Operational Consolidation of Adaptive Compression and Data Stream Simplification to Enhance IoT-based Women’s Safety Applications
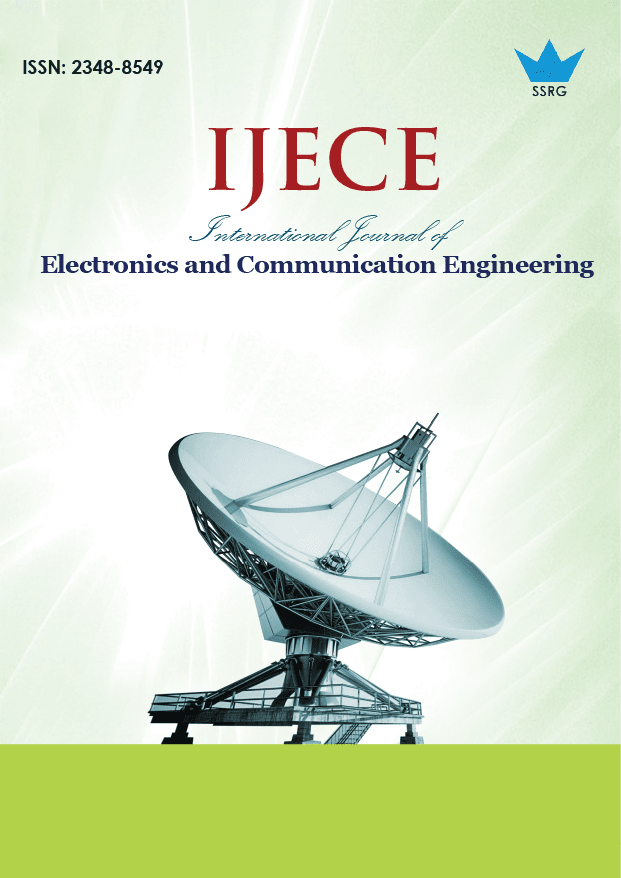
International Journal of Electronics and Communication Engineering |
© 2025 by SSRG - IJECE Journal |
Volume 12 Issue 4 |
Year of Publication : 2025 |
Authors : P. Divya, M. Kumaresan, P. Manikandan |
How to Cite?
P. Divya, M. Kumaresan, P. Manikandan, "Operational Consolidation of Adaptive Compression and Data Stream Simplification to Enhance IoT-based Women’s Safety Applications," SSRG International Journal of Electronics and Communication Engineering, vol. 12, no. 4, pp. 174-184, 2025. Crossref, https://doi.org/10.14445/23488549/IJECE-V12I4P117
Abstract:
Advanced strategies for data management are necessary because of the rapid growth of data produced by Internet of Things (IoT) devices in women's safety applications. Within the VigilNet system, this research recommends a combined approach of Adaptive Context-based Lossless Data Compression (ACLDC) and Dynamic Data Stream Simplification (DDSS) to refine the storage and processing of extensive sensor data. ACLDC efficiently lowers the demands on storage by compressing data based on contextual patterns, all whilst preserving important information to uphold the integrity of its threat detection. As a parallel process, DDSS chooses to filter and preprocess data at the source using edge computing methodologies and only sends key information to the system for thorough analysis. This dual methodology minimizes the requirements for bandwidth and storage, cuts latency, and boosts system responsiveness. In trials, the unified methodology of ACLDC and DDSS maintained a 96.8% Critical Data Retention Efficiency (CDRE) and 98% Data Reconstruction Integrity (DRI), significantly enhancing real-time threat detection accuracy and system responsiveness with optimal storage potentials. This approach improved scalability and reliability in diverse safety monitoring applications for women.
Keywords:
Compression, Data Streaming, Safety Applications, Sensor Data, Retention.
References:
[1] K.M. Karthick Raghunath et al., “Utilization of IoT-Assisted Computational Strategies in Wireless Sensor Networks for Smart Infrastructure Management,” International Journal of System Assurance Engineering and Management, vol. 15, pp. 28-34, 2024.
[CrossRef] [Google Scholar] [Publisher Link]
[2] C.E.A. Winslow, L.P. Herrington, and A.P. Gagge, “Physiological Reactions of the Human Body to Varying Environmental Temperatures,” American Journal of Physiology-Legacy Content, vol. 120, no. 1, pp. 1-22, 1937.
[CrossRef] [Google Scholar] [Publisher Link]
[3] K.M. Karthick Raghunath, and N. Rengarajan, “Response Time Optimization with Enhanced Fault-Tolerant Wireless Sensor Network Design for On-Board Rapid Transit Applications,” Cluster Computing, vol. 22, pp. 9737-9753, 2019.
[CrossRef] [Google Scholar] [Publisher Link]
[4] Ihab Nassra, and Juan V. Capella, “Data Compression Techniques in IoT-Enabled Wireless Body Sensor Networks: A Systematic Literature Review and Research Trends for QoS Improvement,” Internet of Things, vol. 23, pp. 1-29, 2023.
[CrossRef] [Google Scholar] [Publisher Link]
[5] Chunsheng Liu et al., “Robust Online Tensor Completion for IoT Streaming Data Recovery,” IEEE Transactions on Neural Networks and Learning Systems, vol. 34, no. 12, pp. 10178-10192, 2023.
[CrossRef] [Google Scholar] [Publisher Link]
[6] J. Schoeman, and L.P. Linde, “Employing a Measure of Sparseness to Investigate Sparse Data Compression in Awgn Conditions,” SAIEE Africa Research Journal, vol. 97, no. 2, pp. 157-161, 2006.
