Supervised Learning-Based Noise Detection to Improve the Performance of Filter-Based ECG Signal Denoising
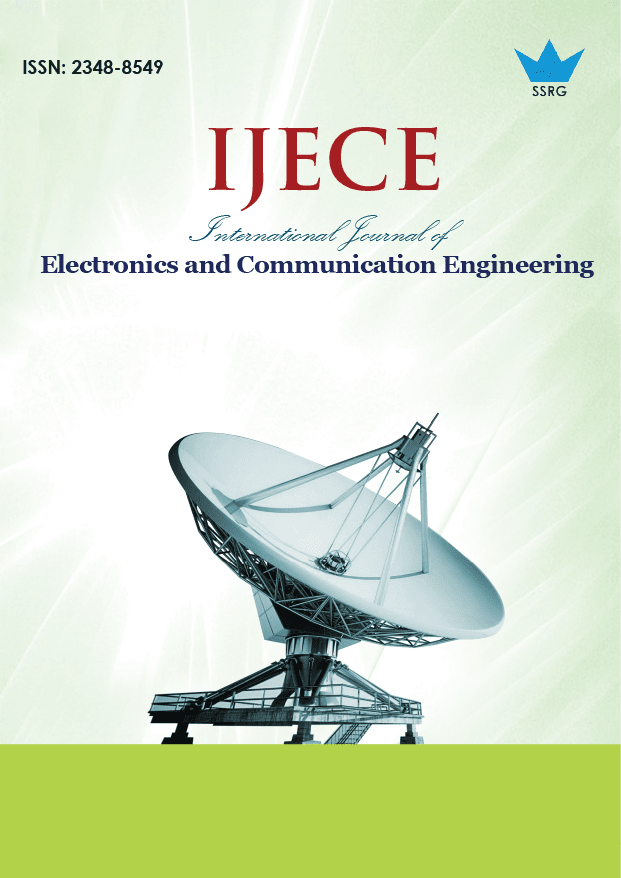
International Journal of Electronics and Communication Engineering |
© 2023 by SSRG - IJECE Journal |
Volume 10 Issue 6 |
Year of Publication : 2023 |
Authors : Veerabomma Supraja, Pasumarthy Nageswara Rao, Mahendra Nanjappa Giri Prasad |
How to Cite?
Veerabomma Supraja, Pasumarthy Nageswara Rao, Mahendra Nanjappa Giri Prasad, "Supervised Learning-Based Noise Detection to Improve the Performance of Filter-Based ECG Signal Denoising," SSRG International Journal of Electronics and Communication Engineering, vol. 10, no. 6, pp. 35-51, 2023. Crossref, https://doi.org/10.14445/23488549/IJECE-V10I6P105
Abstract:
A significant purpose in detecting pervasive computing approaches is to improve the specificity and sensitivity of arrhythmia detection using electrocardiograms. Because ECG signals frequently propagate over distributed computer settings such as the medical Internet of things, noise is prevalent (medical IoT). In these distributed and widespread computing-aided methodologies, noisy electrocardiograms are common to false alarms. The fundamental goal of the machine learning-based arrhythmia detection algorithms discussed in this publication is to detect noise scope in electrocardiograms. The suggested approach determines whether or not the provided electrocardiograms are influenced by noise. In this regard, the approach takes advantage of the electrocardiogram's temporal and spectral characteristics. The performance of the suggested technique was evaluated using multifold cross-validation. In addition, a comparative study was performed comparing the average of peak Signal to Noise Ratio, respective standard deviation and filter sequence length obtained from filtering noise by FIR and IIR filters from raw ECG signals and the SLND-selected noisy ECG signals.
Keywords:
Electro Cardiogram (ECG), Baseline Wandering (BA), Powerline Interference (PLI), Weiner Filter (WF), Fourier-transform (FT), FIR, IIR.
References:
[1] A. Kumar, “ECG-Simplified, LifeHugger, 2010.
[Google Scholar]
[2] Robert O. Bonow et al., Braunwald’s Heart Disease: A Textbook of Cardiovascular Medicine, Elsevier Saunders, vol. 2, 9th Edition, 2021.
[Google Scholar] [Publisher Link]
[3] Jimmy Ming-Tai Wu et al., “A Deep Neural Network Electrocardiogram Analysis Framework for Left Ventricular Hypertrophy Prediction,” Journal of Ambient Intelligence and Humanized Computing, pp. 1-17, 2020.
