Brain Tumor Stages Prediction using FMS-DLNN Classifier and Automatic RPO-RG Segmentation
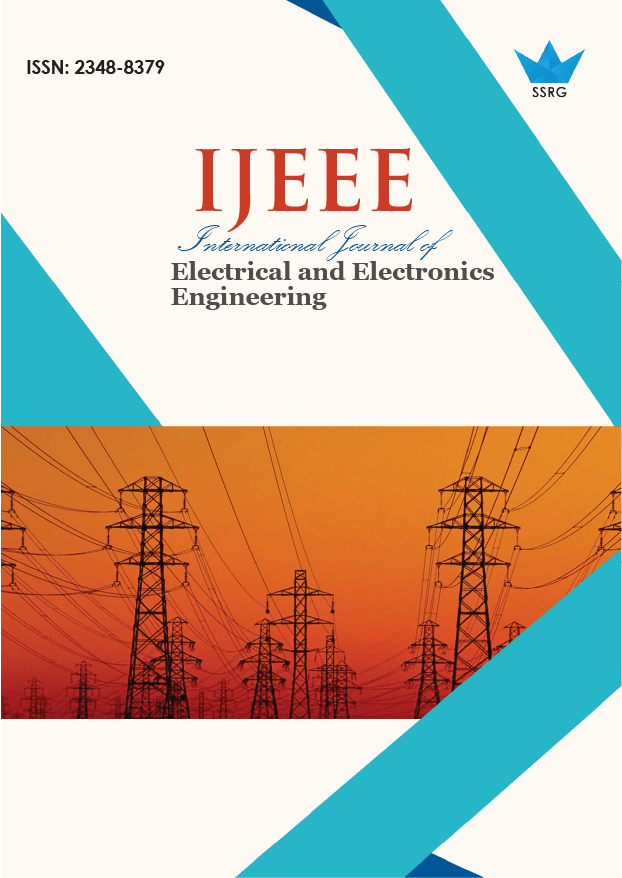
International Journal of Electrical and Electronics Engineering |
© 2023 by SSRG - IJEEE Journal |
Volume 10 Issue 2 |
Year of Publication : 2023 |
Authors : R. Sakthi Prabha, M. Vadivel |
How to Cite?
R. Sakthi Prabha, M. Vadivel, "Brain Tumor Stages Prediction using FMS-DLNN Classifier and Automatic RPO-RG Segmentation," SSRG International Journal of Electrical and Electronics Engineering, vol. 10, no. 2, pp. 110-121, 2023. Crossref, https://doi.org/10.14445/23488379/IJEEE-V10I2P111
Abstract:
Recently, one amongst the deadliest diseases is Brain Tumor (BT). A cluster of abnormal cells, which are clustered around the brain’s inner portion, is contained by the tumour. It amplifies the Intra cranial pressure; thus, the tumour cell growth mounts, leading to death. Hence, diagnosing BTs at an early stage is desirable. For BT-type classification, various ideas are suggested by the prevailing techniques. However, they did not concentrate on the stages of BT. This research aims to predict the tumour’s stages utilizing Range Pelican Optimization-based Region Growing (RPO-RG) segmentation and Fuzzy Memorized and SigTan-based Deep Learning Neural Network (FMS-DLNN) classifier. Primarily, the Gaussian Kernelized Kuwahara Filter (GKKF) pre-processed the input MRI images. Utilizing the Enhanced Farthest First Clustering (EFFC) algorithm, the noiseremoved image is clustered. After that, the tumor region is segmented by the RPO-RG algorithm. After segmentation, features are extracted; also, by utilizing the Logarithmic Fisher Discriminant Analysis (LFDA), the features’ dimensionality is reduced. Lastly, for classifying the BT stages, the necessary features are given to FMS-DLNN. With the prevailing approaches, the proposed mechanism is analogized. The experimental assessment exhibits that the proposed system was more efficient in classifying the various stages of tumours.
Keywords:
Deep learning, Magnetic Resonance Imaging (MRI), Brain tumor, Segmentation, Fuzzy Memorized and Sigtan-Deep Learning Neural Network (FMS-DLNN), Clustering.
