A Novel Approach of Speech Stress Emotion Recognition Using Visualized Image of Metrices
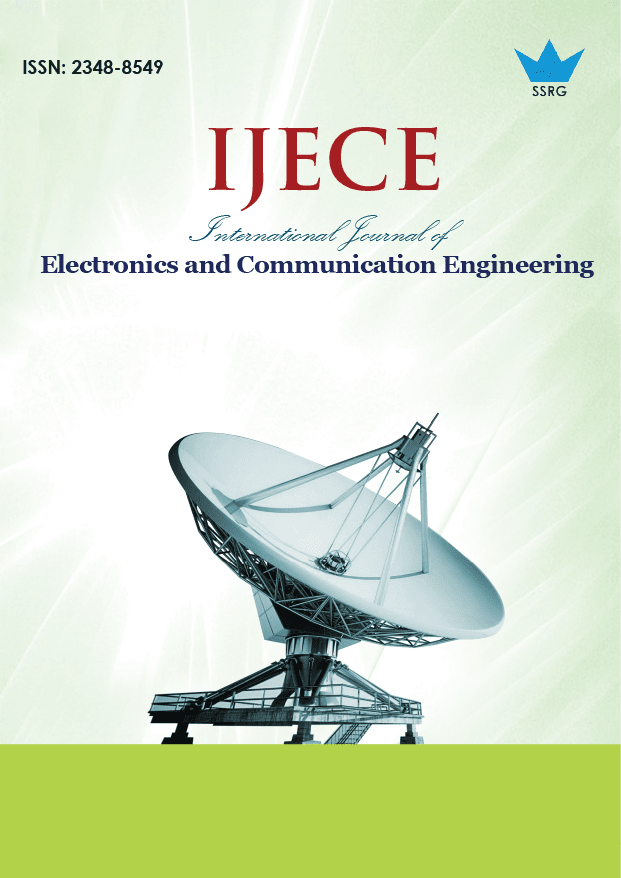
International Journal of Electronics and Communication Engineering |
© 2025 by SSRG - IJECE Journal |
Volume 12 Issue 4 |
Year of Publication : 2025 |
Authors : Vaijanath V. Yerigeri, Seema V. Yerigeri |
How to Cite?
Vaijanath V. Yerigeri, Seema V. Yerigeri, "A Novel Approach of Speech Stress Emotion Recognition Using Visualized Image of Metrices," SSRG International Journal of Electronics and Communication Engineering, vol. 12, no. 4, pp. 19-35, 2025. Crossref, https://doi.org/10.14445/23488549/IJECE-V12I4P103
Abstract:
Algopsychalia is inimical to the host. The present lifestyle of homo sapiens is stressful, due to which they suffer from psychogenic pain. Psychologists warn humans about algopsychalia’s destructive form, i.e. stress. Excessive stress can trigger suicidal tendencies in a person. Stress and emotions are highly co-related; therefore, the paper proposes efficient detection of stress-related emotions using speech to identify the level of stress and intimacy prior to the threat of suicidal ideations. The paper explores cepstral coefficient-based perceptual features like Mel Frequency, Inverted Mel frequency, Gammatone Wavelet, Gammatone Frequency, Perceptual Linear Predictive, Bark Frequency and Revised Perceptual Linear Prediction. Features are represented as an image and are input to the learning model. Representing features as an image and applying a Region-based Convolutional Neural Network (R-CNN) learning algorithm for evaluating auditory cues is the novelty of the proposed work. R-CNN learning reduces computational costs. The performance of a system is analyzed with the help of a benchmark dataset specific to stress, i.e., SUSAS. Comparative analysis is presented to demonstrate improvement in Speech Emotion Detection (SED) performance. The overall accuracy of 90.66% of stress-related emotions is achieved.
Keywords:
Speech Emotion Recognition (SER), Gammatone Wavelet Cepstral Coefficients (GWCC), Revised Perceptual Linear Prediction (RPLP), Bark Frequency Cepstral Coefficients (BFCC), Perceptual Linear Predictive coefficients (PLPC), Gammatone Frequency Cepstral Coefficients (GFCC).