[CrossRef] [Google Scholar] [Publisher Link]
[4] Carlos Van Mieghem, Marc Sabbe, and Daniel Knockaert, "The Clinical Value of the ECG in Non-Cardiac Conditions," Chest, vol. 125, no. 4, pp. 1561-1576, 2004.
[CrossRef] [Google Scholar] [Publisher Link]
[5] Care I. E, "Part 8: Stabilization of the Patient with Acute Coronary Syndromes," Circulation, vol. 112, no. 24_supplement, pp. IV-90- IV-110, 2005.
[CrossRef] [Google Scholar] [Publisher Link]
[6] Hongqiang Li et al., "Arrhythmia Classification Based on Multi-Domain Feature Extraction for an ECG Recognition System," Sensors, vol. 16, no. 10, 2016.
[CrossRef] [Google Scholar] [Publisher Link]
[7] A. Pushpa, and I. Santi Prabha, "Implementation of Constrained Stability Least Mean Square Algorithm for Suppression of Noise in Cardiac Signals," International Journal of Emerging Technology and Advanced Engineering, vol. 3, no. 8, pp. 187-190, 2013.
[Google Scholar] [Publisher Link]
[8] Ubedur Rehman, and V. S. Jadhav, "Noise Removal from ECG using Modified CSLMS Algorithm," International Journal of Electronics, Communication and Instrumentation Engineering Research and Development, vol. 4, no. 3, pp. 53-60, 2014.
[Google Scholar] [Publisher Link]
[9] Madapuri Rudra Kumar, and Vinit Kumar Gunjan, “Review of Machine Learning Models for Credit Scoring Analysis,” Ingeniería Solidaria, vol. 16, no. 1, pp. 1-16, 2020.
[CrossRef] [Google Scholar] [Publisher Link]
[10] Anitha Boge, V. Vijaya, and K. Kishan Rao, "Clearing Artifacts using a Constrained Stability Least Mean Square Algorithm from Cardiac Signals," International Journal of Scientific and Engineering Research, vol. 3, no. 11, pp. 421-426, 2012.
[Google Scholar] [Publisher Link]
[11] Joo-Chang Kim, and Kyungyong Chung, "Neural-Network Based Adaptive Context Prediction Model for Ambient Intelligence," Journal of Ambient Intelligence and Humanized Computing, vol. 11, no. 4, pp. 1451-1458, 2020.
[CrossRef] [Google Scholar] [Publisher Link]
[12] Nusrat J. Shoumy et al., "Multimodal Big Data Affective Analytics: A Comprehensive Survey using Text, Audio, Visual and Physiological Signals," Journal of Network and Computer Applications, vol. 149, pp. 1-34, 2020.
[CrossRef] [Google Scholar] [Publisher Link]
[13] João Rodrigues, David Belo, and Hugo Gamboa, "Noise Detection on ECG Based on Agglomerative Clustering of Morphological Features," Computers in Biology and Medicine, vol. 87, pp. 322-334, 2017.
[CrossRef] [Google Scholar] [Publisher Link]
[14] Haemwaan Sivaraks, and Chotirat Ann Ratanamahatana, "Robust and Accurate Anomaly Detection in ECG Artifacts using Time Series Motif Discovery," Computational and Mathematical Methods in Medicine, vol. 2015, pp. 1-20, 2015.
[CrossRef] [Google Scholar] [Publisher Link]
[15] Udit Satija, Barathram Ramkumar, and M. Sabarimalai Manikandan, "Automated ECG Noise Detection and Classification System for Unsupervised Healthcare Monitoring," IEEE Journal of Biomedical and Health Informatics, vol. 22, no. 3, pp. 722-732, 2018.
[CrossRef] [Google Scholar] [Publisher Link]
[16] S. Cuomo et al., "A Revised Scheme for Real Time ECG Signal Denoising Based on Recursive Filtering, Biomedical Signal Processing and Control, vol. 27, pp. 134-144, 2016.
[CrossRef] [Google Scholar] [Publisher Link]
[17] Santosh Kumar Yadav, Rohit Sinha, and Prabin Kumar Bora, "Electrocardiogram Signal Denoising using Non-Local Wavelet Transform Domain Filtering," IET Signal Processing, vol. 9, no. 1, pp. 88-96, 2015.
