite?
R. Gurumoorthy, M. Kamarasan, "A Systematic Review on Deep Convolutional Neural Network-based Breast Cancer Classification on Histopathological Images," SSRG International Journal of Electronics and Communication Engineering, vol. 10, no. 4, pp. 31-40, 2023. Crossref, https://doi.org/10.14445/23488549/IJECE-V10I4P104
Abstract:
Breast cancer (BC) is an increasingly prevalent malignant disease in females globally. Lately, early diagnoses and the best adjuvant therapy have considerably enhanced patient outcomes. However, new challenges have occurred as our growing understanding of tumors has exposed their complex nature, and diagnoses by histopathology have proved to be helpful in guiding BC treatment. We faced an absolute necessity for accurate histopathologic BC diagnoses to make better therapy decisions as patient demand for personalized BC therapy increases. Furthermore, current development in memory capacity and computational power resulted in the applications of medical image processing and Deep Learning (DL) techniques to analyze and process histopathological images (HIs) of BC. Therefore, this study performs a Systematic Review of Deep Convolutional Neural Network based BC Classification on HIs. This survey aims to review the conventional and recently developed DL techniques for BC diagnosis using HIs. Firstly, the role of machine learning (ML) and DL algorithms for HI classification for BC detection is elaborated briefly. Next, the recently developed DL-based HI classification models for BC are reviewed in detail. Moreover, a comparison stud of the reviewed models with result analysis is performed. Furthermore, an elaborate description of the challenging issues with possible future directions is identified at the end of the survey.
Keywords:
Computer-aided diagnosis, Histopathological images, Deep learning, Breast cancer, Machine learning.
References:
[1] Yiping Zhou, Can Zhang, and Shaoshuai Gao, “Breast Cancer Classification from Histopathological Images Using Resolution Adaptive Network,” IEEE Access, vol. 10, pp. 35977-35991, 2022.
[CrossRef] [Google Scholar] [Publisher Link]
[2] Md Zahangir Alom et al., “Breast Cancer Classification from Histopathological Images with Inception Recurrent Residual Convolutional Neural Network,” Journal of Digital Imaging, vol. 32, no. 4, pp. 605-617, 2019.
[CrossRef] [Google Scholar] [Publisher Link]
[3] Dr. Surendiran R et al., "Exploring the Cervical Cancer Prediction by Machine Learning and Deep Learning with Artificial Intelligence Approaches," International Journal of Engineering Trends and Technology, vol. 70, no. 7, pp. 94-107, 2022.
[CrossRef] [Publisher Link]
[4] Mahesh Gour, Sweta Jain, and T. Sunil Kumar, “Residual Learning Based CNN for Breast Cancer Histopathological Image Classification,” International Journal of Imaging Systems and Technology, vol. 30, no. 3, pp. 621-635, 2020.
[CrossRef] [Google Scholar] [Publisher Link]
[5] S. Alkassar et al., “Going Deeper: Magnification-Invariant Approach for Breast Cancer Classification Using Histopathological Images,” IET Computer Vision, vol. 15, no. 2, pp. 151-164, 2021.
[CrossRef] [Google Scholar] [Publisher Link]
[6] Mariam Shadan et al., "Histological Categorization of Stromal Desmoplasia in Breast Cancer and its Diagnostic and Prognostic Utility," SSRG International Journal of Medical Science, vol. 4, no. 6, pp. 8-11, 2017.
[CrossRef] [Google Scholar] [Publisher Link]
[7] Ebrahim Mohammed Senan et al., “Classification of Histopathological Images for Early Detection of Breast Cancer Using Deep Learning,” Journal of Applied Science and Engineering, vol. 24, no. 3, pp. 323-329, 2021.
[CrossRef] [Google Scholar] [Publisher Link]
[8] Kadhim, R.R. and Kamil, M.Y., “Evaluation of Machine Learning Models for Breast Cancer Diagnosis Via Histogram of Oriented Gradients Method and Histopathology Images,” International Journal on Recent and Innovation Trends in Computing and Communication, vol. 10, no. 4, pp. 36-42, 2022.
