Fuzzy Refinement-Based Tissue and Ring Connectivity for Brain Skull Segmentation
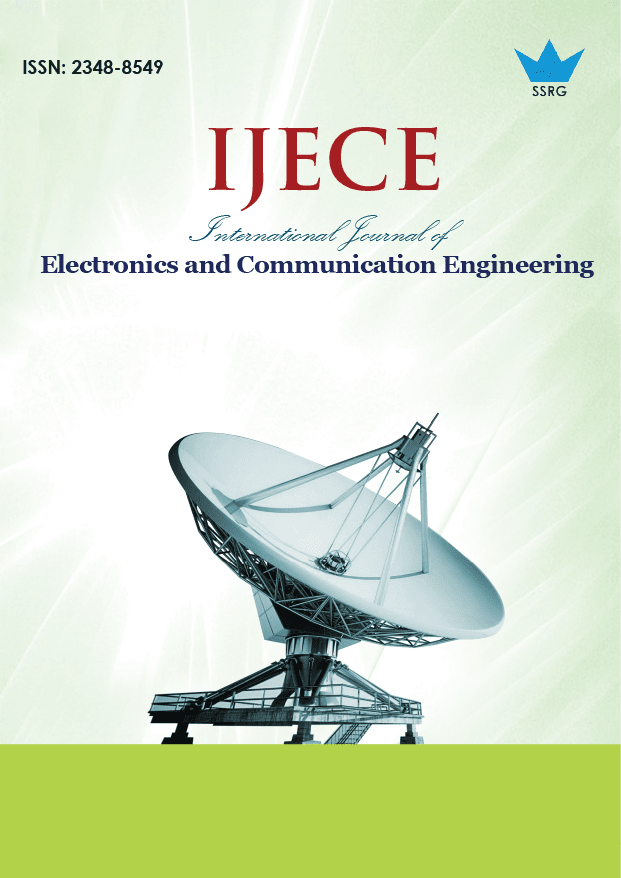
International Journal of Electronics and Communication Engineering |
© 2023 by SSRG - IJECE Journal |
Volume 10 Issue 8 |
Year of Publication : 2023 |
Authors : Nisha Bernad Singh, Victor Jose Marianthiran |
How to Cite?
Nisha Bernad Singh, Victor Jose Marianthiran, "Fuzzy Refinement-Based Tissue and Ring Connectivity for Brain Skull Segmentation," SSRG International Journal of Electronics and Communication Engineering, vol. 10, no. 8, pp. 118-127, 2023. Crossref, https://doi.org/10.14445/23488549/IJECE-V10I8P112
Abstract:
Magnetic Resonance Imaging (MRI) based brain cancer segmentation methods need the skull stripping algorithms as their pre-processing tool. Skull stripping is challenged by less accuracy and high time consumption; hence, an effective skull stripping method is needed for the medical world. In this research, a novel skull stripping method on brain MRI is proposed, which is named 'Skull Stripping in brain MRI using FHECE based enhancement, Fuzzy clustering and Morphological operations (SS_FFM)'. The contribution of this paper is the 'Fusion of Histogram equalization and Edge-based Contrast Enhancement (FHECE)'. The proposed SS_FFM method essentially segments the brain tissue region from the background and skull region of brain MRI. The FHECE method enhances the brain MRI using the novel approach of weighted fusion of Adaptive Histogram Equalization (AHE) and edge-based contrast enhancement. The proposed SS_FFM method is also empowered by a new concept, which is an integrated component based on the three binary clustered outputs along with the 'Tissue and skull ring connectivity detection'. The main advantage of this paper is the independent skull stripping against the 'Tissue and skull ring connectivity' characteristic. Segmentation Accuracy (SA) analysis reveals that the proposed SS_FFM method enhances the SA by 1.39% compared to the second-best method. The proposed method reduces the time consumption by 46.19% compared to the secondbest SS-UNET method. Experimental results in terms of F Score and Segmentation accuracy prove the extended efficiency of the proposed method. Hence, it can be used as a tool for medical practitioners.
Keywords:
Brain skull segmentation, Fuzzy c means, MRI tissue region separation, MRI enhancement, Medical image processing.
References:
[1] Muhammad Assam et al., “An Efficient Classification of MRI Brain Images,” IEEE Access, vol. 9, pp. 33313-33322, 2021.
[CrossRef] [Google Scholar] [Publisher Link]
[2] Siyabonga, and Shira, “Separation from Brain Magnetic Resonance images (MRI) using Multistage Thresholding Technique,” SSRG International Journal of Pharmacy and Biomedical Engineering, vol. 2, no. 3, pp. 9-13, 2015.
[CrossRef] [Publisher Link]
[3] Huile Gao, and Xinguo Jiang, “Progress on the Diagnosis and Evaluation of Brain Tumors,” Cancer Imaging, vol. 13, no. 4, pp. 466-481, 2013.
[CrossRef] [Google Scholar] [Publisher Link]
[4] Ivana Despotović, Bart Goossens, and Wilfried Philips, “MRI Segmentation of the Human Brain: Challenges, Methods, and Applications,” Computational Intelligence Techniques in Medicine, 2015.
