Machine Learning-Based Design of a 2-Stack Gilbert Cell-Based Variable Gain Amplifier for Low-Noise Application
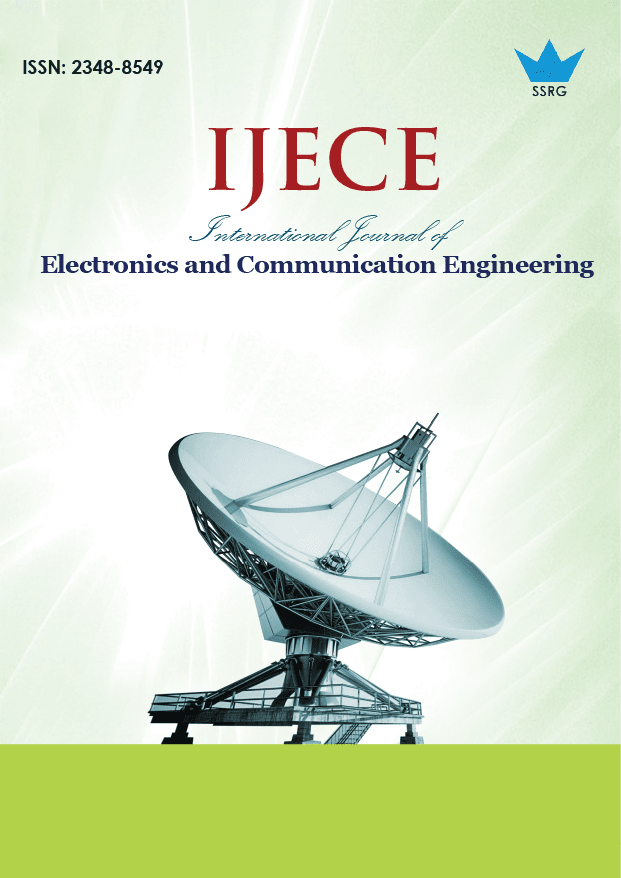
International Journal of Electronics and Communication Engineering |
© 2025 by SSRG - IJECE Journal |
Volume 12 Issue 4 |
Year of Publication : 2025 |
Authors : Dillip Kumar Sahoo, Kanhu Charan Bhuyan, Ananya Dastidar, Chandrabhanu Mishra |
How to Cite?
Dillip Kumar Sahoo, Kanhu Charan Bhuyan, Ananya Dastidar, Chandrabhanu Mishra, "Machine Learning-Based Design of a 2-Stack Gilbert Cell-Based Variable Gain Amplifier for Low-Noise Application," SSRG International Journal of Electronics and Communication Engineering, vol. 12, no. 4, pp. 185-194, 2025. Crossref, https://doi.org/10.14445/23488549/IJECE-V12I4P118
Abstract:
In this paper, a Machine Learning (ML) based design methodology is presented for the cell-based Variable Gain Amplifier (VGA). The designed unit cell consists of the Gilbert Cell configuration, with two cells stacked over each other for the current reuse and multiple channels for recording. The design can be reused for multiple recording channels to successfully record a large number of low-frequency signals. Furthermore, the Gilbert Cell design includes the integration of gm/Id Transconductance Efficiency Factor (TEF) with the k-nearest neighbor (k-NN) based searching algorithm for obtaining the geometry of the cell. The k-NN algorithm analyzes a precomputed look-up table generated by the TEF methodology for the design. The k-NN algorithm, implemented to optimize the VGA's transistor width-to-length (W/L) ratios, demonstrates exceptional precision. With an accuracy of 0.96 in selecting optimal geometric configurations, the k-NN approach significantly enhances the VGA's performance under varied operational conditions, reflecting its robustness and efficacy in real-time design adaptation. The unit cell so configured using the integrated approach is stacked over one another to give two channels for signal acquisition. The VGA gives a gain ranging from -20 dB to 54 dB with 10mW of power consumption.
Keywords:
Variable gain amplifier, Machine Learning, Gilbert Cell, Transconductance Efficiency Factor, K-nearest neighbor, Look-up Table, etc.,
References:
1] S. Devi, Gourav Tilwankar, and Rajesh Zele, “Automated Design of Analog Circuits Using Machine Learning Techniques,” 2021 25th International Symposium on VLSI Design and Test, Surat, India, pp. 1-6, 2021.