[CrossRef] [Google Scholar] [Publisher Link]
[9] Nouman Ahmad, Sohail Asghar, and Saira Andleeb Gillani, “Transfer Learning-Assisted Multi-Resolution Breast Cancer Histopathological Images Classification,” The Visual Computer, vol. 38, no. 8, pp. 2751-2770, 2022.
[CrossRef] [Google Scholar] [Publisher Link]
[10] Mohamed Ali Belaid, "Reliability Evaluation of NLDMOS Transistor Based on Advanced Aging Test Including Hot Carrier Phenomenon," SSRG International Journal of Electronics and Communication Engineering, vol. 9, no. 8, pp. 1-7, 2022.
[CrossRef] [Publisher Link]
[11] Md. Nawaj Shari et al., "Design and Simulation of a Circular Microstrip Patch Antenna for Breast Cancer Diagnosis," International Journal of Recent Engineering Science, vol. 7, no. 3, pp. 57-60, 2020.
[CrossRef] [Google Scholar] [Publisher Link]
[12] Syeda Sara Samreen, and Hakeem Aejaz Aslam, "Hyperspectral Image Classification using Deep Learning Techniques: A Review," SSRG International Journal of Electronics and Communication Engineering, vol. 9, no. 6, pp. 1-4, 2022.
[CrossRef] [Google Scholar] [Publisher Link]
[13] Fouqiya Badar, and Ayesha Naaz, "Detection of Fruit Diseases using Image Processing Techniques: A Review," SSRG International Journal of Electronics and Communication Engineering, vol. 9, no. 4, pp. 10-14, 2022.
[CrossRef] [Publisher Link]
[14] Tahir Mahmood et al., “Artificial Intelligence-Based Mitosis Detection in Breast Cancer Histopathology Images Using Faster R-CNN and Deep CNNs,” Journal of Clinical Medicine, vol. 9, no. 3, p. 749, 2020.
[CrossRef] [Google Scholar] [Publisher Link]
[15] Yuxuan Che et al., “Immunohistochemical HER2 Recognition and Analysis of Breast Cancer Based on Deep Learning,” Diagnostics, vol. 13, no. 2, p. 263, 2023.
[CrossRef] [Google Scholar] [Publisher Link]
[16] Zulaiha Parveen A, and Mr.T.Senthil Kumar, "The Deep Learning Methodology for Improved Breast Cancer Diagnosis in MRI," International Journal of Computer and Organization Trends, vol. 11, no. 3, pp. 11-14, 2021.
[CrossRef] [Publisher Link]
[17] R. Sathesh Raaj, “Breast Cancer Detection and Diagnosis Using Hybrid Deep Learning Architecture,” Biomedical Signal Processing and Control, vol. 82, p. 104558, 2023.
[CrossRef] [Google Scholar] [Publisher Link]
[18] Ronald Ck Chan et al., “Artificial Intelligence in Breast Cancer Histopathology,” Histopathology, vol. 82, no. 1, pp. 198-210, 2023.
[CrossRef] [Google Scholar] [Publisher Link]
[19] De Cai et al., “Efficient Mitosis Detection in Breast Cancer Histology Images by RCNN,” 2019 IEEE 16th International Symposium on Biomedical Imaging, IEEE, pp. 919-922, 2019.
[CrossRef] [Google Scholar] [Publisher Link]
[20] Nidhi Mongoriya, and Vinod Patel, "Review The Breast Cancer Detection Technique Using Hybrid Machine Learning," SSRG International Journal of Computer Science and Engineering, vol. 8, no. 6, pp. 5-8, 2021.
[CrossRef] [Publisher Link]
[21] Salar Razavi et al., “MiNuGAN: Dual Segmentation of Mitoses and Nuclei Using Conditional GANs on Multi-center Breast H&E Images,” Journal of pathology informatics, vol. 13, p. 100002, 2022.