[CrossRef] [Google Scholar] [Publisher Link]
[18] M. Izzetoglu et al., "Motion Artifact Cancellation in NIR Spectroscopy using Wiener Filtering," IEEE Transactions on Biomedical Engineering, vol. 52, no. 5, pp. 934-938, 2005.
[CrossRef] [Google Scholar] [Publisher Link]
[19] Guohua Lu et al., "Removing ECG Noise from Surface EMG Signals using Adaptive Filtering," Neuroscience Letters, vol. 462, no. 1, pp. 14-19, 2009.
[CrossRef] [Google Scholar] [Publisher Link]
[20] C. Marque et al., "Adaptive Filtering for ECG Rejection from Surface EMG Recordings," Journal of Electromyography and Kinesiology, vol. 15, no. 3, pp. 310-315, 2005.
[CrossRef] [Google Scholar] [Publisher Link]
[21] Sarang L. Joshi, Rambabu A Vatti, and Rupali V. Tornekar, "A Survey on ECG Signal Denoising Techniques," 2013 International Conference on Communication Systems and Network Technologies, pp. 60-64, 2013.
[CrossRef] [Google Scholar] [Publisher Link]
[22] Brij N. Singh, and Arvind K. Tiwari, "Optimal Selection of Wavelet Basis Function Applied to ECG Signal Denoising," Digital Signal Processing, vol. 16, no. 3, pp. 275-287, 2006.
[CrossRef] [Google Scholar] [Publisher Link]
[23] Md. Abdul Awal et al., "An Adaptive Level Dependent Wavelet Thresholding for ECG Denoising," Biocybernetics and Biomedical Engineering, vol. 34, no. 4, pp. 238-249, 2014.
[CrossRef] [Google Scholar] [Publisher Link]
[24] S. Sandhya Kumari, and K. Sandhya Rani, "Selection of MSER Region Based Ultrasound Doppler Scan Image Big Data Classification using a Faster RCNN Network," International Journal of Computer Engineering in Research Trends, vol. 9, no. 10, pp. 184-192, 2022.
[Publisher Link]
[25] Pratik Singh, Gayadhar Pradhan, and Shahnawazuddin S, “Denoising of ECG Signal by Non-Local Estimation of Approximation Coefficients in DWT,” Biocybernetics and Biomedical Engineering, vol. 37, no. 3, pp. 599-610, 2017.
[CrossRef] [Google Scholar] [Publisher Link]
[26] Manas Rakshit, and Susmita Das, "An Efficient ECG Denoising Methodology using Empirical Mode Decomposition and Adaptive Switching Mean Filter," Biomedical Signal Processing and Control, vol. 40, pp. 140-148, 2018.
[CrossRef] [Google Scholar] [Publisher Link]
[27] Salim Lahmiri, “Comparative Study of ECG Signal Denoising by Wavelet Thresholding in Empirical and Variational Mode Decomposition Domains,” Healthcare Technology Letters, vol. 1, no. 3, pp. 104-109, 2014.
[CrossRef] [Google Scholar] [Publisher Link]
[28] Kandala N. V. P. S. Rajesh, and Ravindra Dhuli, “Classification of Imbalanced ECG Beats using Re-Sampling Techniques and AdaBoost Ensemble Classifier,” Biomedical Signal Processing and Control, vol. 41, pp. 242-254, 2018.
[CrossRef] [Google Scholar] [Publisher Link]
[29] Paweł Pławiak, and U. Rajendra Acharya, “Novel Deep Genetic Ensemble of Classifiers for Arrhythmia Detection using ECG Signals,” Neural Computing and Applications, vol. 32, no. 15, pp. 11137-11161, 2020.
[CrossRef] [Google Scholar] [Publisher Link]
[30] P. E. Tikkanen, "Nonlinear Wavelet and Wavelet Packet Denoising of Electrocardiogram Signal," Biological Cybernetics, vol. 80, no. 4, pp. 259-267, 1999.
[CrossRef] [Google Scholar] [Publisher Link]
[31] Mangi Kang, Jaelim Ahn, and Kichun Lee, "Opinion Mining using Ensemble Text Hidden Markov Models for Text Classification," Expert Systems with Applications, vol. 94, pp. 218-227, 2018.