References:
[1] Ernest Kramer, “Judgment of Personal Characteristics and Emotions from Nonverbal Properties of Speech,” Psychological Bulletin, vol. 60, no. 4, pp. 408-420, 1963.
[CrossRef] [Google Scholar] [Publisher Link]
[2] Ö. Özgür Bozkurt, and Z. Cihan Tayşı, “Audio-Based Gender and Age Identification,” 22nd Signal Processing and Communications Applications Conference, Trabzon, Turkey, pp. 1371-1374, 2014.
[CrossRef] [Google Scholar] [Publisher Link]
[3] Rafael A. Calvo, and Sidney D'Mello, “Affect Detection: An Interdisciplinary Review of Models, Methods, and Their Applications,” IEEE Transactions on Affective Computing, vol. 1, no. 1, pp. 18-37, 2010.
[CrossRef] [Google Scholar] [Publisher Link]
[4] Moataz El Ayadi, Mohamed S. Kamel, and Fakhri Karray, “Survey on Speech Emotion Recognition: Features, Classification Schemes, and Databases,” Pattern Recognition, vol. 44, no. 3, pp. 572-587, 2011.
[CrossRef] [Google Scholar] [Publisher Link]
[5] Jianhua Ma et al., “Ubiquitous Intelligence and Computing,” Third International Conference Proceedings, Lecture Notes in Computer Science, Wuhan, China, pp. 1-1190, 2006.
[CrossRef] [Google Scholar] [Publisher Link]
[6] Theodoros Kostoulas et al., “Affective Speech Interface in Serious Games for Supporting Therapy of Mental Disorders,” Expert Systems with Applications, vol. 39, no. 12, pp. 11072-11079, 2012.
[CrossRef] [Google Scholar] [Publisher Link]
[7] Karmele López-de-Ipiña et al., “On the Selection of Non-Invasive Methods based on Speech Analysis Oriented to Automatic Alz-heimer Disease Diagnosis,” Sensors, vol. 13, no. 5, pp. 6730-6745, 2013.
[CrossRef] [Google Scholar] [Publisher Link]
[8] Nicola Vanello et al., “Speech Analysis for Mood State Characterization in Bipolar Patients,” Annual International Conference of the IEEE Engineering in Medicine and Biology Society, San Diego, CA, USA, pp. 2104-2107, 2012.
[CrossRef] [Google Scholar] [Publisher Link]
[9] Gillinder Bedi et al., “A Window into the Intoxicated Mind? Speech as an Index of Psychoactive Drug Effects,” Neuropsychopharmacology, vol. 39, pp. 2340-2348, 2014.
[CrossRef] [Google Scholar] [Publisher Link]
[10] Gillinder Bedi et al., “Automated Analysis of Free Speech Predicts Psychosis Onset in High‐Risk Youths,” NPJ Schizophrenia, vol. 1, pp. 1-7, 2015.
[CrossRef] [Google Scholar] [Publisher Link]
[11] Zahi N. Karam et al., “Ecologically Valid Long‐Term Mood Monitoring of Individuals with Bipolar Disorder Using Speech,” 2014 IEEE International Conference on Acoustics, Speech and Signal Processing, Florence, Italy, pp. 4858-4862, 2014.
[CrossRef] [Google Scholar] [Publisher Link]
[12] Amir Muaremi et al., “Assessing Bipolar Episodes using Speech Cues Derived from Phone Calls,” Pervasive Computing Paradigms for Mental Health, pp. 103-114, 2014.
[CrossRef] [Google Scholar] [Publisher Link]
[13] Agnes Grünerbl et al., “Smartphone‐Based Recognition of States and State Changes in Bipolar Disorder Patients,” IEEE Journal of Biomedical and Health Informatics, vol. 19, no. 1, pp. 140-148, 2015.
[CrossRef] [Google Scholar] [Publisher Link]
[14] Venet Osmani, “Smartphones in Mental Health: Detecting Depressive and Manic Episodes,” IEEE Pervasive Computing, vol. 14, no. 3, pp. 10-13, 2015.