[CrossRef] [Google Scholar] [Publisher Link]
[22] S.V. Shwetha, and L. Dharmanna, “An Automatic Recognition, Identification and Classification of Mitotic Cells for the Diagnosis of Breast Cancer Stages,” International Journal of Image Graphics Signal Process, vol. 13, no. 6, pp. 1-11, 2021.
[CrossRef] [Google Scholar] [Publisher Link]
[23] Tasleem Kausar et al., “SmallMitosis: Small Size Mitotic Cells Detection in Breast Histopathology Images,” IEEE Access, vol. 9, pp. 905-922, 2020.
[CrossRef] [Google Scholar] [Publisher Link]
[24] B.Johnson, P.Keerthi Vasan, and V.Thillaivendan, "Performance of Hyperthermia for Breast Cancer," SSRG International Journal of Applied Physics, vol. 3, no. 2, pp. 1-5, 2016.
[CrossRef] [Publisher Link]
[25] Anabia Sohail et al., ‘Mitotic Nuclei Analysis In Breast Cancer Histopathology Images Using Deep Ensemble Classifier,” Medical Image Analysis, vol. 72, p. 102121, 2021.
[CrossRef] [Google Scholar] [Publisher Link]
[26] Sebai, M., Wang, X. and Wang, T., 2020. “Maskmitosis: A Deep Learning Framework for Fully Supervised, Weakly Supervised, and Unsupervised Mitosis Detection in Histopathology Images,” Medical & Biological Engineering & Computing, vol. 58, no. 7, pp. 1603- 1623, 2020.
[CrossRef] [Google Scholar] [Publisher Link]
[27] D. Sujitha Priya, and Dr. B. Sarojini, "Breast Cancer Detection In Mammogram Images Using Region-Growing And Contour-Based Segmentation Techniques," International Journal of Computer & Organization Trends, vol. 3, no. 4, pp. 55-58, 2013.
[Google Scholar] [Publisher Link]
[28] Haijun Lei et al., “Attention-Guided Multi-Branch Convolutional Neural Network for Mitosis Detection from Histopathological Images,” IEEE Journal of Biomedical and Health Informatics, vol. 25, no. 2, pp. 358-370, 2020.
[CrossRef] [Google Scholar] [Publisher Link]
[29] Dev Kumar Das, and Pranab Kumar Dutta., “Efficient Automated Detection of Mitotic Cells from Breast Histological Images Using Deep Convolution Neutral Network with Wavelet Decomposed Patches,” Computers in Biology and Medicine, vol. 104, pp. 29-42, 2019.
[CrossRef] [Google Scholar] [Publisher Link]
[30] Sabeena Beevi K., Madhu S. Nair, and Bindu G.R., “Automatic Mitosis Detection in Breast Histopathology Images Using Convolutional Neural Network Based Deep Transfer Learning,” Biocybernetics and Biomedical Engineering, vol. 39, no. 1, pp. 214- 223, 2019.
[CrossRef] [Google Scholar] [Publisher Link]
[31] Abdalla Saad Abdalla Al-Zawi, "Ki -67 Proliferative Index as a Predictive Tool for Axillary Pathological Complete Response in Node Positive Breast Cancer," SSRG International Journal of Medical Science, vol. 7, no. 11, pp. 1-4, 2020.
[CrossRef] [Google Scholar] [Publisher Link]
[32] I. Onur Sigirci, Abdulkadir Albayrak, and Gokhan Bilgin, “Detection of Mitotic Cells in Breast Cancer Histopathological Images Using Deep Versus Handcrafted Features,” Multimedia Tools and Applications, vol. 81, no. 10, pp. 13179-13202, 2022.
[CrossRef] [Google Scholar] [Publisher Link]
[33] Noorulain Maroof et al., “Mitosis Detection in Breast Cancer Histopathology Images Using Hybrid Feature Space,” Photodiagnosis and Photodynamic Therapy, vol. 31, p.101885, 2020.
