Deep Learning-Based Semantic Segmentation Models for Prostate Gland Segmentation
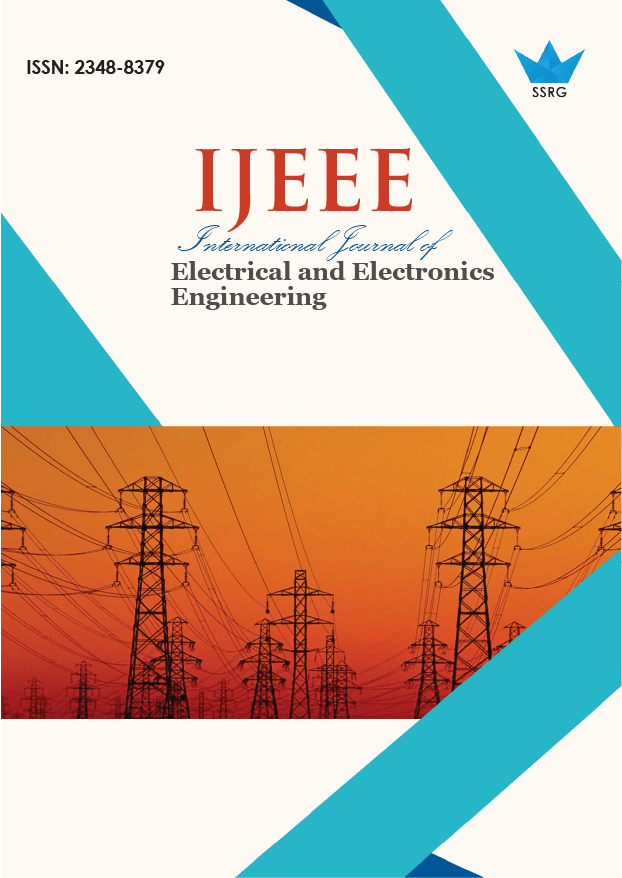
International Journal of Electrical and Electronics Engineering |
© 2023 by SSRG - IJEEE Journal |
Volume 10 Issue 2 |
Year of Publication : 2023 |
Authors : M. N. Rajesh, B. S. Chandrasekar |
How to Cite?
M. N. Rajesh, B. S. Chandrasekar, "Deep Learning-Based Semantic Segmentation Models for Prostate Gland Segmentation," SSRG International Journal of Electrical and Electronics Engineering, vol. 10, no. 2, pp. 157-171, 2023. Crossref, https://doi.org/10.14445/23488379/IJEEE-V10I2P115
Abstract:
Prostate cancer (PCa) is one of the prevalent forms of cancer disease found in males due to the unusual development of cells. Early diagnosis of this PCa can be useful in terms of treatment and medication. Segmentation and classification of the PCa through manual observation are one of the diagnosis methods, but it is highly challenging due to complex boundaries and features. Machine learning-based semantic segmentation architecture models consume more energy and processing time and will lead to reduced scalability and reliability. In order to tackle these limitations, deep learning-based semantic segmentation architecture can be used as it has more advantages in discriminating the features of the lesions efficiently and accurately. The main aim of this work is to segment the PCa lesions accurately and efficiently. Hence, deep learning-based semantic segmentation model-based architectures such as U-Net, Linknet, and PSPNet are proposed in this research. These models are equipped with a backbone as Inception-ResNet-v2 CNN architecture for prostate cancer gland segmentation. Nearest neighbour interpolation and normalization methods are employed as the preprocessing technique for enhancing the PCa MRI images. The normalized image was taken for processing various settings of U-Net, LinkNet and PSP-Net architectures for performing segmentation and for optimizing these models, Adam, Adamax and Nadam optimizers are used. The experiment was performed using NCI-ISBI 2013 dataset. Performance analysis of the proposed models is evaluated using Intersection of Union (IoU) scores, where the LinkNet optimized with Adamax obtained a best IoU score of 0.763337802.
Keywords:
PCa semantic segmentation, U-Net, LinkNet, PSP-Net, Inception-ResNet-v2.