[CrossRef] [Google Scholar] [Publisher Link]
[15] James Z. Zhang et al., “Analysis of Stress in Speech Using Adaptive Empirical Mode Decomposition,” Conference Record of the Forty-Third Asilomar Conference on Signals, Systems and Computers, Pacific Grove, CA, USA, pp. 361-365, 2009.
[CrossRef] [Google Scholar] [Publisher Link]
[16] Harold Schlosberg, “Three Dimensions of Emotions,” Psychological Review, vol. 61, no. 2, pp. 81-88, 1954.
[CrossRef] [Google Scholar] [Publisher Link]
[17] Carl E. Williams, and Kenneth N. Stevens, “Emotions and Speech: Some Acoustic Correlates,” The Journal of the Acoustical Society of America, vol. 52, no. 4, pp. 1238-1250, 1972.
[CrossRef] [Google Scholar] [Publisher Link]
[18] Paul Ekman, “An Argument for Basic Emotions,” Cognition and Emotion, vol. 6, no. 3-4, pp. 169-200, 1992.
[CrossRef] [Google Scholar] [Publisher Link]
[19] Iain R. Murray, and John L. Arnott, “Toward the Simulation of Emotion in Synthetic Speech: A Review of the Literature on Human Vocal Emotion,” The Journal of the Acoustical Society of America, vol. 93, no. 2, pp. 1097-1108, 1993.
[CrossRef] [Google Scholar] [Publisher Link]
[20] Rainer Banse, and Klaus R. Scherer, “Acoustic Profiles in Vocal Emotion Expression,” Journal of Personality and Social Psychology, vol. 70, no. 3, pp. 614-636, 1996.
[CrossRef] [Google Scholar] [Publisher Link]
[21] R. Cowie et al., “Emotion Recognition in Human-Computer Interaction,” IEEE Signal Processing Magazine, vol. 18, no. 1, pp. 32- 80, 2001.
[CrossRef] [Google Scholar] [Publisher Link]
[22] Roddy Cowie, and Randolph R. Cornelius, “Describing the Emotional States that are Expressed in Speech,” Speech Communication, vol. 40, no. 1-2, pp. 5-32, 2003.
[CrossRef] [Google Scholar] [Publisher Link]
[23] Yuan Zong et al., “Double Sparse Learning Model for Speech Emotion Recognition,” Electronics Letters, vol. 52, no. 16, pp. 1410-1412, 2016.
[CrossRef] [Google Scholar] [Publisher Link]
[24] J.F. Gómez-Lopera et al., “The Evaluation Problem in Discrete Semi-Hidden Markov Models,” Mathematics and Computers in Simulation, vol. 137, pp. 350-365, 2017.
[CrossRef] [Google Scholar] [Publisher Link]
[25] Li Zhiyong et al., “Reject Inference in Credit Scoring Using Semi-Supervised Support Vector Machines,” Expert Systems with Applications, vol. 74, pp. 105-114, 2017.
[CrossRef] [Google Scholar] [Publisher Link]
[26] Jase D. Sitton, Yasha Zeinali, and Brett A. Story, “Rapid Soil Classification Using Artificial Neural Networks for Use in Constructing Compressed Earth Blocks,” Construction and Building Materials, vol. 138, pp. 214-221, 2017.
[CrossRef] [Google Scholar] [Publisher Link]
[27] Jesus Maillo et al., “kNN-IS - An Iterative Spark- Based Design of the K-Nearest Neighbors Classifier for Big Data,” Knowledge-Based Systems, vol. 117, pp. 3-15, 2017.
[CrossRef] [Google Scholar] [Publisher Link]
[28] Siddique Latif, Rajiv Rana, and Junaid Qadir, “Adversarial Machine Learning and Speech Emotion Recognition: Utilizing Generative Adversarial Networks for Robustness,” arXiv, pp. 1-7, 2018.
[CrossRef] [Google Scholar] [Publisher Link]
[29] Florian Eyben et al., “The Geneva Minimalistic Acoustic ParameterSet (GeMAPS) for Voice Research and Affective Computing,” Transactions on Affective Computing, vol. 7, no. 2, pp. 190-202, 2016.
