Soil Testing Using Image Processing
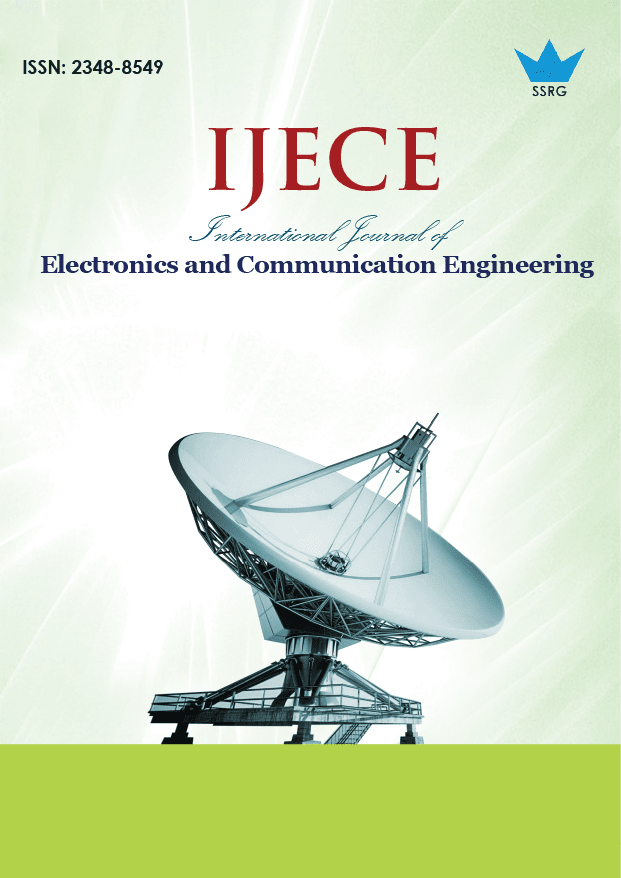
International Journal of Electronics and Communication Engineering |
© 2025 by SSRG - IJECE Journal |
Volume 12 Issue 4 |
Year of Publication : 2025 |
Authors : Shivani Sisodia, Saurabh Dhyani |
How to Cite?
Shivani Sisodia, Saurabh Dhyani, "Soil Testing Using Image Processing," SSRG International Journal of Electronics and Communication Engineering, vol. 12, no. 4, pp. 1-7, 2025. Crossref, https://doi.org/10.14445/23488549/IJECE-V12I4P101
Abstract:
Precision agriculture is a globally growing practice that requires accurate soil health assessment. The health of the soil is characterized by parameters such as pH and nutrients like NPK. Soil testing is very important to predict crop yield and manage fertilizers and pesticides. Traditionally, soil testing methods were time and resource-intensive, often leading to delays. This discouraged farmers from conducting regular soil tests. We propose a machine learning-based application for rapid soil analysis. We have utilized 7000 soil images paired with their corresponding laboratory-tested results. These samples were used to train our CNN (Convolutional Neural Network) model to identify soil properties from images. The machine learning model ensures accuracy and robustness as it has been trained under various lighting conditions. Preliminary evaluations indicate an average prediction variance of 0.02 pH units, 1.5 kg/ha for N, 0.8 kg/ha for P, and 1.2kg/ha for K measured as the Mean Squared Error normalized by the range of actual nutrient values. This innovation aims to contribute to sustainable agricultural practices by making soil testing in real-time possible without the need for any extra equipment or expertise.
Keywords:
Feature extraction, Image processing, Pattern recognition, Remote sensing, Segmentation.
References:
[1] Nafiseh Kakhani et al., “Uncertainty Quantification of Soil Organic Carbon Estimation from Remote Sensing Data with Conformal Prediction,” Remote Sensing, vol. 16, no. 3, pp. 1-22, 2024.
[CrossRef] [Google Scholar] [Publisher Link]
[2] Hak-Jin Kim, Kenneth A. Sudduth, and John W. Hummel, “Soil Macronutrient Sensing for Precision Agriculture,” Journal of Environmental Monitoring, vol. 11, no. 10, pp. 1810-1824, 2009.
[CrossRef] [Google Scholar] [Publisher Link]
[3] Belete Biazen Bezabeh, and Abrham Debasu Mengistu, “The Effects of Multiple Layers Feed-Forward Neural Network Transfer Function in Digital Based Ethiopian Soil Classification and Moisture Prediction,” International Journal of Electrical and Computer Engineering, vol. 10, no. 4, pp. 4073-4079, 2020.
[CrossRef] [Google Scholar] [Publisher Link]
[4] F.M. Riese, and S. Keller, “Soil Texture Classification with 1D Convolutional Neural Networks Based on Hyperspectral Data,” ISPRS Annals of the Photogrammetry, Remote Sensing and Spatial Information Sciences, pp. 615-621, 2019.
[CrossRef] [Google Scholar] [Publisher Link]
[5] Proceedings of the 2016 IEEE Region 10 Conference (TENCON), IEEE Xplore, pp. 1-136, 2016. [Online]. Available: https://ieeexplore.ieee.org/stamp/stamp.jsp?arnumber=7847945
[6] Joseph V. Sinfield, Daniel Fagerman, and Oliver Colic, “Evaluation of Sensing Technologies for on-the-go Detection of Macro-Nutrients in Cultivated Soils,” Computers and Electronics in Agriculture, vol. 70, no. 1, pp. 1-8, 2010.
[CrossRef] [Google Scholar] [Publisher Link]
[7] Shakuntala Laskar, and Subra Mukherjee, “Optical Sensing Methods for Assessment of Soil Macronutrients and other Properties for Application in Precision Agriculture: A Review,” ADBU Journal of Engineering Technology, vol. 4, no. 1, pp. 206-210, 2016.