[CrossRef] [Google Scholar] [Publisher Link]
[7] Charles M. Stein et al., “Latency-Aware Adaptive Micro-Batching Techniques for Streamed Data Compression on Graphics Processing Units,” Concurrency and Computation: Practice and Experience, vol. 33, no. 11, 2021.
[CrossRef] [Google Scholar] [Publisher Link]
[8] Suha Abdulhussein Abdulzahra, Ali Kadhum M. Al-Qurabat, and Ali Kadhum Idrees, “Compression-Based Data Reduction Technique for IoT Sensor Networks,” Baghdad Science Journal, vol. 18, no. 1, pp. 184-184, 2021.
[CrossRef] [Google Scholar] [Publisher Link]
[9] Suayb S. Arslan, and Turguy Goker, “Compress-Store on Blockchain: A Decentralized Data Processing and Immutable Storage for Multimedia Streaming,” Cluster Computing, vol. 25, pp. 1957-1968, 2022.
[CrossRef] [Google Scholar] [Publisher Link]
[10] Dezhao Wang et al., “Neural Data-Dependent Transform for Learned Image Compression,” Proceedings of the IEEE/CVF Conference on Computer Vision and Pattern Recognition, pp. 17379-17388, 2022.
[Google Scholar] [Publisher Link]
[11] Jianming Wang, Wei Wang, and Jianhua Chen, “Adaptive Rate Block Compressive Sensing Based on Statistical Characteristics Estimation,” IEEE Transactions on Image Processing, vol. 31, pp. 734-747, 2021.
[CrossRef] [Google Scholar] [Publisher Link]
[12] Chunwei Liu et al., “Decomposed Bounded Floats for Fast Compression and Queries,” Proceedings of the VLDB Endowment, vol. 14, no. 11, pp. 2586-2598, 2021.
[CrossRef] [Google Scholar] [Publisher Link]
[13] N. Erratt, and Y. Liang, “Compressed Data-Stream Protocol: An Energy-Efficient Compressed Data-Stream Protocol for Wireless Sensor Networks,” IET Communications, vol. 5, no. 18, pp. 2673-2683, 2011.
[CrossRef] [Google Scholar] [Publisher Link]
[14] Sicong Liu et al., "AdaSpring: Context-adaptive and Runtime-Evolutionary Deep Model Compression for Mobile Applications,” Proceedings of the ACM on Interactive, Mobile, Wearable and Ubiquitous Technologies, vol. 5, no. 1, pp. 1-22, 2021.
[CrossRef] [Google Scholar] [Publisher Link]
[15] Priyan Malarvizhi Kumar et al., “Clouds Proportionate Medical Data Stream Analytics for Internet of Things-Based Healthcare Systems,” IEEE Journal of Biomedical and Health Informatics, vol. 26, no. 3, pp. 973-982, 2022.
[CrossRef] [Google Scholar] [Publisher Link]
[16] Hanqing Sun et al., “Intelligent Analysis of Medical Big Data Based on Deep Learning,” IEEE Access, vol. 7, pp. 142022-142037, 2019.
[CrossRef] [Google Scholar] [Publisher Link]
[17] H. Fouad et al., “Analyzing Patient Health Information Based on IoT Sensor with AI for Improving Patient Assistance in the Future Direction,” Measurement, vol. 159, 2020.
[CrossRef] [Google Scholar] [Publisher Link]
[18] I. Daubechies et al., “Nonlinear Approximation and (Deep) Networks,” Constructive Approximation, vol. 55, pp. 127-172, 2022.
[CrossRef] [Google Scholar] [Publisher Link]
[19] Wasim Akram, Mohit Jain, and C. Sweetlin Hemalatha, “Design of a Smart Safety Device for Women Using IoT,” Procedia Computer Science, vol. 165, pp. 656-662, 2019.
[CrossRef] [Google Scholar] [Publisher Link]
[20] Gabriel Signoretti et al., “An Evolving TinyML Compression Algorithm for IoT Environments Based on Data Eccentricity,” Sensors, vol. 21, no. 12, pp. 1-25, 2021.
[CrossRef] [Google Scholar] [Publisher Link]
[21] Zeyu Sun et al., “Edge Computing in Internet of Things: A Novel Sensing-Data Reconstruction Algorithm Under Intelligent-Migratoin Stragegy,” IEEE Access, vol. 8, pp. 50696-50708, 2020.
[CrossRef] [Google Scholar] [Publisher Link]
[22] Ata Ullah et al., “Secure Critical Data Reclamation Scheme for Isolated Clusters in IoT-Enabled WSN,” IEEE Internet of Things Journal, vol. 9, no. 4, pp. 2669-2677, 2022.
[CrossRef] [Google Scholar] [Publisher Link]
[23] Márcio Miguel Gomes et al., “Simplifying IoT Data Stream Enrichment and Analytics in the Edge,” Computers & Electrical Engineering, vol. 92, 2021.
[CrossRef] [Google Scholar] [Publisher Link]
[24] Jiang Bian et al., “Machine Learning in Real-Time Internet of Things (IoT) Systems: A Survey,” IEEE Internet of Things Journal, vol. 9, no. 11, pp. 8364-8386, 2022.
[CrossRef] [Google Scholar] [Publisher Link]
[25] J. Hillman, and I. Warren, “An Open Framework for Dynamic Reconfiguration,” Proceedings. 26th International Conference on Software Engineering, Edinburgh, UK, pp. 594-603, 2004.
[CrossRef] [Google Scholar] [Publisher Link]