Hybrid Multi-Scale Feature Transform Based Fusion of X-Ray and Radar Image
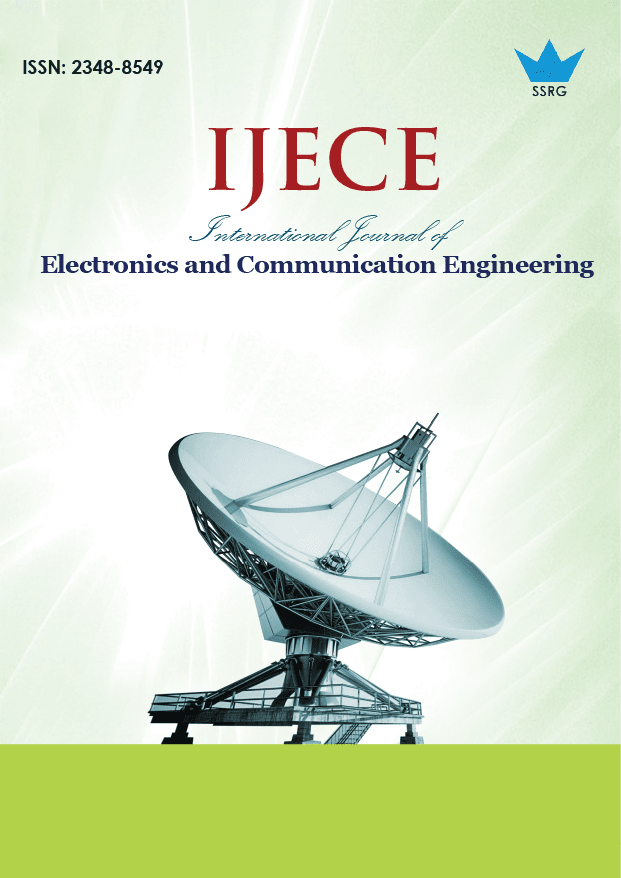
International Journal of Electronics and Communication Engineering |
© 2023 by SSRG - IJECE Journal |
Volume 10 Issue 10 |
Year of Publication : 2023 |
Authors : Gude Ramarao, Chinni.Hima Bindu, T.S.N Murthy |
How to Cite?
Gude Ramarao, Chinni.Hima Bindu, T.S.N Murthy, "Hybrid Multi-Scale Feature Transform Based Fusion of X-Ray and Radar Image," SSRG International Journal of Electronics and Communication Engineering, vol. 10, no. 10, pp. 57-63, 2023. Crossref, https://doi.org/10.14445/23488549/IJECE-V10I10P106
Abstract:
X-ray and radar imaging are two different imaging techniques that can be used for various applications, such as security screening, medical imaging, and geophysical exploration. High-energy X-rays are used to make images of objects by detecting their backscattered radiation. The generated image contains information on the object’s interior structure and composition. Instead, radar imaging employs radio waves to form images by measuring reflected signals’ time delay and intensity. X-ray and radar images are aligned using a feature-based registration method. The goal of feature-based registration is to find corresponding points or features in the pictures and use them to compute the transformation that aligns the images. This paper proposed a Multi-Scale Feature Transform (MSFT) to improve the performance of feature extraction and object recognition tasks. Experimental results using image quality tests demonstrate that Multi-scale transform fusion performs better based on lesser false data, higher colour accuracy, and better image visibility.
Keywords:
Multi-Scale Feature Transform, Feature-based registration, Fusion image, X-Ray and radar image, NDT
References:
[1] Alex Chalmers, “Transmission-Enhanced Backscatter X-Ray Images,” Hard X-Ray and Gamma-Ray Detector Physics III, vol. 4507, pp. 125-131, 2001.
[CrossRef] [Google Scholar] [Publisher Link]
[2] Sanjeevareddy Kolkoori et al., “A New X-Ray Backscatter Imaging Technique for Non-Destructive Testing of Aerospace Materials,” NDT & E International, vol. 70, pp. 41-52, 2015.
[CrossRef] [Google Scholar] [Publisher Link]
[3] Simon Caron-Huot, “Holographic Cameras: An Eye for the Bulk,” Journal of High Energy Physics, vol. 2023, pp. 1-54, 2023.
[CrossRef] [Google Scholar] [Publisher Link]
[4] S.I. Ivashov et al., “Holographic Subsurface Radar as a Device for NDT of Construction Materials and Structures,” Nondestructive Testing of Materials and Structures, pp. 799-804, 2011.
[CrossRef] [Google Scholar] [Publisher Link]
[5] Lingbo Qiao et al., “Exact Reconstruction for Near-Field Three-Dimensional Planar Millimeter-Wave Holographic Imaging,” Journal of Infrared, Millimeter, and Terahertz Waves, vol. 36, pp. 1221-1236, 2015.
