A Comparative Analysis of Deep Learning Models in Diverse AI Healthcare Applications
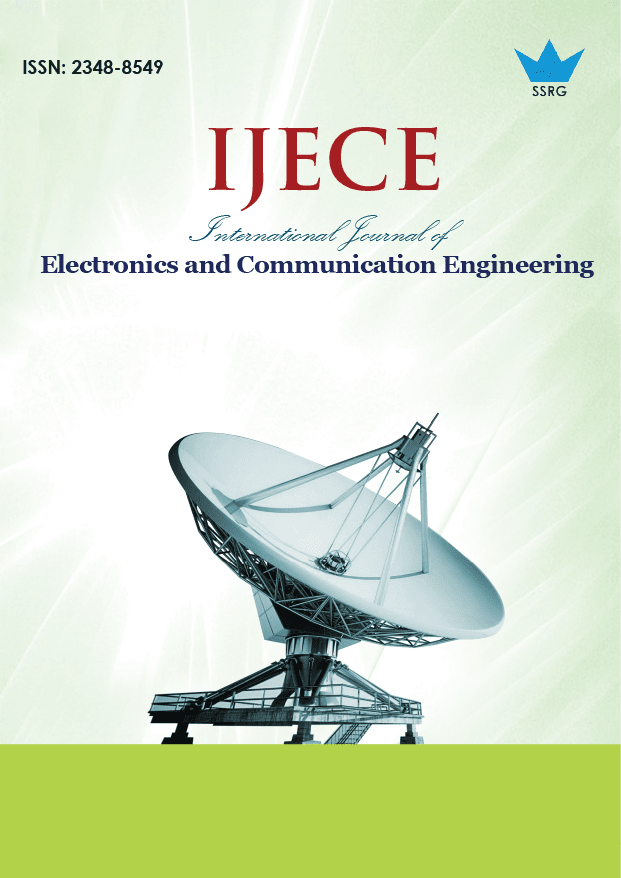
International Journal of Electronics and Communication Engineering |
© 2025 by SSRG - IJECE Journal |
Volume 12 Issue 3 |
Year of Publication : 2025 |
Authors : S. Hemalatha, Kiran Mayee Adavala, S.N. Chandra Shekhar, Pullela SVVSR Kumar, A.R. Venkataramanan, D. Naga Malleswari |
How to Cite?
S. Hemalatha, Kiran Mayee Adavala, S.N. Chandra Shekhar, Pullela SVVSR Kumar, A.R. Venkataramanan, D. Naga Malleswari, "A Comparative Analysis of Deep Learning Models in Diverse AI Healthcare Applications," SSRG International Journal of Electronics and Communication Engineering, vol. 12, no. 3, pp. 116-125, 2025. Crossref, https://doi.org/10.14445/23488549/IJECE-V12I3P111
Abstract:
This review article made the comparative analysis quantitatively evaluates four applications of deep learning in healthcare: tuberculosis detection in chest radiography, skin cancer classification, Electronic Health Record (EHR) analysis, and drug discovery. The studies exhibit distinct model architectures and metrics, with tuberculosis and skin cancer classification achieving AUC values of 0.99 and above 0.91, respectively, using CNNs optimized with transfer learning and data augmentation. EHR analysis, utilizing CNN and RNN hybrids, reports AUCs between 0.70 and 0.85 for tasks like disease progression and patient readmission, demonstrating the variability introduced by heterogeneous, sequential data. Drug discovery models employ RNNs for molecular sequence prediction, highlighting a conceptual framework rather than specific performance metrics. The findings indicate that image-based models excel in quantitative performance and scalability, while models for EHR and molecular data face challenges in standardization and interpretability. This analysis underscores the need for data harmonization and explainability to enhance the clinical readiness of deep learning across diverse healthcare domains.
Keywords:
Deep Learning, Healthcare, Tuberculosis detection, Skin cancer classification, Electronic Health Records (EHR), CNNs, RNNs, Artificial Intelligence (AI).
References:
[1] Andre Esteva et al., “Dermatologist-Level Classification of Skin Cancer with Deep Neural Networks,” Nature, vol. 542, pp. 115-118, 2017.
[CrossRef] [Google Scholar] [Publisher Link]
[2] Alvin Rajkomar, Jeffrey Dean, and Isaac Kohane, “Machine Learning in Medicine,” The New England Journal of Medicine, vol. 380, no. 14, pp. 1347-1358, 2019.
[CrossRef] [Google Scholar] [Publisher Link]
[3] Eric J. Topol, “High-Performance Medicine: The Convergence of Human and Artificial Intelligence,” Nature Medicine, vol. 25, pp. 44-56, 2019.
[CrossRef] [Google Scholar] [Publisher Link]
[4] Salman Razzaki et al., “A Comparative Study of Artificial Intelligence and Human Doctors for the Purpose of Triage and Diagnosis,” arXiv Preprint, pp. 1-15, 2018.
[CrossRef] [Google Scholar] [Publisher Link]
[5] Kit-Kay Mak, and Mallikarjuna Rao Pichika, “Artificial Intelligence in Drug Development: Present Status and Future Prospects,” Drug Discovery Today, vol. 24, no. 3, pp. 773-780, 2019.