[Google Scholar] [Publisher Link]
[8] W.S. Lee et al., “Sensing Technologies for Precision Specialty Crop Production,” Computers and Electronics in Agriculture, vol. 74, no. 1, pp. 2-33, 2010.
[CrossRef] [Google Scholar] [Publisher Link]
[9] Masayuki Yokota, Takuya Okada, and Ichirou Yamaguchi, “An Optical Sensor for Analysis of Soil Nutrients by Using LED Light Sources,” Measurement Science and Technology, vol. 18, no. 7, 2007.
[CrossRef] [Google Scholar] [Publisher Link]
[10] Deepa V. Ramane, Supriya S. Patil, and A.D. Shaligram, “Detection of NPK Nutrients of Soil Using Fiber Optic Sensor,” International Journal of Research in Advent Technology, pp. 66-70, 2015.
[Google Scholar]
[11] Jianhan Lin et al., “Electrochemical Sensors for Soil Nutrient Detection: Opportunity and Challenge,” First IFIP TC 12 International Conference on Computer and Computing Technologies in Agriculture, Wuyishan, China, pp. 1349-1353, 2008. [CrossRef] [Google Scholar] [Publisher Link]
[12] Jeosadaque J. Sene et al., “A Sensor for Monitoring the Volume of Nutrient in a Solid Substrate-Based Growth Media by Using Electrochemical Admittance Spectroscopy,” Sensors and Actuators B: Chemical, vol. 87, no. 2, pp. 268-273, 2002.
[CrossRef] [Google Scholar] [Publisher Link]
[13] John Joshua Federis Montañez, “Soil Parameter Detection of Soil Test Kit-Treated Soil Samples through Image Processing with Crop and Fertilizer Recommendation,” Indonesian Journal of Electrical Engineering and Computer Science, vol. 24, no. 1, pp. 90-98, 2021.
[CrossRef] [Google Scholar] [Publisher Link]
[14] Abraham George Smith et al., “Segmentation of Roots in Soil with U-Net,” Plant Methods, vol. 16, no. 13, pp. 1-15, 2020.
[CrossRef] [Google Scholar] [Publisher Link]
[15] P.R. Shubhashree, and C. Prasanna Kumar, “A Quantitative Analysis of an Advance Smart Soil Analyser with a Soil Tester: A Comprehensive Survey,” International Journal of Engineering Research & Technology, vol. 7, no. 7, pp. 32-35, 2018.
[Google Scholar] [Publisher Link]
[16] Wahidur Rahman et al., “Real-Time and Low-Cost IoT Based Farming Using Raspberry Pi,” Indonesian Journal of Electrical Engineering and Computer Science, vol. 17, no. 1, pp. 197-204, 2020.
[CrossRef] [Google Scholar] [Publisher Link]
[17] Fernandez S. George et al., “Smart Soil Monitoring and Water Conservation Using Irrigation on Technology,” Indonesian Journal of Electrical Engineering and Computer Science, vol. 19, no. 1, pp. 99-107, 2020.
[CrossRef] [Google Scholar] [Publisher Link]
[18] Loubna Rabhi et al., “Digital Agriculture Based on Big Data Analytics: A Focus on Predictive Irrigation for Smart Farming in Morocco,” Indonesian Journal of Electrical Engineering and Computer Science, vol. 24, no. 1, pp. 581-589, 2021.
[CrossRef] [Google Scholar] [Publisher Link]
[19] D.N. Varshitha, and Savita Choudhary, “An Artificial Intelligence Solution for Crop Recommendation,” Indonesian Journal of Electrical Engineering and Computer Science, vol. 25, no. 3, pp. 1688-1695, 2022.
[CrossRef] [Google Scholar] [Publisher Link]
[20] D.N. Varshitha, and Savita Choudhary, “A Bootstrap Aggregation Approach for Adequate Crop Fertilizer and Nutrition Recommendation,” Indonesian Journal of Electrical Engineering and Computer Science, vol. 26, no. 3, pp. 1773-1780, 2022.
[CrossRef] [Google Scholar] [Publisher Link]
[21] Iniyan Shanmugam et al., “Prediction on Field Crops Yield Based on Analysis of Deep Learning Model,” Indonesian Journal of Electrical Engineering and Computer Science, vol. 30, no. 1, pp. 518-527, 2023.
[CrossRef] [Google Scholar] [Publisher Link]
[22] Dhandapani Karthikeyan et al., “Soil pH Periodic Assortment with Smart Irrigation Using Aerial Triboelectric Nanogenerator,” Indonesian Journal of Electrical Engineering and Computer Science, vol. 30, no. 3, pp. 1348-1358, 2023.
[CrossRef] [Google Scholar] [Publisher Link]
[23] Azril Abdul Rahim et al., “Real-Time Soil Monitoring and Irrigation System for Taro Yam Cultivation,” Indonesian Journal of Electrical Engineering and Computer Science, vol. 32, no. 2, pp. 1042-1049, 2023.
[CrossRef] [Google Scholar] [Publisher Link]
[24] Jeremy Jared Rumiche-Cardenas et al., “Internet of Things Meteorological Station for Climate Monitoring and Crop Optimization in Carabayllo-Perú,” Indonesian Journal of Electrical Engineering and Computer Science, vol. 38, no. 2, pp. 755-766, 2025.
[CrossRef] [Google Scholar] [Publisher Link]
[25] Mojtaba Naeimi, Prasad Daggupati, and Asim Biswas, “Image-Based Soil Characterization: A Review on Smartphone Applications,” Computers and Electronics in Agriculture, vol. 227, no. 1, pp. 1-15, 2024.
[CrossRef] [Google Scholar] [Publisher Link]