Performance Analysis of Machine Learning Algorithms for Non-Alcoholic Fatty Liver Disease Prediction and Classification
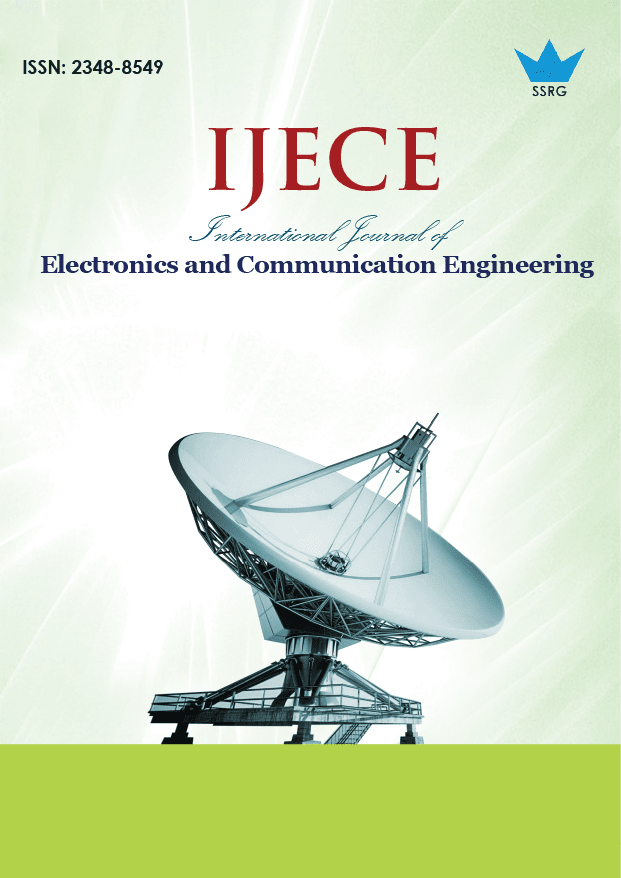
International Journal of Electronics and Communication Engineering |
© 2025 by SSRG - IJECE Journal |
Volume 12 Issue 4 |
Year of Publication : 2025 |
Authors : Pyla Jyothi, P. Ajitha |
How to Cite?
Pyla Jyothi, P. Ajitha, "Performance Analysis of Machine Learning Algorithms for Non-Alcoholic Fatty Liver Disease Prediction and Classification," SSRG International Journal of Electronics and Communication Engineering, vol. 12, no. 4, pp. 49-61, 2025. Crossref, https://doi.org/10.14445/23488549/IJECE-V12I4P105
Abstract:
Non-alcoholic disease detection is one of the leading research works in recent days. Modern life has changed the food and environmental culture, making them overweight, stressed, unhealthy conditions always and which causes various diseases due to overweight and diabetes. Commonly, an alcoholic addict can be affected by Fatty Liver Diseases (FLD), whereas identifying fatty liver diseases for a non-alcoholic person is a challenging task. It is not so easy even suspecting that a patient has FLD at the earlier stage of the symptoms since the symptoms of FLD are very similar to other diseases, and it may lead to wrong diagnosis and treatment. The severity level of 30% of FLD patients is increased suddenly and leads to heart attack, stroke, and death. Thus, based on the symptoms of weight loss, abdominal pain, and fatigue, it is essential to diagnose NAFLD, which can be identified accurately from pathological and genomic data using efficient learning methods to immediately provide the right and better treatment. This paper implements multiple machine learning algorithms for analyzing the pathological information obtained from the NAFLD and NASH DNA datasets and finding the best model concerning the performance. This paper uses 3-fold cross verification with recursive feature elimination methods to improve the original accuracy of the prediction. From the comparison, the SVM model obtained 87% accuracy, which is better than the KNN and RF models. The experimental results with the performance comparison are explained in detail in the paper.
Keywords:
Non-Alcoholic Fatty Liver Disease (NAFLD), Overweight, Diabetes, Fatty Liver Diseases (FLD), Pathological information, NASH DNA datasets.
References:
[1] Liver - Fatty Liver Disease, Better Health Channel. [Online]. Avaliable:
https://www.betterhealth.vic.gov.au/health/conditionsandtreatments/liver-fatty-liver-disease/
[2] Souveek Mitra, Arka De, and Abhijit Chowdhury, “Epidemiology of Non-Alcoholic and Alcoholic Fatty Liver Diseases,” Translational Gastroenterology and Hepatology, vol. 5, no. 16, pp. 1-17, 2020.
[CrossRef] [Google Scholar] [Publisher Link]
[3] Ana Carolina Cardoso, Claudio de Figueiredo-Mendes, and Cristiane A. Villela-Nogueira, “Current Management of NAFLD/NASH,” Liver International, vol. 41, pp. 89-94, 2021.
[CrossRef] [Google Scholar] [Publisher Link]
[4] Leen J.M. Heyens et al., “Liver Fibrosis in Non-Alcoholic Fatty Liver Disease: From Liver Biopsy to Non-Invasive Biomarkers in Diagnosis and Treatment,” Frontiers in Medicine, vol. 8, pp. 1-20, 2021.
