Vibration Analysis of Frequency Domain Data using MATLAB for Application of Rotating Part Machines in Industry
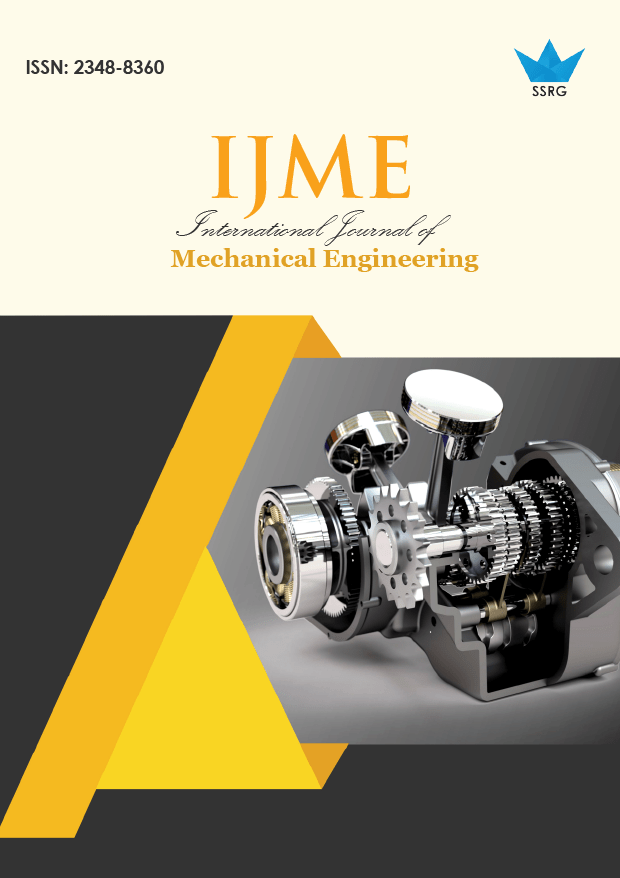
International Journal of Mechanical Engineering |
© 2023 by SSRG - IJME Journal |
Volume 10 Issue 1 |
Year of Publication : 2023 |
Authors : B. K. Pavan Kumar, Yadavalli Basavaraj |
How to Cite?
B. K. Pavan Kumar, Yadavalli Basavaraj, "Vibration Analysis of Frequency Domain Data using MATLAB for Application of Rotating Part Machines in Industry," SSRG International Journal of Mechanical Engineering, vol. 10, no. 1, pp. 1-5, 2023. Crossref, https://doi.org/10.14445/23488360/IJME-V10I1P101
Abstract:
Increasing the number of Condition-Based Profitability and safety are given emphasis in monitoring activity. Maintenance is the prevention of anticipated problems by monitoring the machine in time for it to run, which involves process control, keeping the machine operating, logistics, and improvement. This paper focuses on a unique feature of predictive maintenance utilizing the MATLAB tool's State space model, and accuracy is more than 85%. The frequency data is primarily collected from rotating machines using vibrometers, and the obtained spectrums are analyzed using MATLAB for validation, which clearly defines the severity level of vibration in a component and estimates the machine's life by creating a state space model and analyzing it using the asset tool.
Keywords:
Maintenance, MATLAB, Frequency domain data, Condition monitoring, Vibration analysis.
References:
[1] Mazzoleni, Y. Maccarana, and F. Previdi, "A Comparison of Data-Driven Fault Detection Methods with Application to Aerospace Electro- Mechanical Actuators,” IFAC-Papers, vol. 50, no. 1, pp. 12797-12802, 2017.
[CrossRef] [Google Scholar] [Publisher Link]
[2] Van Tung, Tran, and Bo-Suk Yang, “Machine Fault Diagnosis and Prognosis: the State-of-the-Art,” International Journal of Fluid Machinery and Systems, vol. 2, no. 1, pp. 61-71, 2009.
[CrossRef] [Google Scholar] [Publisher Link]
[3] Aida Rezaei, “Fault Detection and Diagnosis on the Rolling Element Bearing,” Masters Abstracts International, vol. 46, no. 03, 2007.
[Google Scholar] [Publisher Link]
[4] Bin Lu, and Santosh Sharma, “A Literature Review of IGBT Fault Diagnostic and Protection Methods for Power Inverters,” 2008 IEEE Industry Applications Society Annual Meeting, pp. 1-8, 2008.
[CrossRef] [Google Scholar] [Publisher Link]
[5] Attoui, I et al., “Fault Diagnosis of Rotating Machinery Based on Vibration Signal Analysis,” In 3rd International Conference on Advances in Mechanical Engineering, 2017.
[Google Scholar]
[6] Zhihe Duan et al., “Development and Trend of Condition Monitoring and Fault Diagnosis of Multi-Sensors Information Fusion for Rolling Bearings: A Review,” The International Journal of Advanced Manufacturing Technology, vol. 96, no.1-4, pp. 803-819, 2018.
[CrossRef] [Google Scholar] [Publisher Link]
[7] Mellit, Adel, Giuseppe Marco Tina, and Soteris A. Kalogirou, “Fault Detection and Diagnosis Methods for Photovoltaic Systems Using Neural Network: A Review,” Renewable and Sustainable Energy Reviews, vol. 91, pp. 1-17, 2018.
[CrossRef] [Google Scholar] [Publisher Link]
[8] Srinivas Katipamula, and Michael R. Brambley, “Methods for Fault Detection, Diagnostics, and Prognostics for Building Systems-A Review, Part I,” Hvac&R Research, vol. 11, no. 1, pp. 3-25, 2005.
