A Hybrid Visionary for Unveiling Human Motion in the Face of Occlusion with Mask R-CNN, RNN, and MHT
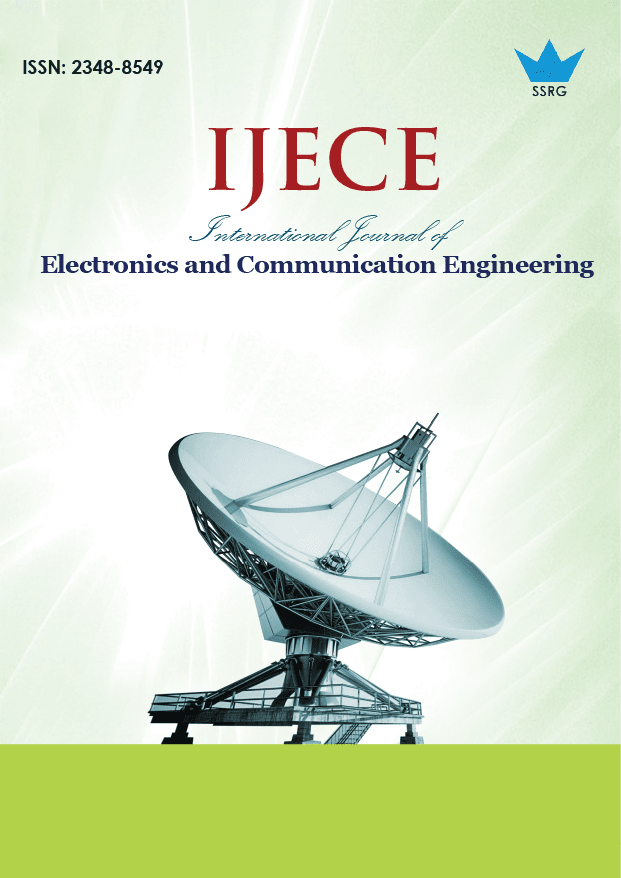
International Journal of Electronics and Communication Engineering |
© 2024 by SSRG - IJECE Journal |
Volume 11 Issue 2 |
Year of Publication : 2024 |
Authors : Jeba Nega Cheltha, Chirag Sharma |
How to Cite?
Jeba Nega Cheltha, Chirag Sharma, "A Hybrid Visionary for Unveiling Human Motion in the Face of Occlusion with Mask R-CNN, RNN, and MHT," SSRG International Journal of Electronics and Communication Engineering, vol. 11, no. 2, pp. 92-102, 2024. Crossref, https://doi.org/10.14445/23488549/IJECE-V11I2P110
Abstract:
Addressing the intricate challenges of Human Motion Detection (HMD), this research presents a pioneering hybrid methodology integrating advanced computer vision and deep learning techniques. Focused primarily on mitigating the impact of occlusion in visual data, the proposed approach employs a Mask Region-based Convolutional Neural Network (Mask R-CNN) for precise motion segmentation. The dual challenges of self-occlusion and partial-occlusion are specifically targeted. The three-fold strategy encompasses motion segmentation, object classification, and tracking algorithms to discern and identify human motion accurately. Motion segmentation involves isolating the moving object within video frames, followed by object classification utilizing a Recurrent Neural Network (RNN) to determine the human presence and to tune the parameter of RNN; this work introduced a novel hybrid Whale Optimization Algorithm and Red Deer Algorithm (WOA-RDA), which gives better convergence speed with high accuracy. To tackle the persistence of occlusion, particularly self-occlusion, Multiple Hypothesis Tracking (MHT) is introduced for robustly tracking human gestures. An innovative aspect of the proposed approach lies in the integration of an RNN trained with 2D representations of 3D skeletal motion, enhancing the model’s understanding of complex human movements. The proposed methodology is rigorously evaluated on diverse datasets, incorporating scenarios with and without occlusion. Experimental results underscore the effectiveness of the hybrid approach, showcasing its ability to accurately identify human motion under varying conditions, thereby advancing the field of human motion detection.
Keywords:
Human Motion Detection, Mask R-CNN, Multiple Hypothesis Tracking, Occlusion, RNN.
References:
[1] Ying Liu et al., “Human Motion Image Detection and Tracking Method Based on Gaussian Mixture Model and CAMSHIFT,” Microprocessors and Microsystems, vol. 82, 2021.
[CrossRef] [Google Scholar] [Publisher Link]
[2] Ivan Mutis, Abhijeet Ambekar, and Virat Joshi, “Real-Time Space Occupancy Sensing and Human Motion Analysis Using Deep Learning for Indoor Air Quality Control,” Automation in Construction, vol. 116, 2020.
