A Novel Deep Learning-Based System for Real-Time Temperature Monitoring of Bone Hyperthermia
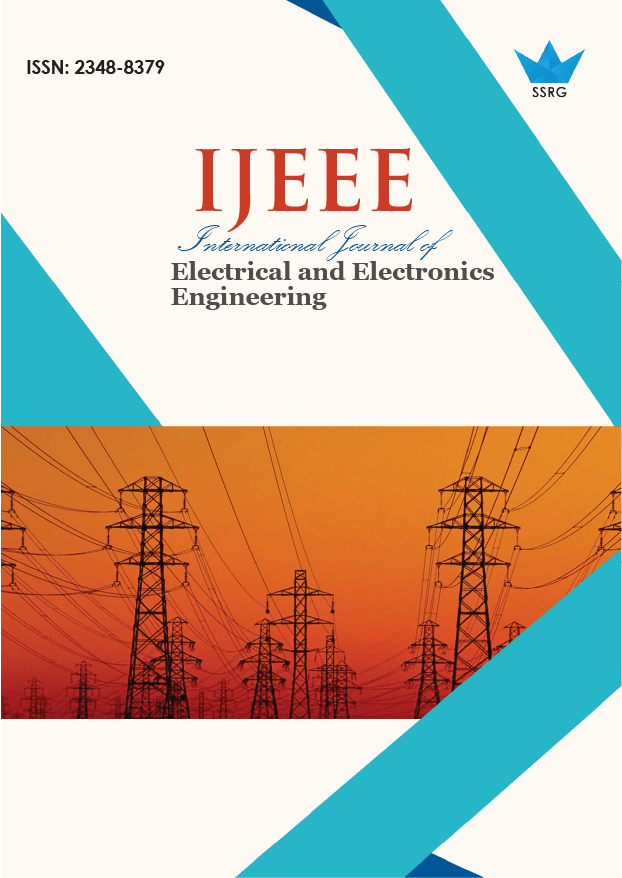
International Journal of Electrical and Electronics Engineering |
© 2023 by SSRG - IJEEE Journal |
Volume 10 Issue 1 |
Year of Publication : 2023 |
Authors : Priscilla Whitin, V. Jayasankar |
How to Cite?
Priscilla Whitin, V. Jayasankar, "A Novel Deep Learning-Based System for Real-Time Temperature Monitoring of Bone Hyperthermia," SSRG International Journal of Electrical and Electronics Engineering, vol. 10, no. 1, pp. 187-196, 2023. Crossref, https://doi.org/10.14445/23488379/IJEEE-V10I1P118
Abstract:
High-intensity focused ultrasound is a form of thermal therapy that does not involve any incisions and is used for hyperthermia and removal treatments. Monitoring the temperature is essential for such operations to provide the required quantity of thermal dosage to the target region without causing damage to the strong tissues that are located in the surrounding area. In order to accomplish this goal, a number of different medical imaging approaches have been developed. Magnetic resonance imaging allows temperature readings to be taken with a high degree of precision. Because it does not emit ionizing radiation, is easily accessible, and has a low cost, ultrasound is a medical imaging modality that is regarded favourably for use in temperature monitoring. This is of particular importance in cases involving bone tumors because of the sensitive tissues that are located nearby. Due to the fact that temperature affects both the rapidity of sound and the reduction of ultrasound waves, it is possible to estimate temperature by utilizing the physical features of ultrasound. In this article, we suggest a system that uses ultrasonography and a deep learning methodology to monitor the temperature. During HIFU therapy, the system collects data from the ultrasound channels and alternates between ablation and monitoring phases. It was created with this functionality in mind. During the monitoring phase, the ultrasonic elements in the probe are in charge of receiving ultrasound pulses that have been consecutively sent from the 256 HIFU components. We train a convolutional long short-term memory computational model using ultrasonic data to generate temperature images. Magnetic resonance thermometry readings are compared to the resulting temperature images. The mean and maximum discrepancies between each picture are calculated as a means of gauging the performance of the proposed neural network. This research suggests using a neural network to recreate thermal pictures. For this purpose, we utilize the ultrasound channel data in conjunction with a CLSTM neural network to create temperature pictures. Images of temperatures are compared with those acquired using magnetic resonance thermometry. Because this approach can acquire the progression of temperature from a vast quantity of facts, it may be less sensitive to the placement of the ultrasonic element. Phantom research allowed us to verify the accuracy of the temperature image reconstruction approach. A technique of temperature monitoring that makes use of an external ultrasonic component and deep learning reconstruction appears to be feasible, according to the encouraging findings obtained.