[CrossRef] [Google Scholar] [Publisher Link]
[5] Joseph C. Ahn et al., “Application of Artificial Intelligence for the Diagnosis and Treatment of Liver Diseases,” Hepatology, vol. 73, no. 6, pp. 2546-2563, 2021.
[CrossRef] [Google Scholar] [Publisher Link]
[6] Yogesh Kumar et al., “Artificial Intelligence in Disease Diagnosis: A Systematic Literature Review, Synthesizing Framework and Future Research Agenda,” Journal of Ambient Intelligence and Humanized Computing, vol. 14, pp. 8459-8486, 2022.
[CrossRef] [Google Scholar] [Publisher Link]
[7] Taher M. Ghazal et al., “Intelligent Model to Predict Early Liver Disease using Machine Learning Technique,” International Conference on Business Analytics for Technology and Security, Dubai, United Arab Emirates, pp. 1-5, 2022.
[CrossRef] [Google Scholar] [Publisher Link]
[8] Shuxuan Xie, Zengchen Yu, and Zhihan Lv, “Multi-Disease Prediction Based on Deep Learning: A Survey,” Computer Modeling in Engineering & Sciences, vol. 128, no. 2, pp. 489-522, 2021.
[CrossRef] [Google Scholar] [Publisher Link]
[9] Optimization Algorithms - Narrow AI Glossary, Complexica. [Online]. Avaliable: https://www.complexica.com/narrow-ai-glossary/optimization-algorithms/
[10] Grace Lai-Hung Wong et al., “Artificial Intelligence in Prediction of Non‐Alcoholic Fatty Liver Disease and Fibrosis,” Journal of Gastroenterology and Hepatology, vol. 36, no. 3, pp. 543-550, 2021.
[CrossRef] [Google Scholar] [Publisher Link]
[11] Weidong Ji et al., “A Machine Learning Based Framework to Identify and Classify Non-alcoholic Fatty Liver Disease in a Large-Scale Population,” Frontiers in Public Health, vol. 10, pp. 1-10, 2022.
[CrossRef] [Google Scholar] [Publisher Link]
[12] Yang-Yuan Chen et al., “Machine-Learning Algorithm for Predicting Fatty Liver Disease in a Taiwanese Population,” Journal of Personalized Medicine, vol. 12, no. 7, pp. 1-10, 2022.
[CrossRef] [Google Scholar] [Publisher Link]
[13] Yuan-Xing Liu et al., “Comparison and Development of Advanced Machine Learning Tools to Predict Non-Alcoholic Fatty Liver Disease: An Extended Study,” Hepatobiliary & Pancreatic Diseases International, vol. 20, no. 5, pp. 409-415, 2021. [CrossRef] [Google Scholar] [Publisher Link]
[14] Miguel Suárez et al., “A Machine Learning-Based Method for Detecting Liver Fibrosis,” Diagnostics, vol. 13, no. 18, pp. 1-14, 2023.
[CrossRef] [Google Scholar] [Publisher Link]
[15] Sina Ghandian et al., “Machine Learning to Predict the Progression of Non‐Alcoholic Fatty Liver to Non‐Alcoholic Steatohepatitis or Fibrosis,” JGH Open, vol. 6, no. 3, pp. 196-204, 2022.
[CrossRef] [Google Scholar] [Publisher Link]
[16] Shenghua Qin et al., “Machine Learning Classifiers for Screening Non-Alcoholic Fatty Liver Disease in General Adults,” Scientific Reports, vol. 13, pp. 1-7, 2023.
[CrossRef] [Google Scholar] [Publisher Link]
[17] Muhamamd Haseeb Aslam, Syed Fawad Hussain, and Raja Hashim Ali, “Predictive Analysis on Severity of Non-Alcoholic Fatty Liver Disease (NAFLD) Using Machine Learning Algorithms,” 17th International Conference on Emerging Technologies, Swabi, Pakistan, pp. 95-100, 2022.
[CrossRef] [Google Scholar] [Publisher Link]
[18] Liang Zhang et al., “Development of Cost-Effective Fatty Liver Disease Prediction Models in a Chinese Population: Statistical and Machine Learning Approaches,” JMIR Formative Research, vol. 8, pp. 1-19, 2024.
[CrossRef] [Google Scholar] [Publisher Link]
[19] Amir Reza Naderi Yaghouti, Hamed Zamanian, and Ahmad Shalbaf, “Machine Learning Approaches for Early Detection of Non-Alcoholic Steatohepatitis Based on Clinical and Blood Parameters,” Scientific Reports, vol. 14, pp. 1-12, 2024.
[CrossRef] [Google Scholar] [Publisher Link]
[20] Aylin Tahmasebi et al., “Ultrasound‐Based Machine Learning Approach for Detection of Nonalcoholic Fatty Liver Disease,” Journal of Ultrasound in Medicine, vol. 42, no. 8, pp. 1747-1756, 2023.
[CrossRef] [Google Scholar] [Publisher Link]
[21] M.K. Praveen Kumar et al., “Enhancing Kyphosis Disease Prediction: Evaluating Machine Learning Algorithms Effectiveness,” International Conference on Expert Clouds and Applications, Bengaluru, India, pp. 938-943, 2024.
[CrossRef] [Google Scholar] [Publisher Link]