Development of a Smart Energy Management System Using Machine Learning and Solar panels
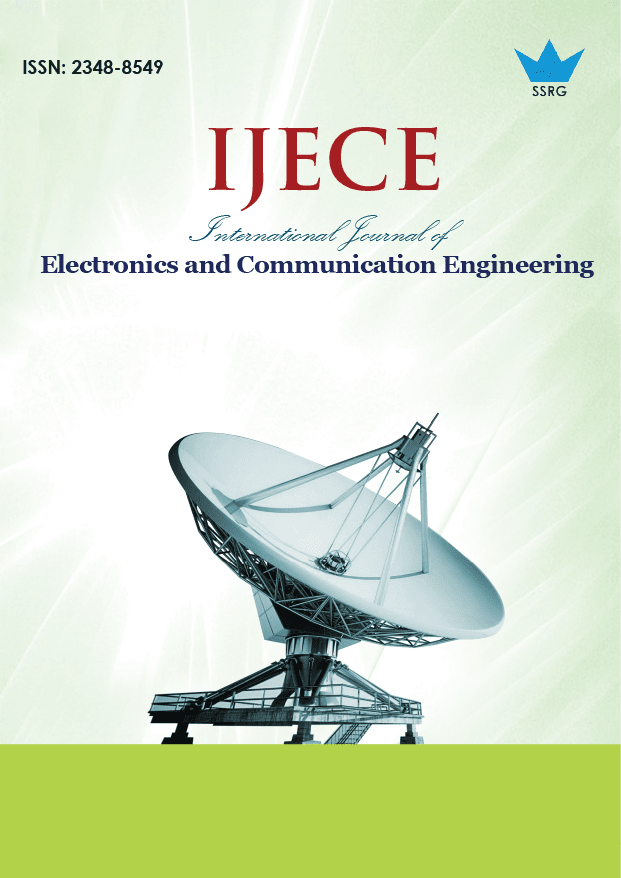
International Journal of Electronics and Communication Engineering |
© 2025 by SSRG - IJECE Journal |
Volume 12 Issue 4 |
Year of Publication : 2025 |
Authors : German Alberto Echaiz Espinoza, Miguel Angel Sanchez Valencia, Carlos Benjamin Huillca Velásquez |
How to Cite?
German Alberto Echaiz Espinoza, Miguel Angel Sanchez Valencia, Carlos Benjamin Huillca Velásquez, "Development of a Smart Energy Management System Using Machine Learning and Solar panels," SSRG International Journal of Electronics and Communication Engineering, vol. 12, no. 4, pp. 110-118, 2025. Crossref, https://doi.org/10.14445/23488549/IJECE-V12I4P110
Abstract:
Households should use good and efficient energy management practices to minimize energy consumption and maximize the use of renewable energy sources. This paper describes a novel approach to regulating household energy consumption that combines solar panels and machine learning approaches. The suggested system estimates energy demand and solar power generation with high accuracy using past data on energy consumption and weather forecasts. The machine learning model dynamically adjusts energy usage patterns and storage solutions, thereby maximizing solar energy utilization and minimizing grid dependency. In addition, the local grid tariff cost is compared to determine the time required in which the implemented system becomes self-sufficient. Simulation results demonstrate significant improvements in energy efficiency and cost savings for residential users.
Keywords:
Home Energy Management, Machine learning, Energy efficiency, Solar panels.
References:
[1] Kumar Siddhant et al., “Solar Energy Forecasting Using Artificial Neural Network,” 2022 IEEE Students Conference on Engineering and Systems, Prayagraj, India, pp. 1-5, 2022.
[CrossRef] [Google Scholar] [Publisher Link]
[2] Prashant Anand et al., “Occupancy-Based Energy Consumption Modelling Using Machine Learning Algorithms for Institutional Buildings,” Energy and Buildings, vol. 252, 2021.
[CrossRef] [Google Scholar] [Publisher Link]
[3] Masood Rizvi, Bhanu Pratap, and Shashi Bhushan Singh, “Optimal Energy Management in a Microgrid under Uncertainties Using Novel Hybrid Metaheuristic Algorithm,” Sustainable Computing: Informatics and Systems, vol. 36, 2022.
[CrossRef] [Google Scholar] [Publisher Link]
[4] Yi Wang et al., “Review of Smart Meter Data Analytics: Applications, Methodologies, and Challenges,” IEEE Transactions on Smart Grid, vol. 10, no. 3, pp. 3125-3148, 2019.
