Modeling River Discharge using Deep Learning in the Ouémé catchment at Savè outlet (Benin, West Africa)
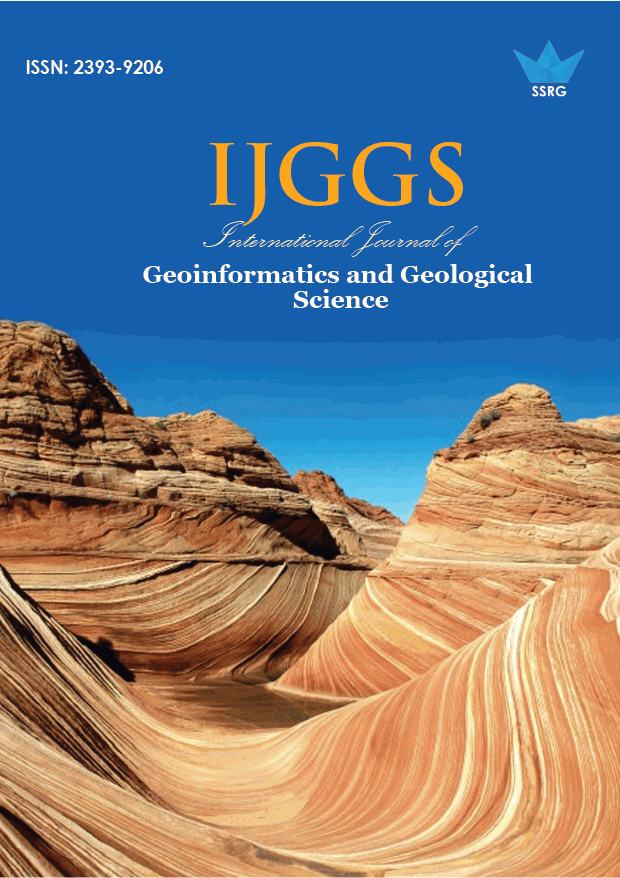
International Journal of Geoinformatics and Geological Science |
© 2023 by SSRG - IJGGS Journal |
Volume 10 Issue 1 |
Year of Publication : 2023 |
Authors : Zohou Pierre Jérôme, Biao Iboukoun Eliézer, Aoga John, Houessou Oscar, Alamou Adéchina Eric, Eugène C.Ezin |
How to Cite?
Zohou Pierre Jérôme, Biao Iboukoun Eliézer, Aoga John, Houessou Oscar, Alamou Adéchina Eric, Eugène C.Ezin, "Modeling River Discharge using Deep Learning in the Ouémé catchment at Savè outlet (Benin, West Africa)," SSRG International Journal of Geoinformatics and Geological Science, vol. 10, no. 1, pp. 29-35, 2023. Crossref, https://doi.org/10.14445/23939206/IJGGS-V10I1P103
Abstract:
This paper presents a modeling approach based on Artificial Neural Networks (ANNs) in the Ouémé river catchment at Savè. To do this, we used precipitation data as input over the period 1965 -2010 to simulate river discharge in the study area by using two ANNs models such as the Long Short Term Memory (LSTM) and Recurrent Gate Networks (GRU) models. Indeed, the description of the stochastic nature of the data is better presented today by ANNs models than the statistical models. We compared the performance of these two models based on different evaluation criteria. The predictions made using these models show a strong similarity between the observed and simulated flows. The deep learning models gave good results. Indeed, in calibration and validation, the Nash Sutcliffe Efficiency (NSE) and the coefficient of determination (R²) are very close to one (calibration: R²= 0.995, NSE= 0.991, and RMSE= 0.18; validation: R² = 0.975, NSE= 0.971, and RMSE= 0.41). This good performance of LSTM and GRU confirms the importance of models based on Artificial Intelligence in modeling hydrological phenomena for better decision-making.
Keywords:
Artificial Neural Networks, Modeling, Ouémé catchment at Savè, Long Short Term Memory, Gated Recurrent Unit.
References:
[1] Agon, Orel, “Rainfall Variability and Impacts on Surface Water Resources in the Zou Basin at Atcherigbe,” International Journal of Progressive Sciences and Technologies, 2020.
[2] A. Alamou, “Application of the Least Action Principle to Rainfall Flow Modeling,” Unique Doctoral Thesis, University of Abomey-Calavi, p. 231, 2016.
[3] Balduíno César Mateus et al., “Comparing LSTM and GRU Models to Predict the Condition of a Pulp Paper Press,” Energies, 2021.
[CrossRef] [Google Scholar] [Publisher Link]
[4] Eliézer Iboukoun Biao et al., "Improving Rainfall-Runoff Modelling through Uncertainties’ Control under Increasing Climate Variability in the Oueme," Doctoral Thesis, University of Abomey-Calavi, 2015.
[5] Junyoung Chung et al., “Empirical Evaluation of Gated Recurrent Neural Networks on Sequence Modeling,” arXiv, 2014.