[CrossRef] [Google Scholar] [Publisher Link]
[2] Sampatrao L. Pinjare et al., “A Gm/Id Based Methodology for Designing Common Source Amplifier,” 2018 2nd International Conference on Micro-Electronics and Telecommunication Engineering, Ghaziabad, India, pp. 304-307, 2018.
[CrossRef] [Google Scholar] [Publisher Link]
[3] Hang Liu et al., “Cell-Based Variable-Gain Amplifiers With Accurate dB-Linear Characteristic in 0.18 μm CMOS Technology,” IEEE Journal of Solid-State Circuits, vol. 50, no. 2, pp. 586-596, 2015.
[CrossRef] [Google Scholar] [Publisher Link]
[4] Inyoung Choi, Heesong Seo, and Bumman Kim, “Accurate dB-Linear Variable Gain Amplifier with Gain Error Compensation,” IEEE Journal of Solid-State Circuits, vol. 48, no. 2, pp. 456-464, 2013.
[CrossRef] [Google Scholar] [Publisher Link]
[5] W.C. Song et al., “High Frequency/High Dynamic Range CMOS VGA,” Electronics Letters, vol. 36, no. 13, pp. 1096-1098, 2000.
[CrossRef] [Google Scholar] [Publisher Link]
[6] Hassan Elwan, Ahmet Tekin, and Kenneth Pedrotti, “A Differential-Ramp Based 65 dB-Linear VGA Technique in 65 nm CMOS,” IEEE Journal of Solid-State Circuits, vol. 44, no. 9, pp. 2503-2514, 2009.
[CrossRef] [Google Scholar] [Publisher Link]
[7] Behzad Razavi, Fundamentals of Microelectronics, 2nd ed., Wiley, pp. 1-903, 2013.
[Google Scholar] [Publisher Link]
[8] Phillip E. Allen, and Douglas R. Holberg, CMOS Analog Circuit Design, Oxford University Press, USA, pp. 1-932, 2011.
[Google Scholar] [Publisher Link]
[9] R. Jacob Baker, CMOS: Circuit Design, Layout, and Simulation, 3rd ed., Wiley-IEEE Press, pp. 1-1208, 2011.
[Google Scholar] [Publisher Link]
[10] Shasanka Sekhar Rout, Sushanta Kumar Mohapatra, and Kabiraj Sethi, “Design of 2.4 GHz Improved Current Reuse Gilbert Mixer with Source Degeneration Technique,” Wireless Personal Communications, vol. 122, pp. 3875-3887, 2022.
[CrossRef] [Google Scholar] [Publisher Link]
[11] Marcus Gavell et al., “Design and Analysis of a Wideband Gilbert Cell VGA in 0.25- μm InP DHBT Technology With DC-40-GHz Frequency Response,” IEEE Transactions on Microwave Theory and Techniques, vol. 65, no. 10, pp. 3962-3974, 2017.
[CrossRef] [Google Scholar] [Publisher Link]
[12] Thangarasu Bharatha Kumar, Kaixue Ma, and Kiat Seng Yeo, “A 4 GHz 60 dB Variable Gain Amplifier with Tunable DC Offset Cancellation in 65 nm CMOS,” IEEE Microwave and Wireless Components Letters, vol. 25, no. 1, pp. 37-39, 2015.
[CrossRef] [Google Scholar] [Publisher Link]
[13] Nameirakpam Premjit Singh, Anil Kumar Gautam, and Tripurari Sharan, 13 - An Insight into the Hardware and Software Aspects of a BCI System with Focus on Ultra-Low Power Bulk Driven OTA and Gm-C Based Filter Design, and a Detailed Review of the Recent AI/ML Techniques, Artificial Intelligence-Based Brain-Computer Interface, Academic Press, pp. 283-315, 2022.
[CrossRef] [Google Scholar] [Publisher Link]
[14] So-Young Kang, Seung-Tak Ryu, and Chul-Soon Park, “A Precise Decibel-Linear Programmable Gain Amplifier Using a Constant Current-Density Function,” IEEE Transactions on Microwave Theory and Techniques, vol. 60, no. 9, pp. 2843-2850, 2012.
[CrossRef] [Google Scholar] [Publisher Link]
[15] Yu-Lun Wei, Hsiao-Chin Chen, and Chi-Yin Chung, “1.35 GHz Programmable Gain Amplifier for 5G Mobile Communication,” 2017 International Conference on Applied System Innovation, Sapporo, Japan, pp. 618-621, 2017.