[CrossRef] [Google Scholar] [Publisher Link]
[30] C.K. Yogesh et al., “A New Hybrid PSO Assisted Biogeography-Based Optimization for Emotion and Stress Recognition from Speech Signal,” Expert Systems with Applications, vol. 69, pp. 149-158, 2017.
[CrossRef] [Google Scholar] [Publisher Link]
[31] C.K. Yogesh et al., “Hybrid BBO_PSO and Higher Order Spectral Features for Emotion and Stress Recognition from Natural Speech,” Applied Soft Computing, vol. 56, pp. 217-232, 2017.
[CrossRef] [Google Scholar] [Publisher Link]
[32] Yue Zhang et al., “Multi-Task Deep Neural Network with Shared Hidden Layers: Breaking Down the Wall between Emotion Representations,” IEEE International Conference on Acoustics, Speech and Signal Processing, New Orleans, LA, USA, pp. 4990-4994, 2017.
[CrossRef] [Google Scholar] [Publisher Link]
[33] Zhong-Qiu Wang, and Ivan Tashev, “Learning Utterance-Level Representations for Speech Emotion and Age/Gender Recognition Using Deep Neural Networks,” IEEE International Conference on Acoustics, Speech and Signal Processing, New Orleans, LA, USA, pp. 5150-5154, 2017.
[CrossRef] [Google Scholar] [Publisher Link]
[34] Siddique Latif et al., “Transfer Learning for Improving Speech Emotion Classification Accuracy,” arXiv, pp. 1-5, 2020.
[CrossRef] [Google Scholar] [Publisher Link]
[35] Arianna Mencattini et al., “Continuous Estimation of Emotions in Speech by Dynamic Cooperative Speaker Models,” IEEE Transactions on Affective Computing, vol. 8, no. 3, pp. 314-327, 2017.
[CrossRef] [Google Scholar] [Publisher Link]
[36] Peng Song, “Transfer Linear Subspace Learning for Cross-corpus Speech Emotion Recognition,” IEEE Transactions on Affective Computing, vol. 10, no. 2, pp. 265-275, 2017.
[CrossRef] [Google Scholar] [Publisher Link]
[37] George M. Slavich, Sara Taylor, and Rosalind W. Picard, “Stress Measurement Using Speech: Recent Advancements, Validation Issues, and Ethical and Privacy Considerations,” The International Journal on the Biology of Stress, vol. 22, no. 4, pp. 408-413, 2019.
[CrossRef] [Google Scholar] [Publisher Link]
[38] Charles R. Marmar et al., “Speech‐Based Markers for Posttraumatic Stress Disorder in US Veterans,” Depression and Anxiety, 2019.
[CrossRef] [Google Scholar] [Publisher Link]
[39] Nurul Lubis et al., “Positive Emotion Elicitation in Chat-Based Dialogue Systems,” IEEE/ACM Transactions on Audio, Speech, and Language Processing, vol. 27, no. 4, pp. 866-877, 2019.
[CrossRef] [Google Scholar] [Publisher Link]
[40] Reza Lotfian, and Carlos Busso, “Curriculum Learning for Speech Emotion Recognition from Crowdsourced Labels,” IEEE/ACM Transactions on Audio, Speech, And Language Processing, vol. 27, no. 4, pp. 815-826, 2019.
[CrossRef] [Google Scholar] [Publisher Link]
[41] Leila Kerkeni et al., Automatic Speech Emotion Recognition Using Machine Learning, Intech Open, 2019.
[CrossRef] [Google Scholar] [Publisher Link]
[42] Ismail Shahin, Ali Bou Nassif, and Shibani Hamsa, “Emotion Recognition Using Hybrid Gaussian Mixture Model and Deep Neural Network,” IEEE Access, vol. 7, pp. 26777-26787, 2019.
[CrossRef] [Google Scholar] [Publisher Link]
[43] Zhaocheng Huang, and Julien Epps, “An Investigation of Partition-Based and Phonetically Aware Acoustic Features for Continuous Emotion Prediction from Speech,” IEEE Transactions on Affective Computing, vol. 11, no. 4, pp. 653-668, 2020.