Keywords:
Temperature, HIFU, Bone tumors, CLSTM, Magnetic resonance.
References:
[1] T. Kujawska et al., “Annular Phased Array Transducer for Preclinical Testing of Anti-Cancer Drug Efficacy on Small Animals,” Ultrasonics, vol. 76, pp. 92-98, 2017. Crossref, https://doi.org/10.1016/j.ultras.2016.12.008
[2] G. Litjens et al., “A Survey on Deep Learning in Medical Image Analysis,” Medical Image Analysis, vol. 42, pp. 60-88, 2017. Crossref, https://doi.org/10.1016/j.media.2017.07.005
[3] K. Prema, and A. Kumar Kombaiya, “An Unsupervised Deep Feature Selection and Ensemble Deep Learning Model for Cancer Classification,” International Journal of Engineering Trends and Technology, vol. 70, no. 9, pp. 20-33, 2022. Crossref, https://doi.org/10.14445/22315381/IJETT-V70I9P203
[4] S. Song et al., “Monitoring Microwave Ablation Using Ultrasound Homodyned K Imaging Based on the Noise-Assisted Correlation Algorithm: an Ex Vivo Study,” Ultrasonics, vol. 110, p. 106287, 2021. Crossref, https://doi.org/10.1016/j.ultras.2020.106287
[5] F. Fani et al., “CT-Based Thermometry: an Overview,” International Journal of Hyperthermia : The Official Journal of European Society for Hyperthermic Oncology, North American Hyperthermia Group, vol. 30, no. 4, pp. 219-227, 2014. Crossref, https://doi.org/10.3109/02656736.2014.922221
[6] Mohammed Saad Alqahtani, “Primary Hemophagocytic Lymphohistocytosis in Adult Woman; A Case Report,” SSRG International Journal of Medical Science, vol. 4, no. 4, pp. 1-3, 2017. Crossref, https://doi.org/10.14445/23939117/IJMS-V4I4P101
[7] P. Bruners et al., “Multi-Slice Computed Tomography: a Tool for Non-Invasive Temperature Measurement?,” International Journal of Hyperthermia : The Official Journal of European Society for Hyperthermic Oncology, North American Hyperthermia Group, vol. 26, no. 4, pp. 359-365, 2010. Crossref, https://doi.org/10.3109/02656731003605654
[8] Ngwobia Peter Agwu, and Ahmed Mohammed Umar, “The Role of Bisphosphonates in the Management of Bone Metastasis,” SSRG International Journal of Medical Science, vol. 7, no. 10, pp. 5-11, 2020. Crossref, https://doi.org/10.14445/23939117/IJMS-V7I10P102
[9] D. Kokuryo, E. Kumamoto, and K. Kuroda, “Recent Technological Advancements in Thermometry,” Advanced Drug Delivery Reviews, vol. 163-164, pp. 19-39, 2020. Crossref, https://doi.org/10.1016/j.addr.2020.11.001
[10] M. Franckena et al., “Hyperthermia Dose-Effect Relationship in 420 Patients with Cervical Cancer Treated with Combined Radiotherapy and Hyperthermia,” European Journal of Cancer, vol. 45, no. 11, pp. 1969-1978, 2009. Crossref, https://doi.org/10.1016/j.ejca.2009.03.009
[11] P. Nishaa et al., “Giant Cell Tumour of the Sternum-Two Cases,” SSRG International Journal of Medical Science, vol. 3, no. 3, pp. 1-3, 2016. Crossref, https://doi.org/10.14445/23939117/IJMS-V3I3P101
[12] G. Litjens et al., “A Survey on Deep Learning in Medical Image Analysis,” Medical Image Analysis, vol. 42, no. 7, pp. 60-88, 2017. Crossref, https://doi.org/10.1016/j.media.2017.07.005
[13] Justin Ker et al., “Deep Learning Applications in Medical Image Analysis,” IEEE Access, vol. 6, pp. 9375-9389, 2018, Crossref, https://doi.org/10.1109/ACCESS.2017.2788044
[14] Dr. Shobha Gusain, “Modifiable Risk Factors for Breast and Cervical Cancer and Their Association with Sample Characteristics,” SSRG International Journal of Nursing and Health Science, vol. 6, no. 1, pp. 10-16, 2020. Crossref, https://doi.org/10.14445/24547484/IJNHS-V6I1P102
[15] V. Gulshan et al., “Development and Validation of a Deep Learning Algorithm for Detection of Diabetic Retinopathy in Retinal Fundus Photographs,” Jama, vol. 316, no. 22, pp. 2402-2410, 2016. Crossref, https://doi.org/10.1001/jama.2016.17216, 2016