[CrossRef] [Google Scholar] [Publisher Link]
[5] Marc Beaudin, and Hamidreza Zareipour, “Home Energy Management Systems: A Review of Modelling and Complexity,” Renewable and Sustainable Energy Reviews, vol. 45, pp. 318-335, 2015.
[CrossRef] [Google Scholar] [Publisher Link]
[6] Chibuike Peter Ohanu, Salihu Ahmed Rufai, and Ugbe Christiana Oluchi, “A Comprehensive Review of Recent Developments in Smart Grid through Renewable Energy Resources Integration,” Heliyon, vol. 10, no. 3, pp. 1-17, 2024.
[CrossRef] [Google Scholar] [Publisher Link]
[7] Muhammad Fahad Zia, Elhoussin Elbouchikhi, and Mohamed Benbouzid, “Microgrids Energy Management Systems: A Critical Review on Methods, Solutions, and Prospects,” Applied Energy, vol. 222, pp. 133-1055, 2018.
[CrossRef] [Google Scholar] [Publisher Link]
[8] Yingchun He, Jun Liu, and Tian Zhang, “Home Energy Management System Optimization Strategy Based on Reinforcement Learning,” Proceedings of the 5th International Conference on Control Engineering and Artificial Intelligence, Sanya, China, pp. 24-30, 2021.
[CrossRef] [Google Scholar] [Publisher Link]
[9] Vipashi Kansal et al., “Optimizing Energy Consumption in Smart Buildings through Reinforcement Learning-Based Demand Response Strategies,” 2024 International Conference on Communication, Computer Sciences and Engineering (IC3SE), Gautam Buddha Nagar, India, pp. 1616-1621, 2024.
[CrossRef] [Google Scholar] [Publisher Link]
[10] José Manuel Álvarez-Alvarado et al., “Hybrid Techniques to Predict Solar Radiation Using Support Vector Machine and Search Optimization Algorithms: A Review,” Applied Sciences, vol. 11, no. 3, pp. 1-17, 2021.
[CrossRef] [Google Scholar] [Publisher Link]
[11] Maimouna Diagne et al., “Review of Solar Irradiance Forecasting Methods and a Proposition for Small-Scale Insular Grids,” Renewable and Sustainable Energy Reviews, vol. 27, pp. 65-76, 2013.
[CrossRef] [Google Scholar] [Publisher Link]
[12] Cyril Voyant et al., “Machine Learning Methods for Solar Radiation Forecasting: A Review,” Renewable Energy, vol. 105, pp. 569-582, 2017.
[CrossRef] [Google Scholar] [Publisher Link]
[13] Haonan Zhang et al., “Artificial Neural Network for Predicting Building Energy Performance: A Surrogate Energy Retrofits Decision Support Framework,” Buildings, vol. 12, no. 6, pp. 1-18, 2022.
[CrossRef] [Google Scholar] [Publisher Link]
[14] Tahereh Shojaei, and Alireza Mokhtar, “Forecasting Energy Consumption with a Novel Ensemble Deep Learning Framework,” Journal of Building Engineering, vol. 96, 2024.
[CrossRef] [Google Scholar] [Publisher Link]
[15] S. Siva Suriya Narayanan, and S. Thangavel, “Machine Learning-Based Model Development for Battery State of Charge–Open Circuit Voltage Relationship Using Regression Techniques,” Journal of Energy Storage, vol. 49, 2022.
[CrossRef] [Google Scholar] [Publisher Link]
[16] Yann Riffonneau et al., “Optimal Power Flow Management for Grid-Connected PV Systems with Batteries,” IEEE Transactions on Sustainable Energy, vol. 2, no. 3, pp. 309-320, 2011.
[CrossRef] [Google Scholar] [Publisher Link]
[17] Angel Recalde et al., “Machine Learning and Optimization in Energy Management Systems for Plug-In Hybrid Electric Vehicles: A Comprehensive Review,” Energies, vol. 17, no. 13, pp. 1-39, 2024.
[CrossRef] [Google Scholar] [Publisher Link]
[18] Zhikang Li, Amro Alsabbagh, and Chengbin Ma, “A Cloud-Based Energy Management Framework with Local Redundancy Control Capability,” 2021 4th IEEE International Conference on Industrial Cyber-Physical Systems, Victoria, BC, Canada, pp. 873-878, 2021.
[CrossRef] [Google Scholar] [Publisher Link]
[19] Muhammad Saidu Aliero et al., “Smart Home Energy Management Systems in Internet of Things Networks for Green Cities Demands and Services,” Environmental Technology & Innovation, vol. 22, 2021.
[CrossRef] [Google Scholar] [Publisher Link]