[CrossRef] [Google Scholar] [Publisher Link]
[16] Xiong Song et al., “A Wideband dB-Linear VGA with Temperature Compensation and Active Load,” IEEE Transactions on Circuits and Systems I: Regular Papers, vol. 66, no. 9, pp. 3279-3287, 2019.
[CrossRef] [Google Scholar] [Publisher Link]
[17] Yangtao Dong et al., “A Wideband Variable-Gain Amplifier with a Negative Exponential Generation in 40-nm CMOS Technology,” 2020 IEEE Radio Frequency Integrated Circuits Symposium, Los Angeles, CA, USA, pp. 375-378, 2020.
[CrossRef] [Google Scholar] [Publisher Link]
[18] Murat Eskiyerli, and Alison Payne, “"Square Root Domain" Filter Design and Performance,” Analog Integrated Circuits and Signal Processing, vol. 22, pp. 231-243, 2000.
[CrossRef] [Google Scholar] [Publisher Link]
[19] Yuan Wang et al., “Topology Generic DC-Model for Accelerating Analog Circuit Optimization,” 2023 International Symposium of Electronics Design Automation, Nanjing, China, pp. 65-70, 2023.
[CrossRef] [Google Scholar] [Publisher Link]
[20] F. Silveira, D. Flandre, and P.G.A. Jespers, “A g/sub m//I/sub D/ Based Methodology for the Design of CMOS Analog Circuits and its Application to the Synthesis of a Silicon-on-Insulator Micropower OTA,” IEEE Journal of Solid-State Circuits, vol. 31, no. 9, pp. 1314-1319, 1996.
[CrossRef] [Google Scholar] [Publisher Link]
[21] Mostafa N. Sabry, Hesham Omran, and Mohamed Dessouky, “Systematic Design and Optimization of Operational Transconductance Amplifier Using gm/ID Design Methodology,” Microelectronics Journal, vol. 75, pp. 87-96, 2018.
[CrossRef] [Google Scholar] [Publisher Link]
[22] Madhusmita Panda et al., “An Evolutionary-Based Design Methodology for Performance Enhancement of a Folded-Cascode OTA Using Symbiotic Organisms Search Algorithm and gm/ID Technique,” Analog Integrated Circuits and Signal Processing, vol. 105, pp. 215-227, 2020.
[CrossRef] [Google Scholar] [Publisher Link]
[23] Hesham Omran, Mohamed H. Amer, and Ahmed M. Mansour, “Systematic Design of Bandgap Voltage Reference Using Precomputed Lookup Tables,” IEEE Access, vol. 7, pp. 100131-100142, 2019.
[CrossRef] [Google Scholar] [Publisher Link]
[24] Abdelaziz Lberni et al., “Analog Circuit Sizing Based on Evolutionary Algorithms and Deep Learning,” Expert Systems with Applications, vol. 237, 2024.
[CrossRef] [Google Scholar] [Publisher Link]
[25] Jie Gui et al., “Fast kNN Search in Weighted Hamming Space with Multiple Tables,” IEEE Transactions on Image Processing, vol. 30, pp. 3985-3994, 2021.
[CrossRef] [Google Scholar] [Publisher Link]
[26] Fouad Khelifi, and Jianmin Jiang, “K-NN Regression to Improve Statistical Feature Extraction for Texture Retrieval,” IEEE Transactions on Image Processing, vol. 20, no. 1, pp. 293-298, 2011.
[CrossRef] [Google Scholar] [Publisher Link]
[27] Nova Kristian, Fikri Adzikri, and Mia Rizkinia, “Ethereum Price Prediction Comparison Using k-NN and Multiple Polynomial Regression,” 2021 17th International Conference on Quality in Research (QIR): International Symposium on Electrical and Computer Engineering, Depok, Indonesia, pp. 141-146, 2021.
[CrossRef] [Google Scholar] [Publisher Link]
[28] Fabian Pedregosa et al., “Scikit-learn: Machine Learning in Python,” Journal of Machine Learning Research, vol. 12, pp. 2825-2830, 2011.
[Google Scholar] [Publisher Link]
[29] M.A. Penna, “Camera Calibration: A Quick and Easy Way to Determine the Scale Factor,” IEEE Transactions on Pattern Analysis and Machine Intelligence, vol. 13, pp. 1240-1245, 1991.
[CrossRef] [Google Scholar] [Publisher Link]