[CrossRef] [Google Scholar] [Publisher Link]
[34] Chao Li et al., “Weakly Supervised Mitosis Detection in Breast Histopathology Images Using Concentric Loss,” Medical image analysis, vol. 53, pp. 165-178, 2019.
[CrossRef] [Google Scholar] [Publisher Link]
[35] B. Lakshmanan, S. Priyadharsini, and B. Selvakumar, “Computer Assisted Mitotic Figure Detection in Histopathology Images Based on DenseNetPCA Framework,” Materials Today: Proceedings, 2022.
[CrossRef] [Google Scholar] [Publisher Link]
[36] Anabia Sohail et al., “Deep Object Detection based Mitosis Analysis in Breast Cancer Histopathological Images,” arXiv preprint arXiv:2003.08803.
[CrossRef] [Google Scholar] [Publisher Link]
[37] Hameed Ullah Khan et al., “MSF-Model: Multi-Scale Feature Fusion-based Domain Adaptive Model for Breast Cancer Classification of Histopathology Images,” IEEE Access, vol. 10, pp. 122530-122547, 2022.
[CrossRef] [Google Scholar] [Publisher Link]
[38] Deshmukh Pramod Bhausaheb, and Kanchan Lata Kashyap, “Detection and Classification of Breast Cancer Availing Deep Canid Optimization Based Deep CNN,” Multimedia Tools and Applications, vol. 82, pp. 1-19, 2022.
[CrossRef] [Google Scholar] [Publisher Link]
[39] Daniel O. Tambasco Bruno et al., “LBP Operators on Curvelet Coefficients as an Algorithm to Describe Texture in Breast Cancer Tissues,” Expert Systems with Applications, vol. 55, pp. 329-340, 2016.
[CrossRef] [Google Scholar] [Publisher Link]
[40] Sara Reis et al., “Automated Classifcation of Breast Cancer Stroma Maturity from Histological Images,” IEEE Transactions on Biomedical Engineering, vol. 64, no. 10, pp. 2344– 2352, 2017.
[CrossRef] [Google Scholar] [Publisher Link]
[41] Yungang Zhang, Bailing Zhang, and Wenjin Lu et al., “Breast Cancer Classification from Histological Images with Multiple Features and Random Subspace Classifier Ensemble,” AIP Conference Proceedings, vol. 1371, no. 1, pp. 19-28, 2011.
[CrossRef] [Google Scholar] [Publisher Link]
[42] Tao Wan et al., “Automated Grading of Breast Cancer Histopathology Using Cascaded Ensemble with Combination of Multi-Level Image Features,” Neurocomputing, vol. 229, pp. 34-44, 2017.
[CrossRef] [Google Scholar] [Publisher Link]
[43] Teresa Araújo et al., “Classification of Breast Cancer Histology Images Using Convolutional Neural Networks,” PloS one, vol. 12, no. 6, p. e0177544, 2017.
[CrossRef] [Google Scholar] [Publisher Link]
[44] Kaushiki Roy et al., “Patch-Based System for Classification of Breast Histology Images Using Deep Learning,” Computerized Medical Imaging and Graphics, vol. 71, pp. 90-103, 2019.
[CrossRef] [Google Scholar] [Publisher Link]
[45] Qanita Bani Baker et al., “Automated Detection of Benign and Malignant in Breast Histopathology Images,” 2018 IEEE/ACS 15th International Conference on Computer Systems and Applications, IEEE, pp. 1-5, 2018.
[CrossRef] [Google Scholar] [Publisher Link]
[46] Tasleem Kausar, MingJiang Wang, and M. S. S. Malik, “Cancer Detection in Breast Histopathology with Convolution Neural Network Based Approach,” 2019 IEEE/ACS 16th International Conference on Computer Systems and Applications, IEEE, pp. 1-5, 2019.
[CrossRef] [Google Scholar] [Publisher Link]
[47] Sabeena Beevi K., Madhu S. Nair, and Bindu G.R., “Automatic Mitosis Detection in Breast Histopathology Images Using Convolutional Neural Network Based Deep Transfer Learning,” Biocybernetics and Biomedical Engineering, vol. 39, no. 1, pp. 214- 223, 2019.