[CrossRef] [Google Scholar] [Publisher Link]
[3] Md. Milon Islam, Md. Repon Islam, and Md. Saiful Islam, “An Efficient Human Computer Interaction through Hand Gesture Using Deep Convolutional Neural Network,” SN Computer Science, vol. 1, pp. 1-9, 2020.
[CrossRef] [Google Scholar] [Publisher Link]
[4] Xiangui Bu, “Human Motion Gesture Recognition Algorithm in Video Based on Convolutional Neural Features of Training Images,” IEEE Access, vol. 8, pp. 160025-160039, 2020.
[CrossRef] [Google Scholar] [Publisher Link]
[5] Yubin Wu et al., “Hybrid Motion Model for Multiple Object Tracking in Mobile Devices,” IEEE Internet of Things Journal, vol. 10, no. 6, pp. 4735-4748, 2023.
[CrossRef] [Google Scholar] [Publisher Link]
[6] Jilong Wang, Chunhong Lu, and Kun Zhang, “Textile‐Based Strain Sensor for Human Motion Detection,” Energy & Environmental Materials, vol. 3, no. 1, pp. 80-100, 2020.
[CrossRef] [Google Scholar] [Publisher Link]
[7] Fatemeh Serpush et al., “Wearable Sensor-Based Human Activity Recognition in the Smart Healthcare System,” Computational Intelligence and Neuroscience, vol. 2022, pp. 1-31, 2022.
[CrossRef] [Google Scholar] [Publisher Link]
[8] Carolin Helbig et al., “Wearable Sensors for Human Environmental Exposure in Urban Settings,” Current Pollution Reports, vol. 7, pp. 417-433, 2021.
[CrossRef] [Google Scholar] [Publisher Link]
[9] Sakorn Mekruksavanich, and Anuchit Jitpattanakul, “Biometric User Identification Based on Human Activity Recognition Using Wearable Sensors: An Experiment Using Deep Learning Models,” Electronics, vol. 10, no. 3, pp. 1-21, 2021.
[CrossRef] [Google Scholar] [Publisher Link]
[10] Miao He, Guangming Song, and Zhong Wei, “Human Behavior Feature Representation and Recognition Based on Depth Video,” Journal of Web Engineering, vol. 19, no. 5-6, pp. 883-902, 2020.
[CrossRef] [Google Scholar] [Publisher Link]
[11] Thamer Alanazi, Khalid Babutain, and Ghulam Muhammad, “A Robust and Automated Vision-Based Human Fall Detection System Using 3D Multi-Stream CNNs with an Image Fusion Technique,” Applied Sciences, vol. 13, no. 12, pp. 1-20, 2023.
[CrossRef] [Google Scholar] [Publisher Link]
[12] Jing Zhao et al., “Reconstructing Clear Image for High-Speed Motion Scene with a Retina-Inspired Spike Camera,” IEEE Transactions on Computational Imaging, vol. 8, pp. 12-27, 2021.
[CrossRef] [Google Scholar] [Publisher Link]
[13] Snehasis Mukherjee, Leburu Anvitha, and T. Mohana Lahari, “Human Activity Recognition in RGB-D Videos by Dynamic Images,” Multimedia Tools and Applications, vol. 79, pp. 19787-19801, 2020.
[CrossRef] [Google Scholar] [Publisher Link]
[14] Matteo Zago et al., “3D Tracking of Human Motion Using Visual Skeletonization and Stereoscopic Vision,” Frontiers in Bioengineering and Biotechnology, vol. 8, pp. 1-11, 2020.
[CrossRef] [Google Scholar] [Publisher Link]
[15] Monica Gruosso, Nicola Capece, and Ugo Erra, “Human Segmentation in Surveillance Video with Deep Learning,” Multimedia Tools and Applications, vol. 80, pp. 1175-1199, 2021.
[CrossRef] [Google Scholar] [Publisher Link]
[16] Ghazaleh Khodabandelou et al., “A Fuzzy Convolutional Attention-Based GRU Network for Human Activity Recognition,” Engineering Applications of Artificial Intelligence, vol. 118, 2023.
[CrossRef] [Google Scholar] [Publisher Link]
[17] Naoya Yoshimura et al., “Acceleration-Based Activity Recognition of Repetitive Works with Lightweight Ordered-Work Segmentation Network,” Proceedings of the ACM on Interactive, Mobile, Wearable and Ubiquitous Technologies, vol. 6, no. 2, pp. 1-39, 2022.
[CrossRef] [Google Scholar] [Publisher Link]
[18] Chengqun Song et al., “Spatial-Temporal 3D Dependency Matching with Self-Supervised Deep Learning for Monocular Visual Sensing,” Neurocomputing, vol. 481, pp. 11-21, 2022.
[CrossRef] [Google Scholar] [Publisher Link]
[19] Xiaoli Liu et al., “TrajectoryCNN: A New Spatio-Temporal Feature Learning Network for Human Motion Prediction,” IEEE Transactions on Circuits and Systems for Video Technology, vol. 31, no. 6, pp. 2133-2146, 2021.