References:
[1] David Gillespie et al., “Deep Learning in Magnetic Resonance Prostate Segmentation: A Review and a New Perspective,” Electrical Engineering and Systems Science, Image and Video Processing, arXiv preprint, 2020. Crossref, https://doi.org/10.48550/arXiv.2011.07795
[2] Xiangbin Liu et al., “A Review of Deep-Learning-Based Medical Image Segmentation Methods,” Sustainability, vol. 13, no. 3, pp. 1224, 2021. Crossref, https://doi.org/10.3390/su13031224
[3] Zia Khan et al., “Recent Automatic Segmentation Algorithms of MRI Prostate Regions: A Review,” IEEE Access, vol. 9, pp. 97878- 97905, 2021. Crossref, https://doi.org/10.1109/ACCESS.2021.3090825
[4] Leonardo Rundo et al., “CNN-Based Prostate Zonal Segmentation on T2-weighted MR Images: A Cross-dataset Study,” Neural Approaches to Dynamics of Signal Exchanges, Smart Innovation, Systems and Technologies, vol. 151, pp. 269–280. 2020. Crossref, https://doi.org/10.1007/978-981-13-8950-4_25
[5] Fouzia Altaf et al., “Going Deep in Medical Image Analysis: Concepts, Methods, Challenges, and Future Directions,” IEEE Access, vol. 7, pp. 99540-99572, 2019. Crossref, https://doi.org/10.1109/ACCESS.2019.2929365
[6] Saeid Asgari Taghanaki et al., “Deep Semantic Segmentation of Natural and Medical Images: A Review,” Artificial Intelligence Review, vol. 54, pp. 137–178, 2021. Crossref, https://doi.org/10.1007/s10462-020-09854-1
[7] Paulo Lapa et al., “Enhancing Classification Performance of Convolutional Neural Networks for Prostate Cancer Detection on Magnetic Resonance Images: A Study with the Semantic Learning Machine,” Proceedings of the Genetic and Evolutionary Computation Conference Companion, pp. 381–382, 2019. Crossref, https://doi.org/10.1145/3319619.3322035
[8] Sushma Shrestha et al., “A Novel Solution of Using Deep Learning for Prostate Cancer Segmentation: Enhanced Batch Normalization,” Multimedia Tools and Applications, vol. 80, no. 8, pp. 21293–21313, 2021.
Crossref, https://doi.org/10.1007/s11042-021-10779-2
[9] Nader Aldoj et al., “Automatic Prostate and Prostate Zones Segmentation of Magnetic Resonance Images Using DenseNet‑like U‑net,” Scientific Reports, vol. 10, no. 14315, pp. 1-17. 2020. Crossref, https://doi.org/10.1038/s41598-020-71080-0
[10] Lingfei Yan et al., “PSP Net-Based Automatic Segmentation Network Model for Prostate Magnetic Resonance Imaging,” Computer Methods and Programs in Biomedicine, vol. 207, no. 106211, pp. 1-8, 2021.
Crossref, https://doi.org/10.1016/j.cmpb.2021.106211
[11] Vladimir Zaichick, and Sofia Zaichick, "Using Prostatic Fluid Levels of Zinc to Iron Concentration Ratio in Non-Invasive and Highly Accurate Screening for Prostate Cancer," SSRG International Journal of Medical Science, vol. 6, no. 11, pp. 24-31, 2019. Crossref, https://doi.org/10.14445/23939117/IJMS-V6I11P108
[12] Taresh Sarvesh Sharan et al., “Encoder Modified U-Net and Feature Pyramid Network for Multi-class Segmentation of Cardiac Magnetic Resonance Images,” IETE Technical Review, vol. 39, no. 5, pp. 1-13, 2021.
Crossref, https://doi.org/10.1080/02564602.2021.1955760
[13] Davood Karimi et al., “Prostate Segmentation in MRI using a Convolutional Neural Network Architecture and Training Strategy Based on Statistical Shape Models,” International Journal of Computer Assisted Radiology and Surgery, vol. 13, no. 8, pp. 1211–1219,018. Crossref, https://doi.org/10.1007/s11548-018-1785-8
[14] Md Sazzad Hossain, Andrew P. Paplinski, and John M. Betts, “Residual Semantic Segmentation of the Prostate from Magnetic Resonance Images,” International Conference on Neural Information Processing, pp. 510–521, 2018.
Crossref, https://link.springer.com/book/10.1007/978-3-030-04167-0
[15] V .P. Amadi, N.D Nwiabu, and V. I. E. Anireh, "Case-Based Reasoning System for the Diagnosis and Treatment of Breast, Cervical and Prostate Cancer," SSRG International Journal of Computer Science and Engineering , vol. 8, no. 8, pp. 13-20, 2021. Crossref, https://doi.org/10.14445/23488387/IJCSE-V8I8P103
[16] Pulung Hendro Prastyo, and Amin Siddiq Sumi, “Optic Cup Segmentation using U-Net Architecture on Retinal Fundus Image,” Journal of Information Technology and Computer Engineering, vol. 4, no. 2, pp. 105-109, 2020.