[16] Diego Ardila et al., “End-To-End Lung Cancer Screening with Three-Dimensional Deep Learning on Low-Dose Chest Computed Tomography,” Nature Medicine, vol. 25, pp. 954-961, 2019. Crossref, https://doi.org/10.1038/s41591-019-0447-x
[17] K. Munir et al., “Cancer Diagnosis Using Deep Learning: a Bibliographic Review,” Cancers, vol. 11, no. 9, p. 908873, 2019. Crossref, https://doi.org/10.3390/cancers11091235
[18] R. Raman et al., “Fundus Photograph-Based Deep Learning Algorithms in Detecting Diabetic Retinopathy,” Eye, vol. 33, no. 1, pp. 97–109, 2019. Crossref, https://doi.org/10.1038/s41433-018-0269-y
[19] P. Schelb et al., “Classification of Cancer at Prostate MRI: Deep Learning Versus Clinical PI-RADS Assessment,” Radiology, vol. 293, no. 3, pp. 607–17. 2019. Crossref, https://doi.org/10.1148/radiol.2019190938
[20] S. Wang et al., “Predicting EGFR Mutation Status in Lung Adenocarcinoma on Computed Tomography Image Using Deep Learning,” European Respiratory Journal, vol. 53, no. 3, p. 1800986, 2019. Crossref, https://doi.org/10.1183/13993003.00986- 2018 Priscilla Whitin & V. Jayasankar/ IJEEE, 10(1), 187-196, 2023 196
[21] Y. Xu et al., “Deep Learning Predicts Lung Cancer Treatment Response from Serial Medical Imaging,” Clinical cancer research : an official journal of the American Association for Cancer Research, vol. 25, no. 11, pp. 3266-3275, 2019. Crossref, https://doi.org/10.1158/1078- 0432.Ccr-18-2495
[22] S. H. AlDubayan et al., “Detection of Pathogenic Variants with Germline Genetic Testing Using Deep Learning vs Standard Methods in Patients with Prostate Cancer and Melanoma,” Jama, vol. 324, no. 19, pp. 1957-1969, 2020. Crossref, https://doi.org/10.1001/ jama.2020.20457
[23] W. Bulten et al., “Automated Deep-Learning System for Gleason Grading of Prostate Cancer Using Biopsies: a Diagnostic Study,” Lancet Oncology, vol. 21, no. 2, pp. 233-241, 2020. Crossref, https://doi.org/10.1016/s1470-2045(19)30739-9
[24] S. W. Chung et al., “Automated Detection and Classification of the Proximal Humerus Fracture by Using Deep Learning Algorithm,” Acta Orthopaedica, vol. 89, no. 4, pp. 468-473, 2018. Crossref, https://doi.org/10.1080/ 17453674.2018.1453714
[25] D. H. Kim, and T. MacKinnon, “Artificial Intelligence in Fracture Detection: Transfer Learning from Deep Convolutional Neural Networks,” Clinical radiology, vol. 73, no. 5, pp. 439-445, 2018. Crossref, https://doi.org/10.1016/j.crad.2017.11.015
[26] N. Tomita et al., “Deep Neural Networks for Automatic Detection of Osteoporotic Vertebral Fractures on CT Scans,” Computers in Biology and Medicine, vol. 98, pp. 8-15, 2018. Crossref, https://doi.org/10.1016/j.compbiomed.2018.05.011
[27] C. T. Cheng et al., “Application of a Deep Learning Algorithm for Detection and Visualization of Hip Fractures on Plain Pelvic Radiographs,” European Radiology, vol. 29, no. 10, pp. 5469-5477, 2019. Crossref, https://doi.org/10.1007/s00330-019-06167-y
[28] I. Š tajduhar et al., “Semi-Automated Detection of Anterior Cruciate Ligament Injury from MRI,” Computer Methods and Programs in Biomedicine, vol. 140, pp. 151-164, 2017. Crossref, https://doi.org/10.1016/j.cmpb.2016.12.006
[29] N. Bien et al., “Deep-Learning Assisted Diagnosis for Knee Magnetic Resonance Imaging: Development and Retrospective Validation of MRNet,” PloS Med, vol. 15, no. 11, p. e1002699. 2018. Crossref, https://doi.org/10.1371/journal.pmed.1002699
[30] C. Spampinato et al., “Deep Learning for Automated Skeletal Bone Age Assessment in X-Ray Images,” Medical Image Analysis, vol. 36, pp. 41–51, 2017. Crossref, https://doi.org/10.1016/j.media.2016.10.010
