Sensor-Assisted Machine Learning Models Approach to Equipoise Renewable Energy using Microgrids
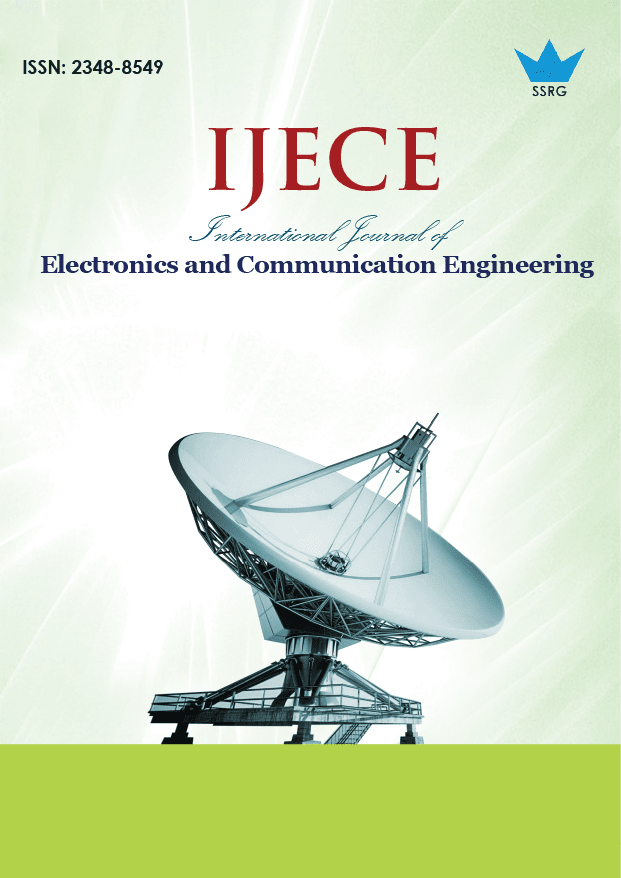
International Journal of Electronics and Communication Engineering |
© 2023 by SSRG - IJECE Journal |
Volume 10 Issue 7 |
Year of Publication : 2023 |
Authors : Shreenidhi H S, Narayana Swamy Ramaiah |
How to Cite?
Shreenidhi H S, Narayana Swamy Ramaiah, "Sensor-Assisted Machine Learning Models Approach to Equipoise Renewable Energy using Microgrids," SSRG International Journal of Electronics and Communication Engineering, vol. 10, no. 7, pp. 63-73, 2023. Crossref, https://doi.org/10.14445/23488549/IJECE-V10I7P107
Abstract:
Sensor-assisted machine learning framework for renewable energy balancing in microgrids (MG). Integrating renewable energy sources into microgrid systems brings the challenge of managing renewable energy generation's intermittent and variable nature. The proposed framework leverages sensor technology to collect real-time data on energy generation, consumption, and grid conditions. Machine learning algorithms are then applied to analyze this data and optimize energy flow within the microgrid. The proposed machine learning models can use historical data to forecast renewable energy generation and demand, enabling proactive energy management (PEM). The framework also incorporates optimization techniques to allocate energy efficiently, considering storage capacity, load demand, and grid stability factors. The sensorassisted machine learning algorithm enhances microgrid systems' reliability, precision, fi-score, recall, and support by dynamically adapting energy generation and consumption based on real-time conditions. This framework represents a significant step towards achieving sustainable and resilient microgrid operations by maximizing the utilization of renewable energy resources. The optimized results by sensing the physical quantity achieved an accuracy of 70%.
Keywords:
Sensor, Machine learning, Proactive energy management, Microgrids, Renewable energy.
References:
[1] Lijun He et al., “A Day-Ahead Scheduling Optimization Model of Multi-Microgrid Considering Interactive Power Control,” 2019 4th International Conference on Intelligent Green Building and Smart Grid (IGBSG), Hubei, China, pp. 666-669, 2019.
[CrossRef] [Google Scholar] [Publisher Link]
[2] Esmat Samad, Ali Badri, and Reza Ebrahimpour, “Decentralized Multi-Agent Based Energy Management of Microgrid using Reinforcement Learning,” International Journal of Electrical Power & Energy Systems, vol. 122, p. 106211, 2020.
[CrossRef] [Google Scholar] [Publisher Link]
[3] Puli Dilliswar Reddy, and L. Rama Parvathy, “Prediction Analysis using Random Forest Algorithms to Forecast the Air Pollution Level in a Particular Location,” 2022 3rd International Conference on Smart Electronics and Communication (ICOSEC), Trichy, India, pp. 1585-1589, 2022.
[CrossRef] [Google Scholar] [Publisher Link]
[4] Meijin Lin, and Haoyuan Luo, “Log AdaBoost: Optimizing Polylog Loss Function to Improve the Generalization Performance of AdaBoost,” 2022 37th Youth Academic Annual Conference of Chinese Association of Automation (YAC), Beijing, China, pp. 958-961, 2022.
[CrossRef] [Google Scholar] [Publisher Link]
[5] Yue Wu et al., “Use of Radiomic Features and Support Vector Machine to Discriminate Subjective Cognitive Decline and Healthy Controls,” 2020 42nd Annual International Conference of the IEEE Engineering in Medicine & Biology Society (EMBC), Montreal, QC, Canada, pp. 1762-1765, 2020.
[CrossRef] [Google Scholar] [Publisher Link]
[6] Xiaodong Liang, and Chowdhury Andalib-Bin-Karim, “Harmonics and Mitigation Techniques through Advanced Control in GridConnected Renewable Energy Sources: A Review,” IEEE Transactions on Industry Applications, vol. 54, no. 4, pp. 3100-3111, 2018.