Crossref, https://doi.org/10.25077/jitce.4.02.105-109.2020
[17] Abhishek Chaurasia, and Eugenio Culurciello, “LinkNet: Exploiting Encoder Representations for Efficient Semantic Segmentation,” IEEE Visual Communications and Image Processing, pp. 1-4, 2017.
Crossref, https://doi.org/10.1109/VCIP.2017.8305148
[18] Komarala Narendra Babu et al., "An Updated Review on Nanoparticles Targeting Prostate Cancer," SSRG International Journal of Medical Science, vol. 9, no. 6, pp. 18-31, 2022. Crossref, https://doi.org/10.14445/23939117/IJMS-V9I6P103
[19] Long D. Nguyen et al., “Deep CNNs for Microscopic Image Classification by Exploiting Transfer Learning and Feature Concatenation,” IEEE International Symposium on Circuits and Systems, pp. 1-5, 2018.
Crossref, https://doi.org/10.1109/ISCAS.2018.8351550
[20] NCI-ISBI 2013. [Online]. Available: https://wiki.cancerimaging archive.net/display/Public/NCI-ISBI+2013+Challeng e+Automated+Segmentation+of+Prostate+Structures.
[21] Hamid Moradi, and Amir Hossein Foruzan, “Integration of Dynamic Multi-Atlas and Deep Learning Techniques to Improve Segmentation of the Prostate in MR Images,” International Journal of Image and Graphics, vol. 22, no. 4, pp. 1-17, 2022. Crossref, https://doi.org/10.1142/S0219467822500310
[22] C. Narmatha, and M Surendra Prasad “A Review on Prostate Cancer Detection using Deep Learning Techniques,” Journal of Computational Science and Intelligent Technologies,; vol. 1, no. 2, pp. 26–33, 2020.
Crossref, https://doi.org/10.53409/mnaa.jcsit20201204
[23] M. N. Rajesh, and B. S. Chandrasekar, “Imaging Modalities used in Prostate Cancer Detection,” Journal of Computational Science and Intelligent Technologies, vol. 2, no. 1, pp. 27–33, 2021. Crossref, https://doi.org/10.53409/mnaa/jcsit/2105
[24] M. N. Rajesh, B. S. Chandrasekar, and S. Shivakumar Swamy, "Feature Extraction and Analysis of Prostate Cancer MR Images," 2nd International Conference on Technological Advancements in Computational Sciences (ICTACS), pp. 563-572. 2022. Crossref, https://doi.org/10.1109/ICTACS56270.2022.9988410
[25] M. N. Rajesh, B. S. Chandrasekar, and S. Shivakumar Swamy, "Prostate Cancer Detection using Radiomics-based Feature Analysis with ML Algorithms and MR Images," SSRG International Journal of Engineering Trends and Technology, vol. 70, no. 12, pp. 42-58, 2022. Crossref, https://doi.org/10.14445/22315381/IJETT-V70I12P206
[26] M. N. Rajesh, and B. S. Chandrasekar, "Prostate Gland Segmentation using Semantic Segmentation Models U-Net and LinkNet," SSRG International Journal of Engineering Trends and Technology, vol. 70, no. 12, pp. 252-271, 2022.
Crossref, https://doi.org/10.14445/22315381/IJETT-V70I12P224
[27] Albert Comelli et al., “Deep Learning-Based Methods for Prostate Segmentation in Magnetic Resonance Imaging,” Applied Sciences, vol. 11, no. 2, pp. 782, 2021. Crossref, https://doi.org/10.3390/app11020782
[28] Yulian Zhu et al., “MRI-Based Prostate Cancer Detection with High-Level Representation and Hierarchical Classification,” Medical Physics. vol. 44, no. 3, pp. 1028–1039. 2017. Crossref, https://doi.org/10.1002/mp.12116
[29] Ekam Singh Chahal et al., “Unet based Xception Model for Prostate Cancer Segmentation from MRI Images,” Multimedia Tools and Applications, vol. 81, pp. 37333–37349, 2022. Crossref, https://doi.org/10.1007/s11042-021-11334-9