[CrossRef] [Google Scholar] [Publisher Link]
[20] Marcin Woźniak et al., “Body Pose Prediction Based on Motion Sensor Data and Recurrent Neural Network,” IEEE Transactions on Industrial Informatics, vol. 17, no. 3, pp. 2101-2111, 2021.
[CrossRef] [Google Scholar] [Publisher Link]
[21] I.A. Bustoni et al., “Classification Methods Performance on Human Activity Recognition,” Journal of Physics: Conference Series, vol. 1456, pp. 1-9, 2020.
[CrossRef] [Google Scholar] [Publisher Link]
[22] Siqi Cai et al., “Automatic Detection of Compensatory Movement Patterns by a Pressure Distribution Mattress Using Machine Learning Methods: A Pilot Study,” IEEE Access, vol. 7, pp. 80300-80309, 2019.
[CrossRef] [Google Scholar] [Publisher Link]
[23] X. Wang, and S. Hosseinyalamdary, “Human Detection Based on a Sequence of Thermal Images Using Deep Learning,” The International Archives of the Photogrammetry, Remote Sensing and Spatial Information Sciences, vol. 42, pp. 127-132, 2019.
[CrossRef] [Google Scholar] [Publisher Link]
[24] Manahil Waheed et al., “An LSTM-Based Approach for Understanding Human Interactions Using Hybrid Feature Descriptors over Depth Sensors,” IEEE Access, vol. 9, pp. 167434-167446, 2021.
[CrossRef] [Google Scholar] [Publisher Link]
[25] Divya Gaur, and Sanjay Kumar Dubey, “Development of Activity Recognition Model Using LSTM-RNN Deep Learning Algorithm,” Journal of Information and Organizational Sciences, vol. 46, no. 2, pp. 277-291, 2022.
[Google Scholar] [Publisher Link]
[26] Alefiya Laturwala et al., “Human Pose Estimation Using Machine Learning,” Journal of Emerging Technologies and Innovative Research, vol. 8, no. 12, pp. 403-406, 2021.
[Publisher Link]
[27] Xunyun Chang, and Liangqing Peng, “Visual Sensing Human Motion Detection System for Interactive Music Teaching,” Journal of Sensors, vol. 2021, pp. 1-10, 2021.
[CrossRef] [Google Scholar] [Publisher Link]
[28] Sha Ji, and Chengde Lin, “Human Motion Pattern Recognition Based on Nano-Sensor and Deep Learning,” Information Technology and Control, vol. 52, no. 3, pp. 776-788, 2023.
[CrossRef] [Google Scholar] [Publisher Link]
[29] George A. Oguntala et al., “Passive RFID Module with LSTM Recurrent Neural Network Activity Classification Algorithm for Ambient-Assisted Living,” IEEE Internet of Things Journal, vol. 8, no. 13, pp. 10953-10962, 2021.
[CrossRef] [Google Scholar] [Publisher Link]
[30] Phat Nguyen Huu, and Tan Phung Ngoc, “Hand Gesture Recognition Algorithm Using SVM and HOG Model for Control of Robotic System,” Journal of Robotics, vol. 2021, pp. 1-13, 2021.
[CrossRef] [Google Scholar] [Publisher Link]
[31] Anzhu Miao, and Feiping Liu, “Application of Human Motion Recognition Technology in Extreme Learning Machine,” International Journal of Advanced Robotic Systems, vol. 18, no. 1, 2021.
[CrossRef] [Google Scholar] [Publisher Link]
[32] William Taylor et al., “An Intelligent Non-Invasive Real-Time Human Activity Recognition System for Next-Generation Healthcare,” Sensors, vol. 20, no. 9, pp. 1-20, 2020.
[CrossRef] [Google Scholar] [Publisher Link]
[33] Endang Sri Rahayu et al., “Human Activity Classification Using Deep Learning Based on 3D Motion Feature,” Machine Learning with Applications, vol. 12, 2023.
[CrossRef] [Google Scholar] [Publisher Link]
[34] Yong Zhang et al., “Human Activity Recognition Based on Motion Sensor Using U-Net,” IEEE Access, vol. 7, pp. 75213-75226, 2019.
[CrossRef] [Google Scholar] [Publisher Link]
[35] Fabio Carrara et al., “LSTM-Based Real-Time Action Detection and Prediction in Human Motion Streams,” Multimedia Tools and Applications, vol. 78, pp. 27309-27331, 2019.
[CrossRef] [Google Scholar] [Publisher Link]
[36] Rajiv Vincent et al., “Human Activity Recognition Using LSTM/BiLSTM,” International Journal of Advanced Science and Technology, vol. 29, no. 4, pp. 7468-7474, 2020.
[Google Scholar] [Publisher Link]