[CrossRef] [Google Scholar] [Publisher Link]
[6] Shuang Qiu et al., “Artifact Suppression Using Cross-Circular Polarization for Millimeter-Wave Imaging,” Frontiers in Physics, vol. 11, pp. 1-6, 2023.
[CrossRef] [Google Scholar] [Publisher Link]
[7] S. Kolkoori et al., “Novel X-Ray Backscatter Technique for Detection of Dangerous Materials: Application to Aviation and Port Security,” Journal of Instrumentation, vol. 8, 2013.
[CrossRef] [Google Scholar] [Publisher Link]
[8] Abdulkarim Z. Khalf et al., “Study of Density, Molar Volume, X-Ray Diffraction and Infrared Spectra of Phosphate Glasses,” SSRG International Journal of Applied Physics, vol. 8, no. 2, pp. 16-20, 2021.
[CrossRef] [Publisher Link]
[9] Lorenzo Capineri, “High Resolution Subsurface Imaging with a Holographic Radar Mounted on a Robotic Scanner,” 6 th Internation Congress on Science and Technology for the Safeguard of Cultural Heritage in the Mediterrean Basin, vol. 1, pp. 314-319, 2014.
[Google Scholar] [Publisher Link]
[10] Lorenzo Capineri et al., Holographic and Impulse Subsurface Radar for Landmine and IED Detection, NATO Science for Peace and Security Series. B, Physics and Biophysics, pp. 33-52, 2019.
[Publisher Link]
[11] Velmurugan Subbiah Parvathy, and Sivakumar Pothiraj, “Multi-Modality Medical Image Fusion Using Hybridization of Binary Crow Search Optimization,” Health Care Management Science, vol. 23, pp. 661-669, 2020.
[CrossRef] [Google Scholar] [Publisher Link]
[12] Suzhen Lin et al., “Integrating Model-and Data-Driven Methods for Synchronous Adaptive Multi-Band Image Fusion,” Information Fusion, vol. 54, pp. 145-160, 2020.
[CrossRef] [Google Scholar] [Publisher Link]
[13] Amal Azeroual et al., “Artificial Intelligence Applied to COVID-19 Lung Infection Segmentation from CT Images,” International Journal of Engineering Trends and Technology, vol. 71, no. 7, pp. 124-131, 2023.
[CrossRef] [Publisher Link]
[14] T. Joel, and R. Sivakumar, “An Extensive Review on Despeckling of Medical Ultrasound Images Using Various Transformation Techniques,” Applied Acoustics, vol. 138, pp. 18-27, 2018.
[CrossRef] [Google Scholar] [Publisher Link]
[15] Aparna M. Harale, and Vinayak K. Bairagi, “Computer-Aided Detection (CAD) System for the Detection and Classification of Pulmonary Nodules in Lung Cancer Using CT images,” International Journal of Engineering Trends and Technology, vol. 71, no. 1, pp. 31-40, 2023.
[CrossRef] [Publisher Link]
[16] Aneeta Christopher, R. Hari Kishan, and P.V. Sudeep, Image Reconstruction Using Deep Learning, Machine Learning Algorithms for Signal and Image Processing, 2022.
[CrossRef] [Publisher Link]
[17] Alankrita Aggarwal, et al., A Deep Analysis on the Role of Deep Learning Models Using Generative Adversarial Networks, Blockchain and Deep Learning, pp. 179-197, 2022.
[CrossRef] [Google Scholar] [Publisher Link]
[18] G.N. Hounsfield, “Computerized Transverse Axial Scanning (Tomography): Part 1. Description of System,” The British Journal of Radiology, vol. 46, no. 552, pp. 1015-1088, 1973.
[CrossRef] [Google Scholar] [Publisher Link]
[19] S. Boopathiraja et al., “Computational 2D and 3D Medical Image Data Compression Models,” Archives of Computational Methods in Engineering, vol. 29, pp. 975-1007, 2022.
[CrossRef] [Google Scholar] [Publisher Link]
[20] S.J. Pawan, and Jeny Rajan, “Capsule Networks for Image Classification: A Review,” Neurocomputing, vol. 509, pp. 102-120, 2022.
[CrossRef] [Google Scholar] [Publisher Link]
[21] Sepp Hochreiter, “The Vanishing Gradient Problem during Learning Recurrent Neural Nets and Problem Solutions,” International Journal of Uncertainty, Fuzziness and Knowledge-Based Systems, vol. 6, no. 2, pp. 107-116, 1998.
[CrossRef] [Google Scholar] [Publisher Link]
[22] David J. Brady, Lu Fang, and Zhan Ma, “Deep Learning for Camera Data Acquisition, Control, and Image Estimation,” Advances in Optics and Photonics, vol. 12, no. 4, pp. 787-846, 2020.
[CrossRef] [Google Scholar] [Publisher Link]
[23] Raku Son et al., “Morphomics via Next-generation Electron Microscopy,” Medical Physics, 2021.
[CrossRef] [Google Scholar] [Publisher Link]