[CrossRef] [Google Scholar] [Publisher Link]
[6] Daniel A. Hashimoto et al., “Artificial Intelligence in Surgery: Promises and Perils,” Annals of Surgery, vol. 268, no. 1, pp. 70-76, 2018.
[CrossRef] [Google Scholar] [Publisher Link]
[7] Fei Jiang et al., “Artificial Intelligence in Healthcare: Past, Present and Future,” Stroke and Vascular Neurology, vol. 2, no. 4, pp. 230-243, 2017.
[CrossRef] [Google Scholar] [Publisher Link]
[8] Deekshaa Khanna, “Use of Artificial Intelligence in Healthcare and Medicine,” International Journal of Innovations in Engineering Research and Technology, vol. 5, no. 12, pp. 1-4, 2018.
[Google Scholar] [Publisher Link]
[9] Sirina Keesara, Andrea Jonas, and Kevin Schulman, “Covid-19 and Health Care’s Digital Revolution,” The New England Journal of Medicine, vol. 382, no. 23, pp. 1-3, 2020.
[CrossRef] [Google Scholar] [Publisher Link]
[10] Reed T. Sutton et al., “An Overview of Clinical Decision Support Systems: Benefits, Risks, and Strategies for Success,” NPJ Digital Medicine, vol. 3, pp. 1-10, 2020.
[CrossRef] [Google Scholar] [Publisher Link]
[11] Ziad Obermeyer, and Ezekiel J. Emanuel, “Predicting the Future-Big data, Machine Learning, and Clinical Medicine,” The New England Journal of Medicine, vol. 375, no. 13, pp. 1216-1219, 2016.
[CrossRef] [Google Scholar] [Publisher Link]
[12] Benjamin Shickel et al., “Deep EHR: A Survey of Recent Advances in Deep Learning Techniques for Electronic Health Record (EHR) Analysis,” IEEE Journal of Biomedical and Health Informatics, vol. 22, no. 5, pp. 1589-1604, 2018.
[CrossRef] [Google Scholar] [Publisher Link]
[13] Paras Lakhani, and Baskaran Sundaram, “Deep Learning at Chest Radiography: Automated Classification of Pulmonary Tuberculosis by Using Convolutional Neural Networks,” Radiology, vol. 284, no. 2, pp. 574-582, 2017.
[CrossRef] [Google Scholar] [Publisher Link]
[14] Guang-Zhong Yang et al., “Medical Robotics-Regulatory, Ethical, and Legal Considerations for Increasing Levels of Autonomy,” Science Robotics, vol. 2, no. 4, pp. 1-2, 2017.
[CrossRef] [Google Scholar] [Publisher Link]
[15] Alexander Selvikvåg Lundervold, and Arvid Lundervold, “An Overview of Deep Learning in Medical Imaging Focusing on MRI,” Journal of Medical Physics, vol. 29, no. 2, pp. 102-127, 2019.
[CrossRef] [Google Scholar] [Publisher Link]
[16] Yogesh K. Dwivedi et al., “Artificial Intelligence (AI): Multidisciplinary Perspectives on Emerging Challenges, Opportunities, and Agenda for Research, Practice and Policy,” International Journal of Information Management, vol. 57, 2021.
[CrossRef] [Google Scholar] [Publisher Link]
[17] Yasunari Matsuzaka, and Ryu Yashiro, “Applications of Deep Learning for Drug Discovery Systems with BigData,” BioMedInformatics, vol. 2, no. 4, pp. 603-624, 2022.
[CrossRef] [Google Scholar] [Publisher Link]
[18] Elias Hossain et al., “Natural Language Processing in Electronic Health Records in Relation to Healthcare Decision-Making: A Systematic Review,” Computers in Biology and Medicine, vol. 155, 2023.
[CrossRef] [Google Scholar] [Publisher Link]
[19] Badawy, Mohammed, Nagy Ramadan, and Hesham Ahmed Hefny et al., “Healthcare Predictive Analytics Using Machine Learning and Deep Learning Techniques: A Survey,” Journal of Electrical Systems and Information Technology, vol. 10, no. 1, pp. 1-45, 2023.
[CrossRef] [Google Scholar] [Publisher Link]
[20] Mohamed Khalifa, and Mona Albadawy, “AI in Diagnostic Imaging: Revolutionising Accuracy and Efficiency,” Computer Methods and Programs in Biomedicine Update, vol. 5, 2024.
[CrossRef] [Google Scholar] [Publisher Link]
[21] Nicholas J. Schork, Artificial Intelligence and Personalized Medicine, Precision Medicine in Cancer Therapy, vol. 178, pp. 265-283, 2019.
[CrossRef] [Google Scholar] [Publisher Link]
[22] Ahmed M. Alhuwaydi, “Exploring the Role of Artificial Intelligence in Mental Healthcare: Current Trends and Future Directions-A Narrative Review for a Comprehensive Insight,” Risk Management and Healthcare Policy, vol. 17, pp. 1339-1348, 2024.
[CrossRef] [Google Scholar] [Publisher Link]
[23] Muhammad Rafiq et al., “Predictive Analytics Support for Complex Chronic Medical Conditions: An Experience-Based Co-Design Study of Physician Managers’ Needs and Preferences,” International Journal of Medical Informatics, vol. 187, 2024.[CrossRef] [Google Scholar] [Publisher Link]