[CrossRef] [Google Scholar] [Publisher Link]
[32] N. Sathya, "An Area Efficient Denoising Architecture using Adaptive Rank Order Filter," International Journal of Recent Engineering Science (IJRES), vol. 1, no. 1, pp. 11-15, 2014.
[Publisher Link]
[33] S. Iravanian, and L. Tung, "A Novel Algorithm for Cardiac Biosignal Filtering Based on Filtered Residue Method," IEEE Transactions on Biomedical Engineering, vol. 49, no. 11, pp. 1310-1317, 2002.
[CrossRef] [Google Scholar] [Publisher Link]
[34] J. M. Leski, "Robust Weighted Averaging of Biomedical Signals," IEEE Transactions on Biomedical Engineering, vol. 49, no. 8, pp. 796-804, 2002.
[CrossRef] [Google Scholar] [Publisher Link]
[35] Norden E. Huang et al., "The Empirical Mode Decomposition and the Hilbert Spectrum for Nonlinear and Non-Stationary Time Series Analysis," Proceedings: Mathematical, Physical and Engineering Sciences, vol. 454, no. 1971, pp. 903-995, 1998.
[CrossRef] [Google Scholar] [Publisher Link]
[36] Md. Ashfanoor Kabir, and Celia Shahnaz, "Denoising of ECG Signals Based on Noise Reduction Algorithms in EMD and Wavelet Domains," Biomedical Signal Processing and Control, vol. 7, no. 5, pp. 481-489, 2012.
[CrossRef] [Google Scholar] [Publisher Link]
[37] V. Kishen Ajay Kumar et al., "Dynamic Wavelength Scheduling by Multiobjective in OBS Networks," Journal of Mathematics, vol. 2022, 2022.
[CrossRef] [Google Scholar] [Publisher Link]
[38] Allan Kardec Barros, Ali Mansour, and Noboru Ohnishi, "Removing Artifacts from Electrocardiographic Signals using Independent Components Analysis," Neurocomputing, vol. 22, no. 1-3, pp. 173-186, 1998.
[CrossRef] [Google Scholar] [Publisher Link]
[39] S. Sandhya Kumari, and K. Sandhya Rani, "Empirical Mode Decomposition and Dual Sigmoid Activation Function-Based Faster RCNN for Big Data Doppler Scan Image Classification," International Journal of Computer Engineering in Research Trends, vol. 8, no. 9, pp. 151-165, 2021.
[Google Scholar] [Publisher Link]
[40] Muhammad Zia Ur Rahman, Rafi Ahamed Shaik, and D. V. Rama Koti Reddy, "Efficient and Simplified Adaptive Noise Cancelers for ECG Sensor Based Remote Health Monitoring," IEEE Sensors Journal, vol. 12, no. 3, pp. 566-573, 2012.
[CrossRef] [Google Scholar] [Publisher Link]
[41] M. M. Venkata Chalapathi et al., "Ensemble Learning by High-Dimensional Acoustic Features for Emotion Recognition from Speech Audio Signal," Security and Communication Networks, vol. 2022, 2022.
[CrossRef] [Google Scholar] [Publisher Link]
[42] Dahee Chung et al., "Construction of an Electrocardiogram Database Including 12 Lead Waveforms," Healthcare Informatics Research, vol. 24, no. 3, pp. 242-246, 2018.
[CrossRef] [Google Scholar] [Publisher Link]
[43] Angelina Prima Kurniati et al., "Process Mining in Oncology using the MIMIC-III Dataset," Journal of Physics: Conference Series, vol. 971, no. 1, 2018.
[CrossRef] [Google Scholar] [Publisher Link]
[44] Dukyong Yoon et al., "System for Collecting Biosignal Data from Multiple Patient Monitoring Systems," Healthcare Informatics Research, vol. 23, no. 4, pp. 333-337, 2017.
[CrossRef] [Google Scholar] [Publisher Link]
[45] Shailesh Kumar, Damodar Panigrahy, and P. K. Sahu, "Denoising of Electrocardiogram (ECG) Signal by using Empirical Mode Decomposition (EMD) with Non-Local Mean (NLM) Technique," Biocybernetics and Biomedical Engineering, vol. 38, no. 2, pp. 297- 312, 2018.