[CrossRef] [Google Scholar] [Publisher Link]
[5] Sindhia et al., “Brain Tumor Detection Using MRI by Classification and Segmentation,” SSRG International Journal of Medical Science, vol. 6, no. 3, pp. 12-14, 2019.
[CrossRef] [Google Scholar] [Publisher Link]
[6] P. Kalavathi, and V.B. Prasath, “Methods on skull stripping of MRI head scan images-a review”, Journal of digit imaging, vol. 29, no. 3, pp. 365-379, 2016.
[CrossRef] [Google Scholar] [Publisher Link]
[7] P. Kanmani, P.Marikkannu, and M. Brindha, “A Medical Image Classification using ID3 Classifier,” SSRG International Journal of Computer Science and Engineering, vol. 3, no. 10, pp. 8-11, 2016.
[CrossRef] [Google Scholar] [Publisher Link]
[8] Sylvain Faisan et al., “Topology Preserving Warping of Binary Images Application to Atlas-Based Skull Segmentation,” Medical Image Computing and Computer Assisted Intervention, vol. 5241, pp. 211-218, 2008.
[CrossRef] [Google Scholar] [Publisher Link]
[9] Jong Geun Park, and Chulhee Lee, “Skull Stripping Based on Region Growing for Magnetic Resonance Brain Images,” NeuroImage, vol. 47, no.4, pp. 1394-1407, 2009.
[CrossRef] [Google Scholar] [Publisher Link]
[10] K. Somasundaram, and P. Kalavathi, “A Hybrid Method for Automatic Skull Stripping of Magnetic Resonance Images (MRI) of Human Head Scans using Image Contour,” Second International Conference on Computing Communication and Networking Technologies, Karur, India, pp. 1-5, 2010.
[CrossRef] [Google Scholar] [Publisher Link]
[11] Jin Liu et al., “Spatially Constrained Fuzzy Hyper-Prototype Clustering with Application to Brain Tissue Segmentation,” International Conference on Bioinformatics And Biomedicine, Hong Kong, China, pp. 397-400, 2010.
[CrossRef] [Google Scholar] [Publisher Link]
[12] Payam B. Bijari et al., “Three-Dimensional Coupled-Object Segmentation using Symmetry and Tissue Type Information,” Computerized Medical Imaging and Graphics, vol. 34, no. 3, 236-249, 2010.
[CrossRef] [Google Scholar] [Publisher Link]
[13] Juan Eugenio Iglesias et al., “Agreement-Based Semi-Supervised Learning for Skull Stripping,” Medical Image Computing and Computer Assisted Intervention, vol. 6363, pp. 147–154, 2010.
[CrossRef] [Google Scholar] [Publisher Link]
[14] Yaping Wang et al., “Robust Deformable-Surface-Based Skull-Stripping for Large-Scale Studies,” Medical Image Computing and Computer Assisted Intervention, vol. 6893, pp. 635–642, 2011.
[CrossRef] [Google Scholar] [Publisher Link]
[15] William Speier et al., “Robust Skull Stripping of Clinical Glioblastoma Multiforme Data,” Medical Image Computing and Computer Assisted Intervention, vol. 6893, pp. 659-666, 2011.
[CrossRef] [Google Scholar] [Publisher Link]
[16] Jiayin Zhou et al., “Segmentation of Skull Base Tumors from MRI using a Hybrid Support Vector Machine-Based Method,” Machine Learning in Medical Imaging, vol. 7009, pp. 134-141, 2011.
[CrossRef] [Google Scholar] [Publisher Link]
[17] Vaclav Uher et al., “3D Brain Tissue Selection and Segmentation from MRI,” 36th International Conference on Telecommunications and Signal Processing, Rome, Italy, pp. 839-842, 2013.
[CrossRef] [Google Scholar] [Publisher Link]
[18] P. Kalavathi, “Brain Tissue Segmentation in MR Brain Images using Multiple Otsu's Thresholding Technique,” 8th International Conference on Computer Science and Education, Colombo, Sri Lanka, pp. 639-642, 2013.
[CrossRef] [Google Scholar] [Publisher Link]
[19] Jaya H. Dewan et al., “Brain Tumor Type Identification from MR Images Using Texture Features and Machine Learning Techniques,” International Journal of Engineering Trends and Technology, vol. 71, no. 5, pp. 303-312, 2023.
[CrossRef] [Google Scholar] [Publisher Link]
[20] Wenchao Lv et al., “Reconstruction of Brain Tissue Surface based on Three-Dimensional T1-Weighted MRI Images,” 8th International Congress on Image and Signal Processing, Shenyang, China, pp. 481-486, 2015.
[CrossRef] [Google Scholar] [Publisher Link]
[21] Manit Chansuparp et al., “The Automated Skull Stripping of Brain Magnetic Resonance Images using the Integrated Method,” 8th Biomedical Engineering International Conference, Pattaya, Thailand, pp.1-5, 2015.
[CrossRef] [Google Scholar] [Publisher Link]