[CrossRef] [Google Scholar] [Publisher Link]
[9] Mariela Cerrada et al., “A Review on Data-Driven Fault Severity Assessment in Rolling Bearings,” Mechanical Systems and Signal Processing, vol. 99, pp. 169-196, 2018.
[CrossRef] [Google Scholar] [Publisher Link]
[10] Olivier Janssens et al., “Convolutional Neural Network-Based Fault Detection for Rotating Machinery,” Journal of Sound and Vibration, vol. 377, pp. 331-345, 2016.
[CrossRef] [Google Scholar] [Publisher Link]
[11] S.P. Pirogov et al., "Simulation of Forced Oscillations of Pressure Monitoring Devices," International Journal of Engineering Trends and Technology, vol. 70, no. 3, pp. 37-47, 2022.
[CrossRef] [Google Scholar] [Publisher Link]
[12] Linlin Li et al., “Diagnostic Observer Design for T–S Fuzzy Systems: Application to Real-Time-Weighted Fault-Detection Approach,” IEEE Transactions on Fuzzy Systems, vol. 26, no. 2, pp. 805-816, 2018.
[CrossRef] [Google Scholar] [Publisher Link]
[13] A Aherwar, “An Investigation on Gearbox Fault Detection Using Vibration Analysis Techniques: A Review,” Australian Journal of Mechanical Engineering, vol. 10, no. 2, pp. 169-184, 2011.
[CrossRef] [Google Scholar] [Publisher Link]
[14] S.P. Berge, B.F. Lund, and R. Ugarelli, “Condition Monitoring for Early Failure Detection. Frognerparken Pumping Station as Case Study,” Procedia Engineering, vol. 70, pp. 162–171, 2014.
[CrossRef] [Google Scholar] [Publisher Link]
[15] Y H Kim, A C C Tan, and V Kosse, “Condition Monitoring of Low Speed Bearings - A Review,” Australian Journal of Mechanical Engineering, vol. 6, no. 1, pp. 61-68, 2015.
[CrossRef] [Google Scholar] [Publisher Link]
[16] I. M. Jamadar et al., “Model-Based Condition Monitoring for the Detection of Failure of a Ball Bearing in a Centrifugal Pump,” Journal of Failure Analysis and Prevention, vol. 19, pp. 1556-68, 2019.
[CrossRef] [Google Scholar] [Publisher Link]
[17] M. Lokesha et al., “Fault Detection and Diagnosis Ingears Using Wavelet Enveloped Power Spectrum and ANN,” International Journal of Research In Engineering and Technology, vol. 2, no. 9, pp. 146-158, 2013.
[CrossRef] [Google Scholar] [Publisher Link]
[18] Chunzhi Wu et al., “Intelligent Fault Diagnosis of Rotating Machinery Based on One-Dimensional Convolutional Neural Network,” Computers in Industry, vol. 108, pp. 53-61, 2019.
[CrossRef] [Google Scholar] [Publisher Link]
[19] Pankaj Gupta, and M. K Pradhan, “Fault Detection Analysis In Rolling Element Bearing: A Review,” Materials Today: Proceedings, vol. 4, no. 2, pp. 2085–2094, 2017.
[CrossRef] [Google Scholar] [Publisher Link]
[20] V. Devaraj, and M. Kumaresan, "An Elite LOA-TFWO Approach for Load-Frequency Control of Islanded Micro-Grids Incorporating Renewable Sources," International Journal of Engineering Trends and Technology, vol. 70, no. 10, pp. 166-187, 2022.
[CrossRef] [Publisher Link]
[21] James Wakiru et al., “A Data Mining Approach for Lubricant-Based Fault Diagnosis,” Journal of Quality in Maintenance Engineering, vol. 27, no. 2, pp. 1355-1365, 2020.
[CrossRef] [Google Scholar] [Publisher Link]
[22] Pengfei Liang et al., “Compound Fault Diagnosis of Gearboxes via Multi-Label Convolutional Neural Network and Wavelet Transform,” Computers In Industry, vol. 113, no. c, 2019.
[CrossRef] [Google Scholar] [Publisher Link]
[23] Soumava Boral, Sanjay Kumar Chaturvedi, and V.N.A. Naikan, “A Case Based Reasoning System for Fault Detection and Isolation, A Case Study on Complex Gearboxes,” Journal of Quality In Maintenance Engineering, vol. 25, no. 2, pp. 213-235, 2019.
[CrossRef] [Google Scholar] [Publisher Link]
[24] Weiwei Qian et al., “A Novel Transfer Learning Method for Robust Fault Diagnosis of Rotating Machines Under Variable Working Conditions,” Journal of Measurement, vol. 138, pp. 514-525, 2019.
[CrossRef] [Google Scholar] [Publisher Link]
[25] Fafa Chen et al., “Performance Degradation Prediction of Mechanical Equipment Based on Optimized Multi-Kernel Relevant Vector
Machine and Fuzzy Information Granulation,” Measurement, vol. 151, 2020.
[CrossRef] [Google Scholar] [Publisher Link]
[26] Ana Gonzalez Muniz, Ignacio Diaz, and Abel Cuadrado, “DCNN for Condition Monitoring and Fault Detection in Rotating Machines and Its Contribution to the Understanding of Machine Nature,” Heliyon Journal, vol. 6, no. 2, pp. 3395-3405, 2020.
[CrossRef] [Google Scholar] [Publisher Link]
[27] Fanbiao Li et al., “Fault Detection Filtering for Nonhomogeneous Markovian Jump Systems via a Fuzzy Approach,” IEEE Transactions on Fuzzy Systems, vol. 26, no. 1, pp. 131-141, 2018.
[CrossRef] [Google Scholar] [Publisher Link]