[CrossRef] [Google Scholar] [Publisher Link]
[44] Siddique Latif et al., “Mobile Health in the Developing World: Review of Literature and Lessons from a Case Study,” IEEE Access, vol. 5, pp. 11540-1156, 2017.
[CrossRef] [Google Scholar] [Publisher Link]
[45] Jun Deng et al., “Exploitation of Phase- Based Features for Whispered Speech Emotion Recognition,” IEEE Access, vol. 4, pp. 4299-4309, 2016.
[CrossRef] [Google Scholar] [Publisher Link]
[46] Suman Deb, and Samarendra Dandapat, “Emotion Classification Using Segmentation of Vowel-Like and Non- Vowel-Like Regions,” IEEE Transactions on Affective Computing, vol. 10, no. 3, pp. 360-373, 2019.
[CrossRef] [Google Scholar] [Publisher Link]
[47] Yehav Alkaher, Osher Dahan, and Yair Moshe, “Detection of Distress in Speech,” IEEE International Conference on the Science of Electrical Engineering, Eilat, Israel, pp. 1-5, 2016.
[CrossRef] [Google Scholar] [Publisher Link]
[48] Mohinish Shukla, Katherine S. White, and Richard N. Aslin, “Prosody Guides the Rapid Mapping of Auditory Word Forms Onto Visual Objects in 6-Month-Old Infants,” The Proceedings of the National Academy of Sciences, vol. 108, no. 15, pp. 6038-6043, 2016.
[CrossRef] [Google Scholar] [Publisher Link]
[49] Kunxia Wang et al., “Speech Emotion Recognition Using Fourier Parameters,” IEEE Transactions on Affective Computing, vol. 6, no. 1, pp. 69-75, 2015.
[CrossRef] [Google Scholar] [Publisher Link]
[50] S. Lalitha et al., “Time-Frequency and Phase Derived Features for Emotion Classification,” Annual IEEE India Conference, New Delhi, India, pp. 1-5, 2015.
[CrossRef] [Google Scholar] [Publisher Link]
[51] S. Lalitha et al., “Emotion Detection Using MFCC and Cepstrum Features,” Procedia Computer Science, vol. 70, pp. 29-35, 2015.
[CrossRef] [Google Scholar] [Publisher Link]
[52] Jesús B. Alonso et al., “New Approach in Quantification of Emotional Intensity from the Speech Signal: Emotional Temperature,” Expert Systems with Applications, vol. 42, no. 24, pp. 9554-9564, 2015.
[CrossRef] [Google Scholar] [Publisher Link]
[53] Anila M. D'Mello, Peter E. Turkeltaub, and Catherine J. Stoodley, “Cerebellar tDCS Modulates Neural Circuits during Semantic Prediction: A Combined tDCS-fMRI Study,” Journal of Neuroscience, vol. 37, no. 6, pp. 1604-1613, 2015.
[CrossRef] [Google Scholar] [Publisher Link]
[54] Ismail Shahin, and Mohammed Nasser Ba-Hutair, “Talking Condition Recognition in Stressful and Emotional Talking Environments Based on CSPHMM2s,” International Journal of Speech Technology, vol. 18, pp. 77-90, 2015.
[CrossRef] [Google Scholar] [Publisher Link]
[55] Hariharan Muthusamy, Kemal Polat, and Sazali Yaacob, “Improved Emotion Recognition Using Gaussian Mixture Model and Extreme Learning Machine in Speech and Glottal Signals,” Mathematical Problems in Engineering, vol. 2015, no. 1, pp. 1-13, 2015.
[CrossRef] [Google Scholar] [Publisher Link]
[56] Houwei Cao, Ragini Verma, and Ani Nenkova, “Speaker-Sensitive Emotion Recognition via Ranking: Studies on Acted and Spontaneous Speech,” Computer Speech & Language, vol. 29, pp. 186-202, 2015.
[CrossRef] [Google Scholar] [Publisher Link]
[57] Suman Deb, and Samarendra Dandapat, “A Novel Breathiness Feature for Analysis and Classification of Speech under Stress,” Twenty First National Conference on Communications, Mumbai, India, pp. 1-5, 2015.
