A Hybrid Compressive Sensing Network for ROI-based Medical Image Recovery
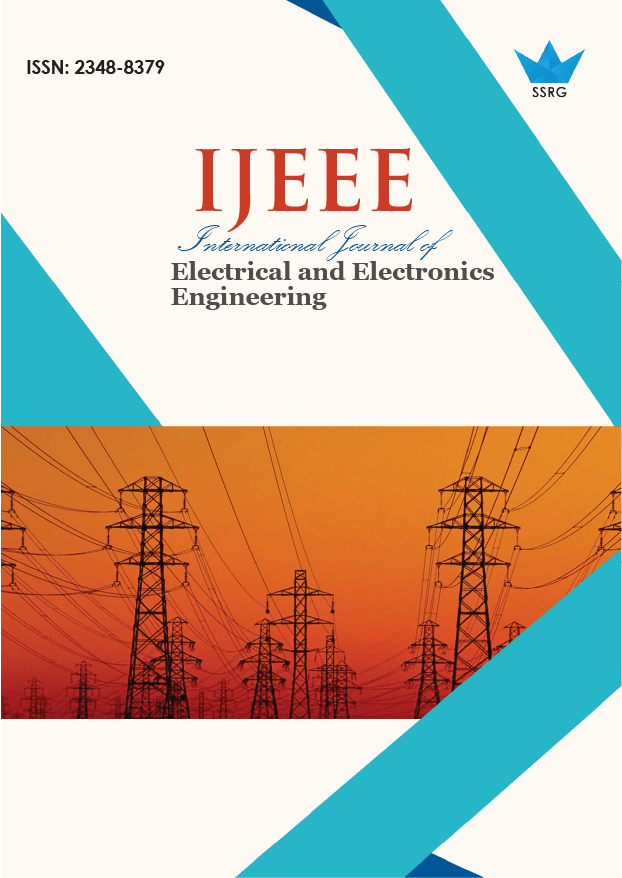
International Journal of Electrical and Electronics Engineering |
© 2023 by SSRG - IJEEE Journal |
Volume 10 Issue 3 |
Year of Publication : 2023 |
Authors : A N Shilpa, C S Veena |
How to Cite?
A N Shilpa, C S Veena, "A Hybrid Compressive Sensing Network for ROI-based Medical Image Recovery," SSRG International Journal of Electrical and Electronics Engineering, vol. 10, no. 3, pp. 8-19, 2023. Crossref, https://doi.org/10.14445/23488379/IJEEE-V10I3P102
Abstract:
The analog signals are changed to digital signals for an instance of time designed as a powerful imaging system. The imaging system produced in the digital form as it evolves the analog imaging devices has the ability to perform digital technology. Therefore, the image expansion is guided by the diagnostic system and surgical systems. The present research work used a hybrid compressive sensing algorithm consisting of an optimized neural network and lossy-lossless compression using Adaptively learned sparsifying based on L1 minimization. The Region of Interest (ROI) is compressed using Integer-based Lifting Wavelet Transform with lossless compression, and the non-ROI using an Optimized neural network. The lossy models are irreversible and achieve a higher compression ratio; therefore, the medical image processing has the visual quality in reconstruction showing compression ratio at the highest. The proposed method overcomes the dimensional reduction problem for optimizing with sparsity to non-ROI regions. Therefore, the medical image is transmitted over the network with limited bandwidth. The proposed work outcomes showed that the developed model attained a PSNR of 35.62 dB and SSIM of 0.984 better when compared to the existing ROI-CS Net model, which obtained a PSNR of 29.55 dB and SSIM of 0.89.
Keywords:
Hybrid compressive sensing, Lifting wavelet transform, Optimized neural network, Region of interest.
References:
[1] Mingli Zhang et al., ‘Robust Brain MR Image Compressive Sensing Via Re-weighted Total Variation and Sparse Regression,” Magnetic Resonance Imaging, vol. 85, pp. 271–286, 2022.
CrossRef | Google Scholar | Publisher Link
[2] Morteza Mardani et al., “Deep Generative Adversarial Neural Networks for Compressive Sensing MRI,” IEEE Transactions on Medical Imaging, vol. 38, no. 1, pp. 167–179, 2019.
CrossRef | Google Scholar | Publisher Link
[3] Minghui Zhang et al., “High-dimensional Embedding Network Derived Prior for Compressive Sensing MRI Reconstruction,” Medical Image Analysis, vol. 64, p. 101717, 2020.
CrossRef | Google Scholar | Publisher Link
[4] Yan Yang et al., “ADMM-CSNet: A Deep Learning Approach for Image Compressive Sensing,” IEEE Transactions on Pattern Analysis and Machine Intelligence, vol. 42, no. 3, pp. 521–538, 2020.
CrossRef | Google Scholar | Publisher Link
[5] Agouzal Mehdi, Merzouqi Maria, and Moha Arouch, “An Original Method of Extraction Bands Based On Spectral And Spatial Characteristic To Reduce The Hyperspectral Image,” International Journal of Engineering Trends and Technology, vol. 70, no. 1, pp. 185-194, 2022.
CrossRef | Publisher Link
[6] Shrividya Guruprasad, S. H. Bharathi, and D. Anto Ramesh Delvi, “Effective Compressed Sensing MRI Reconstruction Via Hybrid GSGWO Algorithm,” Journal of Visual Communication and Image Representation, vol. 80, p. 103274, 2021.