[CrossRef] [Google Scholar] [Publisher Link]
[7] Payman Rezaei et al., “A Novel Energy Management Scheme for a Microgrid with Renewable Energy Sources Considering Uncertainties and Demand Response,” 2022 12th Smart Grid Conference (SGC), Kerman, Iran, pp. 1-5, 2022.
[CrossRef] [Google Scholar] [Publisher Link]
[8] M. F. Roslan et al., “Microgrid Control Methods Toward Achieving Sustainable Energy Management,” Applied Energy, vol. 240, pp. 583-607, 2019.
[CrossRef] [Google Scholar] [Publisher Link]
[9] Jennifer Cronin, Gabrial Anandarajah, and Olivier Dessens, “Climate Change Impacts on the Energy System: A Revie of Trends and Gaps,” Climatic Change, vol. 151, no. 2, pp. 79-93, 2018.
[CrossRef] [Google Scholar] [Publisher Link]
[10] H. C. Bloomfield et al., “Quantifying the Sensitivity of European Power Systems to Energy Scenarios and Climate Change Projections,” Renewable Energy, vol. 164, pp. 1062-1075, 2021.
[CrossRef] [Google Scholar] [Publisher Link]
[11] Ali Q. Al-Shetwi et al., “Grid-Connected Renewable Energy Sources: Review of the Recent Integration Requirements and Control Methods,” Journal of Cleaner Production, vol. 253, p. 119831, 2020.
[CrossRef] [Google Scholar] [Publisher Link]
[12] Siva Prasad Chowdary Machina, Sriranga Suprabhath Koduru, and Sreedhar Madichetty, “Solar Energy Forecasting using Deep Learning Techniques,” 2022 2nd International Conference on Power Electronics & IoT Applications in Renewable Energy and Its Control (PARC), Mathura, India, pp. 1-6, 2022.
[CrossRef] [Google Scholar] [Publisher Link]
[13] Sunil M P, and Hariprasad S A, “Facial Emotion Recognition using a Modified Deep Convolutional Neural Network Based on the Concatenation of XCEPTION and RESNET50 V2,” SSRG International Journal of Electrical and Electronics Engineering, vol. 10, no. 6, pp. 94-105, 2023.
[CrossRef] [Google Scholar] [Publisher Link]
[14] Fermín Rodríguez et al., “Very Short-Term Wind Power Density Forecasting through Artificial Neural Networks for Microgrid Control,” Renewable Energy, vol. 145, pp. 1517-1527, 2020.
[CrossRef] [Google Scholar] [Publisher Link]
[15] L. Maria Anthony Kumar, S. Murugan, and A. Therasa Alphonsa, “Robust Human Activity Recognition using Equilibrium Optimizer with Deep Extreme Learning Machine Model,” SSRG International Journal of Electrical and Electronics Engineering, vol. 10, no. 5, pp. 1-13, 2023.
[CrossRef] [Publisher Link]
[16] Vaibhav Aggarwal et al., “Integration of Waste Management Companies in Micro Grids through Machine Learning,” 2021 Second International Conference on Electronics and Sustainable Communication Systems (ICESC), Coimbatore, India, pp. 1881-1886, 2021.
[CrossRef] [Google Scholar] [Publisher Link]
[17] Beh Xiao Yan et al., “Development of a Self-Adaptive Based Renewable Energy Management in Smart Micro Grid,” 2021 IEEE 15th Malaysia International Conference on Communication (MICC), Malaysia, pp. 54-59, 2021.
[CrossRef] [Google Scholar] [Publisher Link]
[18] Ramya Ravibarathi, and Tamilarasu Viswanathan, “Assimilation of Gesture using 9 Axis Acclerometer Sensor,” SSRG International Journal of Electrical and Electronics Engineering, vol. 5, no. 2, pp. 1-4, 2018.
[CrossRef] [Google Scholar] [Publisher Link]
[19] Jaiganesh P M, and B. Meenakshi Sundaram, “IoT Based Power Monitoring System,” SSRG International Journal of Computer Science and Engineering, vol. 8, no. 4, pp. 4-7, 2021.
[CrossRef] [Publisher Link]
[20] Georgios Tziolis et al., “Comparative Analysis of Machine Learning Models for Short-Term Net Load Forecasting in Renewable Integrated Microgrids,” 2022 2nd International Conference on Energy Transition in the Mediterranean Area (SyNERGY MED), Thessaloniki, Greece, pp. 1-5, 2022.
[CrossRef] [Google Scholar] [Publisher Link]
[21] Nachat Nasser, and Meghdad Fazeli, “Buffered-Microgrid Structure for Future Power Networks; A Seamless Microgrid Control,” IEEE Transactions on Smart Grid, vol. 12, no. 1, pp. 131-140, 2021.
[CrossRef] [Google Scholar] [Publisher Link]
[22] Guishuo Wang, Xiaoli Wang, and Xiang Gao, “Improved Seamless Switching Control Strategy for AC/DC Hybrid Microgrid,” IEEE Access, vol. 9, pp. 55790-55801, 2021.
[CrossRef] [Google Scholar] [Publisher Link]
[23] U. S. Department of Energy Combined Heat & Power and Microgrid Installation Databases, [Online]. Available: https://doe.icfwebservices.com/downloads/microgrid
[24] Bogdan Marinescu et al., “Dynamic Virtual Power Plant: A New Concept for Grid Integration of Renewable Energy Sources,” IEEE Access, vol. 10, pp. 104980-104995, 2022.
[CrossRef] [Google Scholar] [Publisher Link]