References:
[1] Ahmed H. Abdel-Gawad, Lobna A. Said, and Ahmed G. Radwan, “Optimized Edge Detection Technique for Brain Tumor Detection in MR Images,” IEEE Access, vol. 8, pp. 136243–136259, 2020. Crossref, https://doi.org/10.1109/ACCESS.2020.3009898
[2] Mahnoor Ali et al., “Brain Tumour Image Segmentation Using Deep Networks,” IEEE Access, vol. 8, pp. 153589–153598, 2020. Crossref, https://doi.org/10.1109/ACCESS.2020.3018160
[3] Javaria Amin et al., “Brain Tumor Detection by Using Stacked Autoencoders in Deep Learning,” Journal of Medical Systems, vol. 44, p. 32, 2020. Crossref, https://doi.org/10.1007/s10916-019-1483-2
[4] S. Bhuvaneswari et al., "Disease Detection in Plant Leaf using LNet Based on Deep Learning," International Journal of Engineering Trends and Technology, vol. 70, no. 9, pp. 64-75, 2022. Crossref, https://doi.org/10.14445/22315381/IJETT-V70I9P207
[5] Javeria Amin et al., “A New Approach for Brain Tumor Segmentation and Classification Based on Score Level Fusion Using Transfer Learning,” Journal of Medical Systems, vol. 43, p. 326, 2019. Crossref, https://doi.org/10.1007/s10916-019-1453-8
[6] Dr R Cristin, Dr K Suresh Kumar, and Dr P Anbhazhagan, “Severity Level Classification of Brain Tumor Based on MRI Images Using Fractional-Chicken Swarm Optimization Algorithm,” Computer Journal, vol. 64, no. 10, pp. 1514–1530, 2021. Crossref, https://doi.org/10.1093/comjnl/bxab057
[7] Wu Deng et al., “Deep Learning-Based HCNN and CRF-RRNN Model for Brain Tumor Segmentation,” IEEE Access, vol. 8, pp. 26665–26675, 2020. Crossref, https://doi.org/10.1109/ACCESS.2020.2966879
[8] Francisco Javier Díaz-Pernas et al., “A Deep Learning Approach for Brain Tumor Classification and Segmentation Using a Multiscale Convolutional Neural Network,” Healthcare, vol. 9, no. 2, p. 153, 2021. Crossref, https://doi.org/10.3390/healthcare9020153
[9] R. Surendiran et al., " Exploring the Cervical Cancer Prediction by Machine Learning and Deep Learning with Artificial Intelligence Approaches," International Journal of Engineering Trends and Technology, vol. 70, no. 7, pp. 94-107, 2022. Crossref, https://doi.org/10.14445/22315381/IJETT-V70I7P211
[10] Abdu Gumaei et al., “A Hybrid Feature Extraction Method with Regularized Extreme Learning Machine for Brain Tumor Classification,” IEEE Access, vol. 7, pp. 36266–36273, 2019. Crossref, https://doi.org/10.1109/ACCESS.2019.2904145
[11] N. Pradeepa, A. Renuga Devi, and Saumiya Jose Thomas, "Survey of MRI Brain Image Segmentation Methods," SSRG International Journal of Electronics and Communication Engineering, vol. 2, no. 2, pp. 21-23, 2015. Crossref, https://doi.org/10.14445/23488549/IJECE-V2I2P105
[12] P. Harish, and S. Baskar, “MRI Based Detection and Classification of Brain Tumor Using Enhanced Faster R-CNN and Alex Net Model,” Materials Today: Proceedings, 2020. Crossref, https://doi.org/10.1016/j.matpr.2020.11.495
[13] Ali Hatamizadeh et al., “Swin UNETR: Swin Transformers for Semantic Segmentation of Brain Tumors in MRI Images,” Lecture Notes in Computer Science, pp. 272–284, 2022. Crossref, https://doi.org/10.1007/978-3-031-08999-2_22
[14] Dr Ravi N et al., "MRI Evaluation of Different Spectrum of Spinal Tumors," SSRG International Journal of Medical Science, vol. 1, no. 2, pp. 11-23, 2014. Crossref, https://doi.org/10.14445/23939117/IJMS-V1I2P102
[15] Kai Hu et al., “Brain Tumor Segmentation Using Multi-Cascaded Convolutional Neural Networks and Conditional Random Field,” IEEE Access, vol. 7, pp. 92615–92629, 2019. Crossref, https://doi.org/10.1109/ACCESS.2019.2927433
[16] Md Khairul Islam et al., “Brain Tumor Detection in MR Image Using Superpixels, Principal Component Analysis and Template Based K-Means Clustering Algorithm,” Machine Learning with Applications, vol. 5, p. 100044, 2021. Crossref, https://doi.org/10.1016/j.mlwa.2021.100044
[17] Ravi Ningappa, and Bagath Singh K, "Study of Brain Mass Lesions by MRI- On Special Sequences," SSRG International Journal of Medical Science, vol. 2, no. 2, pp. 1-10, 2015. Crossref, https://doi.org/10.14445/23939117/IJMS-V2I2P101