[31] S. Koitka et al., “Ossification Area Localization in Pediatric Hand Radiographs Using Deep Neural Networks for Object Detection,” PloS One, vol. 13, no. 11, pp. 0207496. 2018. Crossref, https://doi.org/10.1371/journal.pone.0207496
[32] D. B. Larson et al., “Performance of a Deep-Learning Neural Network Model in Assessing Skeletal Maturity on Pediatric Hand Radiographs,” Radiology, vol. 287, no. 1, pp. 313-322, 2018. Crossref, https://doi.org/10.1148/radiol.2017170236
[33] C. Tong et al., “A Deep Automated Skeletal Bone Age Assessment Model with Heterogeneous Features Learning,” Journal of Medical Systems, vol. 42, no. 12, pp. 249, 2018. Crossref, https://doi.org/10.1007/s10916-018-1091-6
[34] X. Ren et al., “Regression Convolutional Neural Network for Automated Pediatric Bone Age Assessment From Hand Radiograph,” IEEE Journal of Biomedical and Health Informatics, vol. 23, no. 5, pp. 2030–8, 2019. Crossref, https://doi.org/10.1109/jbhi.2018.2876916
[35] Y. Gao et al., “Bone Age Assessment Based on Deep Convolution Neural Network Incorporated with Segmentation,” International Journal of Computer Assisted Radiology and Surgery, vol. 15, no. 12, pp. 1951–62, 2020. Crossref, https://doi.org/10.1007/s11548-020-02266-0
[36] Sneha Iyer et al., “In Silico Drug Designing And Phytochemicals Prospect In Liver Cancer Therapeutics,” International Journal of Biotech Trends and Technology, vol. 11, no. 3, pp. 31-41, 2021. Crossref, http://ijbttjournal.org/archives/ijbtt-v11i3p605
[37] R. Righetti et al., “Elastographic Characterization of HIFU-Induced Lesions in Canine livers,” Ultrasound in Medicine & Biology, vol. 25, no. 7, pp. 1099-1113, 1999.
[38] Debanjoy Paul et al., “Computer-Aided And Herbal Informatics Based Drug Designing for Potential Lung Cancer Therapeutics,” International Journal of Biotech Trends and Technology, vol. 11, no. 3, pp. 8-17, 2021. Crossref, https://doi.org/10.14445/22490183/IJBTT-V11I3P602
[39] S. J. S. Ruiter, W. J. Heerink, and K. P. de Jong, “Liver Microwave Ablation: a Systematic Review of Various FDA-Approved Systems,” European Radiology, vol. 29, pp. 4026–4035, 2019. Crossref, https://doi.org/10.1007/s00330-018-5842-z
[40] Chensi Cao et al., “Deep Learning and Its Applications in Biomedicine,” Genomics Proteomics Bioinformatics, vol. 16, no. 1, pp. 17-32, 2018.
[41] M. Aruna, S. Sukumaran, and V. Srinivasan, “Hybridization of Fuzzy Label Propagation and Local Resultant Evidential Clustering Method for Cancer Detection,” International Journal of Engineering Trends and Technology, vol. 70, no. 9, pp. 34-46, 2022. Crossref, https://doi.org/10.14445/22315381/IJETT-V70I9P204
[42] D. S. Kermany et al., “Identifying Medical Diagnoses and Treatable Diseases by Image-Based Deep Learning,” Cell, vol. 172, no. 5, pp. 1122–1131.e1129, 2018. Crossref, https://doi.org/10.1016/ j.cell.2018.02.010
[43] Ijaz Sheik et al., “Current Review on Nanoparticles Targeting Colorectal Cancer,” International Journal of Biotech Trends and Technology, vol. 12, no. 3, pp. 8-17, 2022. Crossref, https://doi.org/10.14445/22490183/IJBTT-V12I3P602
[44] Jijo Paul, Thomas J. Vogl, and Annamma Chacko, “Dual-Energy Computed Tomography Thermometry During Hepatic Microwave Ablation in an Exvivo Porcine Model,” European Journal of Medical Physics, vol. 31, pp. 683–691, 2015. Crossref, https://doi.org/10.1016/j.ejmp.2015.05.014
[45] Sehyo Yune et al., “Beyond Human Perception: Sexual Dimorphism in Hand and Wrist Radiographs Is Discernible by a Deep Learning Model,” Journal of Digit Imaging, vol. 32, no. 4, pp. 665– 71, 2019. Crossref, https://doi.org/10.1007/s10278-018-0148-x