[CrossRef] [Google Scholar] [Publisher Link]
[58] Patricia Henríquez et al., “Nonlinear Dynamics Characterization of Emotional Speech,” Neurocomputing, vol. 132, pp. 126-135, 2014.
[CrossRef] [Google Scholar] [Publisher Link]
[59] Qirong Mao et al., “Learning Salient Features for Speech Emotion Recognition Using Convolutional Neural Networks,” IEEE Transactions on Multimedia, vol. 16, no. 8, pp. 2203-2213, 2014.
[CrossRef] [Google Scholar] [Publisher Link]
[60] Dorota Kamińska, Tomasz Sapiński, and Adam Pelikant, “Comparison of Perceptual Features Efficiency for Automatic Identification of Emotional States from Speech,” 6th International Conference on Human System Interactions, Sopot, Poland, pp. 210-213, 2013.
[CrossRef] [Google Scholar] [Publisher Link]
[61] Silvia Monica Feraru, Dagmar Schuller, and Bj÷rn Schuller, “Cross-Language Acoustic Emotion Recognition: An Overview and Some Tendencies,” International Conference on Affective Computing and Intelligent Interaction, Xi'an, China, pp. 125-131, 2015.
[CrossRef] [Google Scholar] [Publisher Link]
[62] Iker Luengo, Eva Navas, and Inmaculada Hernáez, “Feature Analysis and Evaluation for Automatic Emotion Identification in Speech,” IEEE Transactions on Multimedia, vol. 12, no. 6, pp. 490-501, 2010.
[CrossRef] [Google Scholar] [Publisher Link]
[63] André Stuhlsatz et al., “Deep Neural Networks for Acoustic Emotion Recognition: Raising the Benchmarks,” IEEE International Conference on Acoustics, Speech and Signal Processing, Prague, Czech Republic, pp. 5688-5691, 2011.
[CrossRef] [Google Scholar] [Publisher Link]
[64] Wang Yutai et al., “Speaker Recognition Based on Dynamic MFCC Parameters,” International Conference on Image Analysis and Signal Processing, Linhai, China, pp. 406-409, 2008.
[CrossRef] [Google Scholar] [Publisher Link]
[65] Nan Ding et al., “Speech Emotion Features Selection Based on BBO-SVM,” Tenth International Conference on Advanced Computational Intelligence, Xiamen, China, pp. 210-216, 2018.
[CrossRef] [Google Scholar] [Publisher Link]
[66] Yaxin Sun, Guihua Wen, and Jiabing Wang, “Weighted Spectral Features Based on Local Hu Moments for Speech Emotion Recognition,” Biomedical Signal Processing and Control, vol. 18, pp. 80-90, 2015.
[CrossRef] [Google Scholar] [Publisher Link]
[67] Maxim Sidorov et al., “Speech-Based Emotion Recognition: Feature Selection by Self-Adaptive Multi-Criteria Genetic Algorithm,” International Conference on Language Resources and Evaluation, Reykjavik, Iceland, pp. 3481-3485, 2014.
[Google Scholar] [Publisher Link]
[68] Anton Batliner et al., “Combining Efforts for Improving Automatic Classification of Emotional User States,” Procedding 5th Slovenian 1st International Language Technology Conference, pp. 240-245, 2006.
[Google Scholar] [Publisher Link]
[69] Xinzhou Xu et al., “Connecting Subspace Learning and Extreme Learning Machine in Speech Emotion Recognition,” IEEE Transactions on Multimedia, vol. 21, no. 3, pp. 795-808, 2019.
[CrossRef] [Google Scholar] [Publisher Link]
[70] B. Yang, and M. Lugger, “Emotion Recognition from Speech Signals using New Harmony Features,” Signal Processing, vol. 90, no. 5, pp. 1415-1423, 2010.
[CrossRef] [Google Scholar] [Publisher Link]
[71] Anuja Bombatkar et al., “Emotion Recognition Using Speech Processing Using K-Nearest Neighbor Algorithm,” International Journal of Engineering Research and Applications, pp. 68-71, 2014.