[CrossRef] [Google Scholar] [Publisher Link]
[46] Yunzheng Wang et al., "Recent Advances in Real-Time Spectrum Measurement of Soliton Dynamics by Dispersive Fourier Transformation," Reports on Progress in Physics, vol. 83, no. 11, 2020.
[CrossRef] [Google Scholar] [Publisher Link]
[47] Gautier Marti et al., "A Review of Two Decades of Correlations, Hierarchies, Networks and Clustering in Financial Markets," Progress in Information Geometry, pp. 245-274, 2021.
[CrossRef] [Google Scholar] [Publisher Link]
[48] Solomon Osarumwense Alile, "An Ischemic Heart Disease Prediction Model Based on Observed Symptoms using Machine Learning," International Journal of Computer Engineering in Research Trends, vol. 7, no. 9, pp. 9-22, 2020.
[Google Scholar] [Publisher Link]
[49] Tae-Ki An, and Moon-Hyun Kim, "A New Diverse AdaBoost Classifier," 2010 International Conference on Artificial Intelligence and Computational Intelligence, pp. 359-363, 2010.
[CrossRef] [Google Scholar] [Publisher Link]
[50] Benedetto Barabino et al., "An Integrated Approach to Select Key Quality Indicators in Transit Services," Social Indicators Research, pp. 1-36, 2020.
[CrossRef] [Google Scholar] [Publisher Link]
[51] S. Celin, and K. Vasanth, "ECG Signal Classification using Various Machine Learning Techniques," Journal of Medical Systems, vol. 42, no. 12, pp. 1-11, 2018.
[CrossRef] [Google Scholar] [Publisher Link]
[52] G. B. Moody, and R. G. Mark, "The Impact of the MIT-BIH Arrhythmia Database," IEEE Engineering in Medicine and Biology Magazine, vol. 20, no. 3, pp. 45-50, 2001.
[CrossRef] [Google Scholar] [Publisher Link]
[53] M. M. Venkata Chalapathi et al., "Ensemble Learning by High-Dimensional Acoustic Features for Emotion Recognition from Speech Audio Signal," Security and Communication Networks, vol. 2022, 2022.
[CrossRef] [Google Scholar] [Publisher Link]
[54] T. S. Lugovaya, "Biometric Human Identification Based on ECG," Master's Thesis, Faculty of Computing Technologies and Informatics, Electrotechnical University, 2005.
[Publisher Link]
[55] Raghavendra Badiger, and M. Prabhakar, “ASCNet-ECG: Deep Autoencoder Based Attention Aware Skip Connection Network for ECG Filtering,” International Journal of Engineering Trends and Technology, vol. 71, no. 2, pp. 382-398, 2023.
[CrossRef] [Google Scholar] [Publisher Link]
[56] Steven Smith, Digital Signal Processing: A Practical Guide for Engineers and Scientists, Newnes, An Imprint of Elsevier Science, 2013.
[Google Scholar] [Publisher Link]
[57] Python (n.d.), [Online]. Available: https://www.python.org/
[58] V. R. S. Rajesh Kumar, and A. Sivanantharaja, "Feed Forward Neural Network Optimized using PSO and GSA for the Automatic Classification of Heartbeat," Middle-East Journal of Scientific Research, vol. 23, no. 5, pp. 896-901, 2015.
[Google Scholar] [Publisher Link]
[59] S. Arivoli, "A Novel SVM Neural Network Based Clinical Diagnosis of Cardiac Rhythm," SSRG International Journal of Medical Science, vol. 4, no. 1, pp. 8-14, 2017.
[CrossRef] [Google Scholar] [Publisher Link]
[60] C. Haritha, M. Ganesan, and E. P. Sumesh, "A Survey on Modern Trends in ECG Noise Removal Techniques," 2016 International Conference on Circuit, Power and Computing Technologies (ICCPCT), pp. 1-7, 2016.
[CrossRef] [Google Scholar] [Publisher Link]
[61] R. S. Khandpur, Handbook of Biomedical Instrumentation, McGraw Hill Education (India) Private Limited, 3rd Edition, 2014.
[Google Scholar] [Publisher Link]
[62] P. K. Dash, "Electrocardiogram Monitoring," Indian Journal of Anaesthesia, vol. 46, no. 4, pp. 251-260, 2002.
[Google Scholar] [Publisher Link]