CrossRef | Google Scholar | Publisher Link
[7] Xiaohong Fan, Yin Yang, and Jianping Zhang, “Deep Geometric Distillation Network for Compressive Sensing MRI,” 2021 IEEE EMBS International Conference on Biomedical and Health Informatics (BHI), pp. 1–4, 2021.
CrossRef | Google Scholar | Publisher Link
[8] Guillermo Marzik, Shin-ichi Sato, and Mariano Ezequiel Girola, “Compressive Sensing for Perceptually Correct Reconstruction of Music and Speech Signals,” Applied Acoustics, vol. 183, p. 108328, 2021.
CrossRef | Google Scholar | Publisher Link
[9] Hong Lu et al., ‘Deep Convolutional Neural Network for Compressive Sensing of Magnetic Resonance Images,” International Journal of Pattern Recognition and Artificial Intelligence, vol. 35, no. 15, p. 2152019, 2021.
CrossRef | Google Scholar | Publisher Link
[10] Vidyasagar Talwar, and B.S.Sohi, “Dimensionality Reduction of Hyperspectral Image Using Different Methods,” International Journal of Engineering Trends and Technology, vol. 69, no. 2, pp. 139-143, 2021.
CrossRef | Publisher Link
[11] Indrarini Dyah Irawati et al., “Lifting Wavelet Transform in Compressive Sensing for MRI Reconstruction,” Journal of Southwest Jiaotong University, vol. 55, no. 5, 2020.
CrossRef | Google Scholar | Publisher Link
[12] Ping Xu et al., “A Region-based Block Compressive Sensing Algorithm for Plant Hyperspectral Images,” Computers and Electronics in Agriculture, vol. 162, pp. 699–708, 2019.
CrossRef | Google Scholar | Publisher Link
[13] Yujiao Zheng et al., “Compressive Sensing Based Probabilistic Sensor Management for Target Tracking in Wireless Sensor Networks,” IEEE Transactions on Signal Processing, vol. 63, no. 22, pp. 6049–6060, 2015.
CrossRef | Google Scholar | Publisher Link
[14] Soyoung Park et al., “Image Reconstruction in Region-of-interest (or interior) Digital Tomosynthesis (DTS) Based on Compressed-Sensing (CS),” Computer Methods and Programs in Biomedicine, vol. 151, pp. 151–158, 2017.
CrossRef | Google Scholar | Publisher Link
[15] Nobuyuki Kawai et al., “Gadoxetic Acid-enhanced Dynamic Magnetic Resonance Imaging using Optimized Integrated Combination of Compressed Sensing and Parallel Imaging Technique,” Magnetic Resonance Imaging, vol. 57, pp. 111–117, 2019.
CrossRef | Google Scholar | Publisher Link
[16] Liyan Sun et al., “Region-of-interest Undersampled MRI Reconstruction: A Deep Convolutional Neural Network Approach,” Magnetic Resonance Imaging, vol. 63, pp. 185–192, 2019.
CrossRef | Google Scholar | Publisher Link
[17] Balamurali Murugesan et al., “A Deep Cascade of Ensemble of Dual Domain Networks with Gradient-based T1 Assistance and Perceptual Refinement for fast MRI Reconstruction,” Computerized Medical Imaging and Graphics, vol. 91, p.101942, 2021.
CrossRef | Google Scholar | Publisher Link
[18] Ines Njeh et al., “Deep Convolutional Encoder-Decoder Algorithm for MRI Brain Reconstruction,” Medical & Biological Engineering & Computing, vol. 59, pp.85-106, 2021.
CrossRef | Google Scholar | Publisher Link
[19] Wanyu Bian et al., “An Optimization-Based Meta-Learning Model for MRI Reconstruction with Diverse Dataset,” Journal of Imaging, vol. 7, no. 11, 2021.
CrossRef | Google Scholar | Publisher Link
[20] G. Vijayalakshmi, Sudipto Biswas, and Sankeerth Reddy, “Semantic Understanding of Abstract Images,” SSRG International Journal of Computer Science and Engineering, vol. 4, no. 4, pp. 30-34, 2017.
CrossRef | Publisher Link
[21] Adrienne M. Mendrik et al., “MRBrainS Challenge: Online Evaluation Framework for Brain Image Segmentation in 3T MRI Scans,” Computational Intelligence and Neuroscience, 2015.
CrossRef | Google Scholar | Publisher Link
[22] P.Kanmani, P.Marikkannu, and M.Brindha, “A Medical Image Classification using ID3 Classifier,” SSRG International Journal of Computer Science and Engineering, vol. 3, no. 10, pp. 8-11, 2016.
CrossRef | Google Scholar | Publisher Link
[23] Mohamed Ragab, Osama A. Omer, and Mohamed Abdel-Nasser, “Compressive Sensing MRI Reconstruction using Empirical Wavelet Transform and Grey Wolf Optimizer,” Neural Computing and Applications, vol. 32, pp. 2705–2724, 2020.
CrossRef | Google Scholar | Publisher Link
[24] Ejaz Ul Haq et al., “Block-based Compressed Sensing of MR Images using Multi-rate Deep Learning Approach,” Complex & Intelligent Systems, vol. 7, pp. 2437–2451, 2021.
CrossRef | Google Scholar | Publisher Link