[Google Scholar] [Publisher Link]
[72] Md. Kamruzzaman Sarker, Kazi Md. Rokibul Alam, and Md. Arifuzzaman, “Emotion Recognition from Speech based on Relevant Feature and Majority Voting,” International Conference on Informatics, Electronics & Vision, Dhaka, Bangladesh, pp. 1-5, 2014.
[CrossRef] [Google Scholar] [Publisher Link]
[73] Shulan Xia et al., “An Improved Algorithm of Speech Emotion Recognition,” International Journal of u- and e- Service, Science and Technology, vol. 8, no. 12, pp. 217-226, 2015.
[CrossRef] [Google Scholar] [Publisher Link]
[74] Andrzej Majkowski et al., “Classification of Emotions from Speech Signal,” Signal Processing: Algorithms, Architectures, Arrangements, and Applications, Poznan, Poland, pp. 276-281, 2016.
[CrossRef] [Google Scholar] [Publisher Link]
[75] Yan Wang, and Weiping Hu, “Speech Emotion Recognition Based on Improved MFCC,” Proceedings of the 2nd International Conference on Computer Science and Application Engineering, Hohhot China, pp. 1-7, 2018.
[CrossRef] [Google Scholar] [Publisher Link]
[76] Roy Patterson et al., “An Efficient Auditory Filterbank Based on the Gammatone Function,” Speech-Group Meeting of the Institute of Acoustics on Auditory Modelling, pp. 1-33, 1987.
[Google Scholar]
[77] X. Yang, K. Wang, and S.A. Shamma, “Auditory Representations of Acoustic Signals,” IEEE Transactions on Information Theory, vol. 38, no. 2, pp. 824 -839, 1992.
[CrossRef] [Google Scholar] [Publisher Link]
[78] Malcolm Slaney, “An Efficient Implementation of the Patterson-Holdsworth Auditory Filterbank,” Apple Computer Technical Report, no. 35, 1993.
[Google Scholar]
[79] Ludger Solbach, Rolf Wöhrmann, and Jörg Kliewer, The Complex-Valued Continuous Wavelet Transforms as a Preprocessor for Auditory Scene Analysis, 1st ed., Computational Auditory Scene Analysis, CRC Press, pp. 273-292, 1998.
[Google Scholar] [Publisher Link]
[80] Stéphane G. Mallat, A Wavelet Tour of Signal Processing, Academic Press, pp. 1-577, 1998.
[Google Scholar] [Publisher Link]
[81] Arun Venkitaraman, Aniruddha Adiga, and Chandra Sekhar Seelamantula, “Auditory Motivated Gammatone Wavelet Transform,” Signal Processing, vol. 94, pp. 608-619, 2014.
[CrossRef] [Google Scholar] [Publisher Link]
[82] Gerald R. Patterson, John B. Reid, and Thomas J. Dishion, Antisocial Boys, Castalia Publishing Company, pp. 1-193, 1992.
[Google Scholar] [Publisher Link]
[83] Erika Hoff-Ginsberg et al., “Maternal Speech and the Child's Development of Syntax: A Further Look,” Journal of Child Language, vol. 17, no. 1, pp. 85-99, 1990.
[CrossRef] [Google Scholar] [Publisher Link]
[84] W. Hess, Pitch Determination of Speech Signals: Algorithms and Devices, Springer Science & Business Media, pp. 1-700, 2012.
[Google Scholar] [Publisher Link]
[85] L. Rabiner et al., “A Comparative Performance Study of Several Pitch Detection Algorithms,” IEEE Transactions on Acoustics, Speech, and Signal Processing, vol. 24, no. 5, pp. 399-418, 1976.
[CrossRef] [Google Scholar] [Publisher Link]
[86] Sira Gonzalez, and Mike Brookes, “A Pitch Estimation Filter Robust to High Levels of Noise (PEFAC),” 19th European Signal Processing Conference, Barcelona, Spain, pp. 451-455, 2011.
[Google Scholar] [Publisher Link]
[87] Denis Byrne et al., “An International Comparison of Long-Term Average Speech Spectra,” The Journal of the Acoustical Society of America, vol. 96, pp. 2108-2120, 1994.