[CrossRef] [Google Scholar] [Publisher Link]
[48] Duc My Vo, Ngoc-Quang Nguyen, and Sang-Woong Lee, “Classification of Breast Cancer Histology Images Using Incremental Boosting Convolution Networks,” Information Sciences, vol. 482, pp. 123-138, 2019.
[CrossRef] [Google Scholar] [Publisher Link]
[49] Jiajia Cao et al., “An Automatic Breast Cancer Grading Method in Histopathological Images Based on Pixel-, Object-, and Semantic Level Features,” 2016 IEEE 13th International Symposium on Biomedical Imaging, IEEE, pp. 1151-1154, 2016.
[CrossRef] [Google Scholar] [Publisher Link]
[50] SanaUllah Khan et al., “A Novel Deep Learning Based Framework For The Detection And Classification Of Breast Cancer Using Transfer Learning,” Pattern Recognition Letters, vol. 125, pp. 1-6, 2019.
[CrossRef] [Google Scholar] [Publisher Link]
[51] Karan Gupta, and Nidhi Chawla, “Analysis of Histopathological Images for Prediction of Breast Cancer Using Traditional Classifiers With Pre-Trained CNN,” Procedia Computer Science, vol. 167, pp. 878-889, 2020.
[CrossRef] [Google Scholar] [Publisher Link]
[52] Kalpana George et al., “Breast Cancer Detection from Biopsy Images Using Nucleus Guided Transfer Learning and Belief Based Fusion,” Computers in Biology and Medicine, vol. 124, p. 103954, 2020.
[CrossRef] [Google Scholar] [Publisher Link]
[53] Yongjun Wang et al., “Breast Cancer Image Classification via Multi-Network Features and Dual-Network Orthogonal Low-Rank Learning,” IEEE Access, vol. 8, pp. 27779-27792, 2020.
[CrossRef] [Google Scholar] [Publisher Link]
[54] Yusuf Celik et al., “Automated Invasive Ductal Carcinoma Detection Based Using Deep Transfer Learning with Whole-Slide Images,” Pattern Recognition Letters, vol. 133, pp. 232-239, 2020.
[CrossRef] [Google Scholar] [Publisher Link]
[55] Irum Hirra et al., “Breast Cancer Classification from Histopathological Images Using Patch-Based Deep Learning Modeling,” IEEE Access, vol. 9, pp. 24273-24287.
[CrossRef] [Google Scholar] [Publisher Link]
[56] Said Boumaraf et al., “A New Transfer Learning Based Approach to Magnification Dependent and Independent Classification of Breast Cancer in Histopathological Images,” Biomedical Signal Processing and Control, vol. 63, p. 102192, 2021.
[CrossRef] [Google Scholar] [Publisher Link]
[57] Soham Chattopadhyay et al., “DRDA-Net: Dense Residual Dual-Shuffle Attention Network for Breast Cancer Classification Using Histopathological Images,” Computers in Biology and Medicine, vol. 145, p. 105437, 2022.
[CrossRef] [Google Scholar] [Publisher Link]
[58] Amin Alqudah, and Ali Mohammad Alqudah, “Sliding Window Based Support Vector Machine System for Classification of Breast Cancer Using Histopathological Microscopic Images,” IETE Journal of Research, vol. 68, no. 1, pp. 59-67, 2022.
[CrossRef] [Google Scholar] [Publisher Link]
[59] Agaba Ameh Joseph et al., “Improved Multi-Classification of Breast Cancer Histopathological Images Using Handcrafted Features and Deep Neural Network (Dense Layer),” Intelligent Systems with Applications, vol. 14, p. 200066, 2022.
[CrossRef] [Google Scholar] [Publisher Link]
[60] Mamoona Humayun et al., “Framework for Detecting Breast Cancer Risk Presence Using Deep Learning,” Electronics, vol. 12, no. 2, p. 403, 2023.
[CrossRef] [Google Scholar] [Publisher Link]