VSS-LMS Algorithms for Multichannel System Identification using Volterra Filtering
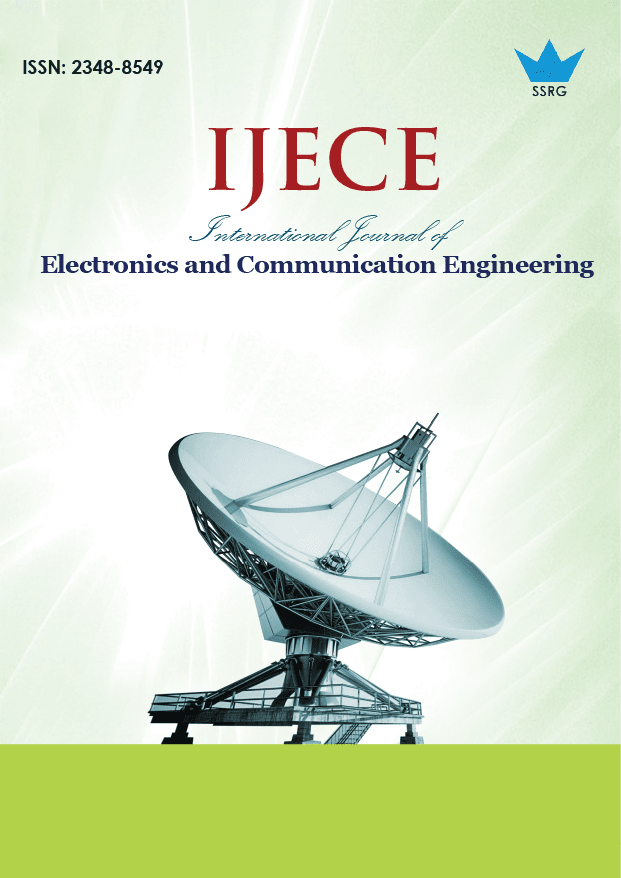
International Journal of Electronics and Communication Engineering |
© 2015 by SSRG - IJECE Journal |
Volume 2 Issue 5 |
Year of Publication : 2015 |
Authors : Sandipta Dutta Gupta and A.K. Kohli |
How to Cite?
Sandipta Dutta Gupta and A.K. Kohli, "VSS-LMS Algorithms for Multichannel System Identification using Volterra Filtering," SSRG International Journal of Electronics and Communication Engineering, vol. 2, no. 5, pp. 10-18, 2015. Crossref, https://doi.org/10.14445/23488549/IJECE-V2I5P106
Abstract:
This paper propounds the adaptive polynomial filtering deploying the multifarious variable step-size least mean square (VSS-LMS) algorithms for the nonlinear Volterra multichannel system identification, and all are compared with a fixed step-size Volterra least mean square (VLMS) algorithm, under the various noise constraints comprising an individual signal-to-noise ratio (SNR). The VSS-LMS algorithm corroborates steady behaviour during convergence, and the stepsize of the adaptive filter is altered in compliance with a gradient descent algorithm delineated to abate the squared estimation error in the course of each iteration, and it also revamps tracking rendition in the smoothly time-varying environments to the choice of the parameters and the boundaries of adaptive filter. In multitudinous practical implementations, the autocorrelation matrix of the input signal has the immense eigenvalue spread in the manifestation of nonlinear Volterra filter than in respect of the linear impulse response filter. In such circumstances, an adaptive step-size is a pertinent option to mitigate the unpropitious effects of eigenvalue spread on the convergence of VLMS adaptive algorithm. The simulation results are exhibited to reinforce the analysis, which compare the VSS-LMS algorithms with fixed step-size of the second-order Volterra filter, and also substantiate that the VSS-LMS algorithms are more robust than the fixed step-size algorithm when the input noise is logistic chaotic type.
Keywords:
least mean square (LMS), minimum mean square error (MMSE), system identification, variable step-size least mean square (VSS-LMS), Volterra filter.
References:
[1] V. John Mathews, Adaptive Polynomial Filters, IEEE Signal Process. Mag., vol. 8, pp. 10-26, Jul. 1991.
[2] A. Stenger, L. Trautmann, and R. Rabenstein, Adaptive Volterra filters for nonlinear acoustic echo cancellation, University of Erlangen, Germany, 1999.
[3] L.F.C. Pessoa, MRL-Filters: A general class of nonlinear systems and their optimal design for image processing, IEEE Trans. On Image Process., vol. 7, no. 7, pp. 966-978, Jul. 1998.
[4] E.J. Coyle and J.H. Lin, Stack filters and the mean absolute error criterion, IEEE Trans. Acoust. Speech Signal Process., vol. 36, pp. 1244-1254, Aug. 1988.