[CrossRef] [Google Scholar] [Publisher Link]
[88] Mike Brookes, VOICEBOX: A Speech Processing Toolbox for MATLAB, 1997.
[Google Scholar] [Publisher Link]
[89] Ross Girshick et al., “Rich Feature Hierarchies for Accurate Object Detection and Semantic Segmentation,” IEEE Conference on Computer Vision and Pattern Recognition, Columbus, OH, USA, pp. 580-587, 2014.
[CrossRef] [Google Scholar] [Publisher Link]
[90] Swati Pahune, and Nilu Mishra, “Emotion Recognition through Combination of Speech and Image Processing,” International Journal on Recent and Innovation Trends in Computing and Communication, vol. 3, no. 2, pp. 134-137, 2015.
[Google Scholar] [Publisher Link]
[91] Vishal B. Waghmare et al., “Development of Isolated Marathi Words Emotional Speech Database,” International Journal of Computer Applications, vol. 94, no. 4, pp. 19-22, 2014.
[CrossRef] [Google Scholar] [Publisher Link]
[92] Vishal B. Waghmare et al., “Emotion Recognition System from Artificial Marathi Speech Using MFCC and LDA Techniques,” Fifth International Conference on Advances in Communication, Network, and Computing, pp. 1-9, 2014.
[Google Scholar]
[93] Wei Jiang et al., “Speech Emotion Recognition with Heterogeneous Feature Unification of Deep Neural Network,” Sensors, vol. 19, no. 12, pp. 1-15, 2019.
[CrossRef] [Google Scholar] [Publisher Link]
[94] Peng Song et al., “Transfer Linear Subspace Learning for Cross-Corpus Speech Emotion Recognition,” IEEE Transactions on Affective Computing, vol. 10, no. 2, pp. 265-275, 2019.
[CrossRef] [Google Scholar] [Publisher Link]
[95] YeSim Ülgen Sonmez, and Asaf Varol, “New Trends in Speech Emotion Recognition,” 7th International Symposium on Digital Forensics and Security, Barcelos, Portugal, pp. 1-7, 2019.
[CrossRef] [Google Scholar] [Publisher Link]
[96] Jianyou Wang et al., “Speech Emotion Recognition with Dual-Sequence LSTM Architecture,” ICASSP 2020 - 2020 IEEE International Conference on Acoustics, Speech and Signal Processing, Barcelona, Spain, pp. 6474-6478, 2020.
[CrossRef] [Google Scholar] [Publisher Link]
[97] Noushin Hajarolasvadi, and Hasan Demirel, “3D CNN-Based Speech Emotion Recognition Using K- Means Clustering and Spectrograms,” Entropy, vol. 21, no. 5, pp. 1-17, 2019.
[CrossRef] [Google Scholar] [Publisher Link]
[98] Abdul Malik Badshah et al., “Deep Features-Based Speech Emotion Recognition for Smart Affective Services,” Multimedia Tools and Applications, vol. 78, pp. 5571-5589, 2019.
[CrossRef] [Google Scholar] [Publisher Link]
[99] Wootaek Lim, Daeyoung Jang, and Taejin Lee, “Speech Emotion Recognition using Convolutional and Recurrent Neural Networks,” Asia-Pacific Signal and Information Processing Association Annual Summit and Conference, Jeju, Korea (South), pp. 1-4, 2016.
[CrossRef] [Google Scholar] [Publisher Link]
[100] Zhengwei Huang et al., “Speech Emotion Recognition Using CNN,” Proceedings of the 22nd ACM International Conference on Multimedia, Orlando, Florida, USA, pp. 801-804, 2014.
[CrossRef] [Google Scholar] [Publisher Link]
[101] Ping Zhou et al., “Speech Emotion Recognition Based on Mixed MFCC,” Applied Mechanics and Materials, vol. 249-250, pp. 1252-1258, 2013.
[CrossRef] [Google Scholar] [Publisher Link]
[102] Dalibor Mitrović, Matthias Zeppelzauer, and Christian Breiteneder, “Features for Content-Based Audio Retrieval,” Advances in Computers, vol. 78, pp. 71-150, 2010.
[CrossRef] [Google Scholar] [Publisher Link]