[18] Jaeyong Kang et al., “MRI-Based Brain Tumor Classification Using Ensemble of Deep Features and Machine Learning Classifiers,” Sensors, vol. 21, no. 6, p. 2222, 2021. Crossref, https://doi.org/10.3390/s21062222
[19] Gökay Karayegen, and Mehmet Feyzi Aksahin, “Brain Tumor Prediction on MR Images with Semantic Segmentation by Using Deep Learning Network and 3D Imaging of Tumor Region,” Biomedical Signal Processing and Control, vol. 66, p. 102458, 2021. Crossref, https://doi.org/10.1016/j.bspc.2021.102458
[20] Sindhia, Ramanitharan, Shankar Mahalingam, and Soundarya Prithiesh, "Brain Tumor Detection Using MRI by Classification and Segmentation," SSRG International Journal of Medical Science, vol. 6, no. 3, pp. 12-14, 2019. Crossref, https://doi.org/10.14445/23939117/IJMS-V6I3P103
[21] Nivea Kesav, and M.G. Jibukumar, “Efficient and Low Complex Architecture for Detection and Classification of Brain Tumor using RCNN with Two Channel CNN,” Journal of King Saud University - Computer and Information Sciences, vol. 34, no. 8, pp. 6229– 6242, 2022. Crossref, https://doi.org/10.1016/j.jksuci.2021.05.008
[22] Amjad Rehman Khan et al., “Brain Tumor Segmentation Using K-Means Clustering and Deep Learning with Synthetic Data Augmentation for Classification,” Microscopy Research and Technique, vol. 84, no. 7, pp. 1389-1399, 2021. Crossref, https://doi.org/10.1002/jemt.23694
[23] Muhammad Attique Khan et al., “Multimodal Brain Tumor Classification Using Deep Learning and Robust Feature Selection: A Machine Learning Application for Radiologists,” Diagnostics, vol. 10, no. 8, p. 565, 2020. Crossref, https://doi.org/10.3390/diagnostics10080565
[24] R. Surendiran et al., "Effective Autism Spectrum Disorder Prediction to Improve the Clinical Traits using Machine Learning Techniques," International Journal of Engineering Trends and Technology, vol. 70, no. 4, pp.343-359, 2022. Crossref, https://doi.org/10.14445/22315381/IJETT-V70I4P230
[25] Muhammad Waqas Nadeem et al., “Brain Sciences Brain Tumor Analysis Empowered with Deep Future Challenges,” Brain Sciences, vol. 10, no. 2, p. 118, 2020. Crossref, https://doi.org/10.3390/brainsci10020118
[26] P. Nagaraj et al., “Programmed Multi-Classification of Brain Tumor Images Using Deep Neural Network,” Proceedings of the International Conference on Intelligent Computing and Control Systems, pp. 865–870, 2020. Crossref, https://doi.org/10.1109/ICICCS48265.2020.9121016
[27] Fareed M.mohammed, Mustafa M.Essa, and Ahmed W. Maseer, "Comparison between MRI and CT-Scan in Diagnosis the Brain Tumor Images," SSRG International Journal of Medical Science, vol. 6, no. 5, pp. 1-4, 2019. Crossref, https://doi.org/10.14445/23939117/IJMS-V6I5P101
[28] Bing Niu et al., “Glioma Stages Prediction Based on Machine Learning Algorithm Combined with Protein-Protein Interaction Networks,” Genomics, vol. 112, no. 1, pp. 837–847, 2020. Crossref, https://doi.org/10.1016/j.ygeno.2019.05.024
[29] Neelum Noreen et al., “A Deep Learning Model based on Concatenation Approach for the Diagnosis of Brain Tumor,” IEEE Access, vol. 8, pp. 55135–55144, 2020. Crossref, https://doi.org/10.1109/ACCESS.2020.2978629
[30] Hasnain Ali Shah et al., “A Robust Approach for Brain Tumor Detection in Magnetic Resonance Images Using Finetuned EfficientNet,” IEEE Access, vol. 10, pp. 65426–65438, 2022. Crossref, https://doi.org/10.1109/ACCESS.2022.3184113
[31] Muhammad Irfan Sharif et al., “Active Deep Neural Network Features Selection for Segmentation and Recognition of Brain Tumors Using MRI Images,” Pattern Recognition Letters, vol. 129, pp. 181–189, 2020. Crossref, https://doi.org/10.1016/j.patrec.2019.11.019
[32] Ankit Vidyarthi et al., “Machine Learning Assisted Methodology for Multiclass Classification of Malignant Brain Tumors,” IEEE Access, vol. 10, pp. 50624–50640, 2022. Crossref, https://doi.org/10.1109/ACCESS.2022.3172303
[33] R. Tamilaruvi et al., “Brain Tumor Detection in MRI Images using Convolutional Neural Network Technique,” SSRG International Journal of Electrical and Electronics Engineering, vol. 9, no. 12, pp. 198-208, 2022. Crossref, https://doi.org/10.14445/23488379/IJEEE-V9I12P118