[CrossRef] [Google Scholar] [Publisher Link]
[6] Sepp Hochreiter, and Jürgen Schmidhuber, “Long Short-Term Memory,” Neural Computation, pp. 1735-1780, 1997.
[CrossRef] [Google Scholar] [Publisher Link]
[7] M.A. Manjunatha Swamy et al., "Evaluating the Impacts of Climate Change on Thunga River Basin Karnataka," SSRG International Journal of Agriculture & Environmental Science, vol. 8, no. 5, pp. 1-8, 2021.
[CrossRef] [Publisher Link]
[8] Y. B. Koffi, Study of the Calibration, Validation and Performance of Formal Neural Networks Based on Hydro-Climatic Data from the Bandama Blanc Watershed in Ivory Coast, 2014.
[9] N. Laborde, Towards a New Approach for Determining the Siltation Rate of Dams, 1998.
[10] S. Lallahem, “Structure and Hydrodynamic Modeling of Two Groundwaters: Application to the Chalky Aquifer of the Northern Border of the Paris Basin,” Lille: Geological Society of the North, 2023.
[11] M.-A. Boucher, J. Q “Data Assimilation for Streamflow Forecasting,” Water Ressources Research, 2020.
[12] Daniel N. Moriasi, “Hydrologic and Water Quality Models: Key Calibration and Validation,” American Society of Agricultural and Biological Engineers, 2015.
[CrossRef] [Google Scholar] [Publisher Link]
[13] K. P. Sudheer, A. K. Gosain, and K. S. Ramasastri, “A Data-Driven Algorithm for Constructing Artificial Neural Network Rainfall-Runoff Models,” Hydrological Processes, Hydrological Processes, vol. 16, no. 6, pp. 1325-1330, 2020.
[CrossRef] [Google Scholar] [Publisher Link]
[14] Mohamed Samir Toukourou, Application of Machine Learning to Flash Flood Forecasting, ParisTech Online Theses, 2015.
[15] A.Vannieuwenhuyze, Machine Learning and Deep Learning by doing, 2019.
[16] Xuan-Hien Le et al., “Comparison of Deep Learning Methods for Hydrological Forecasting in a Large-Scale Basin,” Remote Sensing of Environment, vol. 251, p. 111631, 2020.
[17] S. Sadeghian et al., “Modeling and Forecasting River Flow Using Hybridized Deep Learning Methods,” Journal of Hydrology, vol. 569, pp. 594-606, 2019.
[18] Télesphore Cossi Nounangnonhou et al., “Modelling and Prediction of Ouémé (Benin) River Flows by 2040 Based on Gr2m Approach,” Accra Technical University Library, 2018.
[Google Scholar] [Publisher Link]
[19] V. Banupriya, and S. Anusuya, "Improving Classification of Retinal Fundus Image Using Flow Dynamics Optimized Deep Learning Methods," SSRG International Journal of Electrical and Electronics Engineering, vol. 9, no. 12, pp. 39-48, 2022.
[CrossRef] [Publisher Link]
[20] Poornima, S. Pushpalatha, M. “Prediction of Rainfall Using Intensified LSTM Based Recurrent Neural Network with Weighted Linear Units,” Atmosphere, vol. 10, p. 668, 2019.
[CrossRef] [Google Scholar] [Publisher Link]
[21] Zhongrun Xiang, Jun Yan, and Ibrahim Demir, “A Rainfall-Runoff Model with LSTM-Based Sequence-To-Sequence Learning,” Water Resources Research, vol. 56, p. e2019WR025326, 2020.
[CrossRef] [Google Scholar] [Publisher Link]
[22] Dechao Sun et al., "Prediction of Short-Time Rainfall Based on Deep Learning," Mathematical Problems in Engineering, vol. 2021, 2021.
[CrossRef] [Google Scholar] [Publisher Link]
[23] S. Riad, J. Mania, L. Bouchaou, Y. Najjar, “Rainfall-Runoff Model Usingan Artificial Neural Network Approach,” Mathematical and Computer Modelling, vol. 40, pp. 839-846, 2004.
[CrossRef] [Google Scholar] [Publisher Link]
[24] John Abbot, Jennifer Marohasy, “Input Selection and Optimisation for Monthly Rainfall Forecasting in Queensland, Australia, Using Artificial Neural Networks,” Atmospheric Research, pp. 166-178, 2014.
[CrossRef] [Google Scholar] [Publisher Link]
[25] Christian W. Dawson, and Robert Wilby, “An Artificial Neural Network Approach to Rainfall-Runoff Modelling,” Hydrological Sciences Journal, vol. 43, no. 1, 1998.
[CrossRef] [Google Scholar] [Publisher Link]
[26] Diederik Kingma, and Jimmy Ba, “A Method for Stochastic Optimzation,” arXiv, 2014.
[CrossRef] [Google Scholar] [Publisher Link]
[27] Longzhang Fang, and Dongguo Shao, “Application of Long Short-Term Memory (LSTM) on the Prediction of Rainfall-Runoff in Karst Area,” Frontiers in Physics, vol. 9, p. 790687, 2022.
[CrossRef] [Google Scholar] [Publisher Link]