[5] A.K. Kohli and A. Rai, Numeric variable forgetting factor RLS algorithm for second-order Volterra filtering, Circuit Syst. Signal Process., vol. 32, no.1, pp. 223-232, Feb. 2013.
[6] L. Tan and J. Jiang, Adaptive Volterra filters for active control of nonlinear noise processes,IEEE Trans. Signal Process., vol. 49, no. 8, pp. 1667-1676, Aug. 2001.
[7] V.J. Mathews and G.L. sicuranza, Polynomial Signal Processing, John Wiley & Sons Inc., 2000.
[8] M. Schetzen, The Volterra and Wiener Theories of Nonlinear System, Wiley, New York, 1980.
[9] D.P. Das, S.R. Mohapatra, A. Routray and T.K. Basu, Filtered-s LMS algorithm for multichannel active control of nonlinear processes, IEEE Trans. on Speech and Audio Process., vol. 14, no. 5, pp. 1875-1879, Sep. 2006.
[10] S.M. Kuo and D.R. Morgan, Active noise control systems-Algorithms and DSP implementations, New York: Wiley, 1996.
[11] P. Strauch and B. Mulgrew, Active control of nonlinear noise processes in a linear duct,IEEE Trans. Signal Process., vol. 46, no. 9, pp. 2404-2412, Sep. 1998.
[12] M. Bouchard, B. Paillard, and C. T. L. Dinh, Improved training of neural networks for the nonlinear active noise control of sound and vibration,IEEE Trans. Neural Netw., vol.10, no. 2, pp. 391-401, Mar. 1999.
[13] D.P. Das and G. Panda, Active mitigation of nonlinear noise processes using a novel filtered-s LMS algorithm,IEEE Trans. Speech Audio Process., vol. 12, no. 3, pp. 313-322, May 2004.
[14] G.L. Sicuraranza and A.C. Carini, Filtered-X affine projection algorithm for multichannel active noise control using second-order Volterra filters,IEEE Signal Process. Lett., vol. 11, no. 11, pp. 853-857, Nov. 2004.
[15] B. Farhang- Boroujeny, Adaptive Filters: Theory and Applications, John Wiley & Sons, New York, NY, USA, 1998.
[16] Y. Zhang, N. Li, J. A. Chambers, and Y. Hao, New gradient-based variable step-size LMS algorithms, Journal on Advances in Signal Process., vol. 1, Feb.2008.
[17] S. Karni and G. Zeng, A new convergence factor for adaptive filters, IEEE Trans. on Signal Process., vol. 40, no. 7, pp. 1633-1642, Aug. 1989.
[18] R. H. Kwong and E. W. Johnson, A variable step-size LMS algorithm,IEEE Trans.on Signal Process., vol. 40, no. 7, pp. 1633-1642, Jul. 1992.
[19] V. J. Mathews and Z. Xie, A stochastic gradient adaptive filter with gradient adaptive step-size,IEEE Trans. on Signal Process., vol. 41, no. 6, pp. 2075-2087, Jun. 1993.
[20] T. Aboulnasr and K. Mayyas, A robust variable step-size LMS-type algorithm: analysis and simulations,IEEE Trans. on Signal Process., vol. 45, no. 3, pp. 631-639, Jan. 1997.
[21] D. I. Pazaitis and A. G. Constantinides, A novel kurtosis driven variable step-size adaptive algorithmIEEE Trans. on Signal Process., vol. 47, no. 3, pp. 864-872, Mar. 1999.
[22] W. P. Ang and B. Farhang- Boroujeny, A new class of gradient adaptive step-size LMS algorithms,IEEE Trans. on Signal Process., vol. 49, no. 4, pp. 805-810, Apr. 2001.
[23] A. Rai and A. K. Kohli, Adaptive polynomial filtering using generalised variable step-size least mean pth power (LMP) algorithm,Circuit System and Signal Process., vol. 33, no. 12, pp. 3931-3947, Dec. 2014.
[24] G. V. Raz and B. V. Veen, Baseband Volterra filters for implementing carrier based nonlinearities, IEEE Trans. Signal Process., vol. 46, pp. 103-114, Jan. 1998.
[25] T. Ogunfunmi, Adaptive nonlinear system identification: Volterra and Weiner model approches, Springer, Berlin, 2007.
[26] D. T. Westwick and R. E. Kearney, Identification of Nonlinear Physiological Systems, Wiley, New York, 2003.
[27] T. Ogunfunmi and T. Drullinger, Equalization of nonlinear channels using a Volterra-based nonlinear adaptive filter, in Proceedings of IEEE International Midwest Symposium on Circuits and Systems, Seoul, South Korea, pp. 1-4, Jan. 2011.